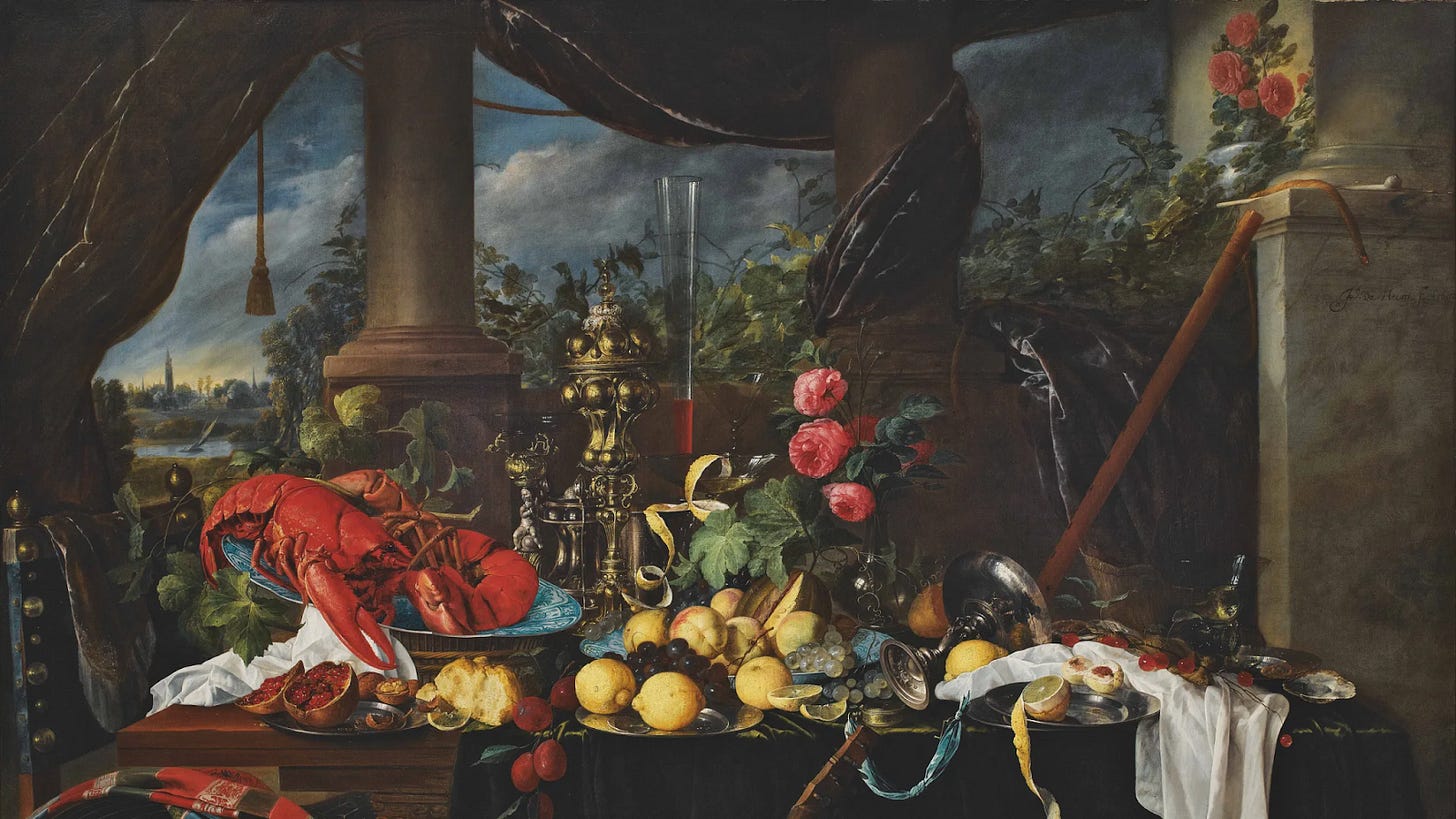
Before we get going, a quick word about affiliations. I left Google DeepMind in early December to pursue independent writing and research. See this post for the fineprint, but the long and short of it is that I’m working on a book about the history of AI with support from Tyler Cowen and the folks from Emergent Ventures. I’ll still be writing here, but I expect to produce more long-form stuff and fewer roundups. Whatever the case, thanks for reading — and please do keep getting in touch with me at hp464@cam.ac.uk.
In December 2023, I wrote about the year that was for AI policy. Mostly, I slalomed through some things I thought were interesting about 2023 and made a few loose predictions about what to expect in 2024. This year, I’m going to interrogate some of the things I said in the 2023 post, pick out claims or rough predictions I made in the weekly roundup, and share some thoughts about how my views have changed in the last twelve months.
You can see a summary of what I think I got wrong, what I reckon I got mostly right, and what I changed my mind about as the year went on in the scorecard above. In this post, I’ll go through these categories in turn and explain the basis of my original comment and how I’m thinking about a particular issue now that some time has passed.
What did I get wrong?
We begin with things that I misjudged. There were loads of examples to choose from here, but generally speaking I opted for big picture issues related to AI and society. Another contender that I omitted was robotics, which—though made some decent progress—wasn’t quite as impressive as I anticipated.
Robust UN action on international governance
I like to talk (and write) about the international governance of AI. By that, I mean less legacy efforts (e.g. the G7 Hiroshima process) or bilateral movements (e.g. collaboration between UK and US AI safety institutes) and more the introduction of new bodies, organisations, and norms to govern the emergence of powerful AI. Everyone has their own favourite model, historical parallel, or acronym (delete as appropriate) to inform AI policy, which in 2024 consolidated around three major winners. These were of course the International Atomic Energy Agency (IAEA), European Organization for Nuclear Research (CERN), and the Intergovernmental Panel on Climate Change (IPCC).
As last year drew to a close, there was some uncertainty about what role the United Nations would play within the oncoming regulatory melee. The first hint of white smoke emerged when the UN’s High Level Advisory Board on Artificial Intelligence released an interim report in December. Rather than proposing a specific model for international governance, the authors instead opted to provide general principles that could guide the formation of new global governance institutions for AI, as well as a broad assessment of the functions that such bodies should perform.
This initial report introduced seven types of possible function, which ranged from those that are easier to implement (e.g. a horizon scanning function similar to the IPCC) to those that are more challenging (e.g. monitoring and enforcement mechanisms “inspired by [the] existing practices of the IAEA”). With this in mind, I suggested that:
“There’ll be much more to come on this one because the Advisory Body will submit a second report by 31 August 2024 that “may provide detailed recommendations on the functions, form, and timelines for a new international agency for the governance of artificial intelligence.” While ‘may’ is obviously doing some heavy lifting I suspect this will be one to mark on the calendars.”
As it turned out, this was absolutely not one to mark on the calendars.
The final report advocated for the IPCC type model whose primary purpose seems to be to produce an article in The Guardian every couple of years. It also looks to be trying to occupy more or less the same space as the International Scientific Report on the Safety of Advanced AI chaired by Yoshua Bengio. Whatever the case, we can look forward to more stakeholder engagement, more reports, and more commentary from the UN for years to come. (You can also read a more optimistic take from me here.)
Widespread deployment of AI agents
In 2023 I expected the year ahead to be all about AI agents. Well, it’s a year later and I am talking about agents — but not in the way that I expected. Last year, I said:
“The likes of [community-built AI agents] BabyAGI and AutoGPT proved that simple GPT-powered agents were possible using non-specialised architectures. There’s also the GPT store that shows us what personalised models look like at scale. Proof of concept broadly in the bag, I expect to see assistants get much better, with OpenAI already thinking about how these types of systems ought to be governed. I also imagine that foundation models for robotics will drive very significant capability increases, so keep an eye out for agents and robotics (or a combination thereof) in the 2024 edition of The Year in Examples.”
To recap: agents are AI systems that can act autonomously. Sometimes called assistants, all the major labs are building agents with the aim of creating a system that can do more or less the same job as your average remote worker. A lofty goal to be sure, but one that I thought would see more progress in 2024 than we actually got. That’s not to say there were no important milestones—Anthropic showed off functionality for Claude that allows it to use computers after all—but none of the major labs got around to deploying autonomous agents for people to use.
At the risk of being wrong again, I expect that will happen in 2025. All the ingredients are in place—multimodality, computer use, more powerful base models—and the pitch has been rolled by announcements like Google’s Project Astra. Concretely, I think that looks like polished agent products from at least two major labs with combined monthly users in the millions.
One interesting unknown with agents is the pricing model, which is a decent bellwether for how lucrative the labs expect them to be. If they go for a ‘horizontal’ App Store-style model that takes a small cut of revenue produced by an agent, they probably expect them to be widely used in lots of different domains by many types of users. If they prefer a ‘vertical’ approach based on a chunky monthly fee (like the coding agent Devin), we can infer that labs believe that technology is more likely to be used by a relatively small number of power users.
Significant economic impact from AI
Because agents didn’t show up as expected, that ultimately meant we saw limited economic impact from AI. Revenues at AI firms probably totalled around $10bn last year, which is something like 0.01% of global GDP. Yes, they are currently accelerating rapidly, but we’re still dealing with a small fraction of the economy. I wrote in December 2023 that:
“In general, I expect much more to come in 2024 as companies, countries, and people grapple with how these technologies reshape the economic environment.”
Has AI reshaped the economic environment, though? I don’t think so. There are few ways we could think about this, but they basically boil down to the microeconomic picture (how AI is influencing the role of individual economic actors) and the macroeconomic picture (the sum of these changes on the economy).
Either way, it all begins with usage. Most of the studies tracking business and individual use seem to show widespread use from workers in developed economies, with approximately 30% of researchers saying that they had used generative AI tools to help write manuscripts. Outside of science, a March 2024 poll from Pew Research found that 43% of American adults aged 18-29 have used ChatGPT (a figure that has increased 10 percentage points since last summer).
The rub, though, is that much of this impact can be characterised as ‘shadow use’ i.e. instances in which individuals use AI tools in their workplace in a way that isn't formally endorsed by their employer. In other words: people are using conversational models at work, but no-one wants to admit it. The moment we’ll see AI reflected in the GDP figures will be once firms begin to formally direct their workers to use AI (though I expect to see increases in productivity in certain industries before that). There’s a weak version of this dynamic that involves better ways of folding conversational models into existing workflows, and a strong version of the dynamic in which organisations and their employees deploy autonomous agents at scale.
At the risk of being trite, we could do worse than to remind ourselves of Amara’s law: We tend to overestimate the effect of a technology in the short run and underestimate the effect in the long run. I expect this will prove to be the case for AI, but I imagine that the ‘long run’ is more like five years than fifty.
What did I get right?
Now for a few things I called more or less correctly. For a couple of these I am reading between the lines a bit — but I have tried to call out those moments to make sure I am properly contextualising these examples. There were also things that I mentioned last year like increases in “multimodal capability” and improvement in “reaction times” (i.e. latency) that I removed from this section as they seemed both too woolly and too much like a sure thing to deserve inclusion.
Europe falls further behind the US
Last year I talked a bit about European competitiveness in the context of its landmark piece of legislation, the mighty AI Act. I said:
“The latest text proposes the regulation of foundation models, which it defines as those trained on large volumes of data and adaptable to various tasks. For these models, developers must draw up technical documentation, comply with EU copyright law, and provide detailed summaries of the content used for training. Questions about the extent to which provisions will hinder the international competitiveness of European companies remain, with French president Macron stating that rules risk hampering European tech firms compared to rivals in the US, UK and China.”
Aphorisms about ‘raising questions’ aside, the broader point here is that, lo and behold, regulation is not in fact a competitive advantage for Europe. The view I hear about this time and again, from Europeans and Americans alike, is that regulation is the primary force preventing the emergence of a European OpenAI.
For a long time I thought that regulation was just one factor amongst many (including shallow capital markets, fragmentation, and ill-defined ‘cultural factors’). Now I’m not so sure. The fact that several frontier AI releases have been delayed (sometimes indefinitely) in Europe does not bode well for the continent. If and when AI begins to turbocharge global GDP, Europe getting left behind due to the Digital Markets Act would be a cruel fate indeed.
Worse, as a Brit, I can’t take any pleasure in this state of affairs because we too are unable to access some of the same models as our American counterparts. The fact that this, at least, doesn’t seem to have materialised as a benefit from the UK’s exit of the European Union is like something from a Samuel Beckett play. That being said, there are some silver linings in the UK and Europe proper. Both are toying with major investments in compute capacity, both have a groundswell of talented AI researchers, and both are looking to tinker with regulations to ensure models can actually, uh, be used by people. The best I think we can hope for, though, is that the gap doesn’t get any bigger — but my money says things will get worse before they get better.
Misinformation remains overpriced
AI and misinformation is a hobby horse of mine. I like to write about it because certain sections of the commentariat can’t seem to shake the idea that an AI-powered misinformation apocalypse is still just around the corner — even when all evidence points to the contrary. In January this year, I wrote:
“AI hasn’t yet hopelessly degraded our epistemic security—despite the emergence of powerful and widely available tools that have the potential to do so…The reason epistemic security is a good case study is that it confronts us with the uncomfortable gap between what we think should be happening and what is actually happening. This distance is directly proportional to marginal risk. When the marginal risk of deploying a new technology is smaller than we anticipate, the perception gap between speculated impact and real impact inflates.”
First of all, I am primarily talking about the stickiness of misinformation as an issue that people tend to inflate — rather than saying it will continue to pose a minimal risk for the foreseeable future. As a result, this falls into the ‘less’ bucket in terms of the things that I ‘more or less got right’. Nonetheless, I did write a piece in October where I returned to the issue through the lens of elections. Before that, in June, I wrote about a study published in Nature that argues that misinformation is a deeply understood phenomenon. As the authors explain: “Here we identify three common misperceptions: that average exposure to problematic content is high, that algorithms are largely responsible for this exposure and that social media is a primary cause of broader social problems such as polarization.”
I’m not going to get into the details (you can read about the ins and outs in the pieces linked above), but the broader point is that the gap between what some people think about the purported and actual harms of misinformation doesn’t seem to have gotten any smaller. Lest we forget that in January the World Economic Forum said that in the next two years AI-powered misinformation and disinformation represented a greater risk than “economic downturn[s]”, “extreme weather events”, and even “interstate armed conflict”. On the plus side, the WEF will be releasing a follow-up edition of that report in a few weeks — so we can see if they still think AI-powered misinformation should be canonised as the fifth horseman of the apocalypse.
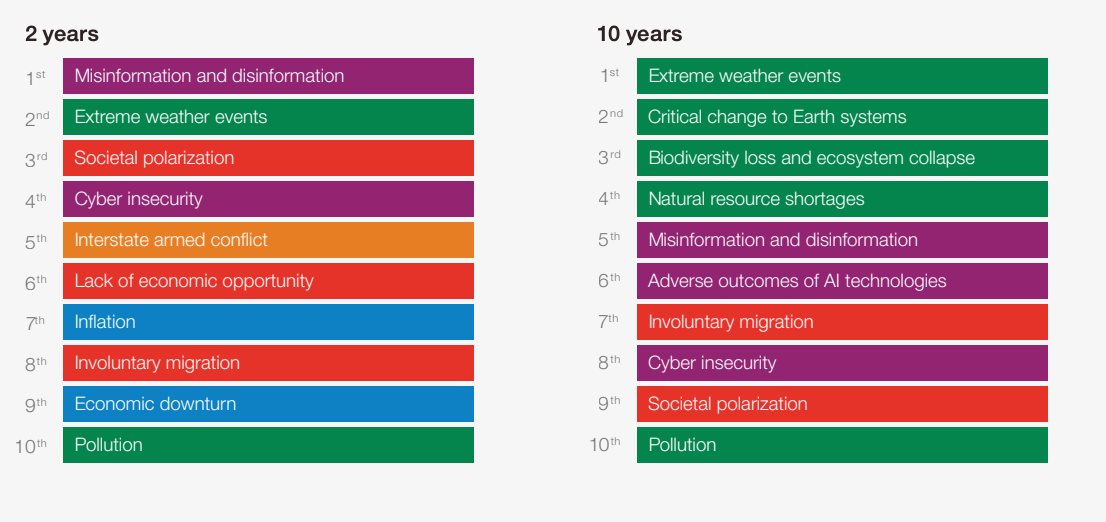
A model passes the ARC-AGI eval
Now for something a bit more concrete: predictions about whether a model will pass the ARC-AGI eval. As a reminder, entrepreneur Mike Knoop and deep learning researcher François Chollet launched a competition to solve an AI reasoning test in the middle of the year. Known as the Abstraction and Reasoning Corpus for Artificial General Intelligence (ARC-AGI), the test was originally created in 2019, but the top rated scores hadn’t improved much up to the launch of the competition.
Partly, that was because Chollet designed the test to include novel problems, which means—even ingesting massive chunks of the internet—large models are unlikely to have seen a critical mass of similar examples in their training data. The upshot is that, according to Chollet, because language models only apply existing templates to solve problems, they get stuck on tests that human children would be able to manage comfortably. Second, the test wasn’t particularly well known, which narrowed the pool of researchers trying to solve it relative to popular benchmarks. Then came the prize launch, which put something of a target on the benchmark. I said in June:
“The (quite literally) million dollar question is whether ARC-AGI will stand the test of time. If I had to guess, I would expect major progress on the challenge within the next year or so. This is because a) bigger models with some clever algorithmic improvements seem to be doing something other than simple pattern matching; and b) there’s already been some improvement on the benchmark since it was released.”
A few early entrants improved baseline scores to more respectable levels, but it wasn’t until OpenAI’s o3 model scored 87.5 percent on the ARC-AGI benchmark (albeit with a very costly process) that we could safely say the benchmark was passed. I’ll get into what’s going on here in my section below on ‘test time compute’, but the news caused even the most hardcore LLM sceptics to update their world model. Chollet himself, a well known critic of large language models’ ability to reason, said “all intuition about AI capabilities will need to get updated”, while Melanie Mitchell (also a long-time sceptic) called it “quite amazing”.
What did I change my mind about?
The third and final section deals with some of the things I changed my perspective on. There were a load of things that I could have chosen to include here, but I decided to stick with the ceiling of three per section given this post is getting a bit long. But before we get into the specifics, here’s a general reflection on the state of play: I feel more positive about the development of AI than I have at any point since I started working with AI companies.
Yes, that might prove to be completely unfounded, but I basically think our current instantiation of AI a) poses a much smaller existential risk than is typically assumed, b) is likely to have a net positive economic impact on workers, and c) will take a much longer time to reshape the world than we think (even assuming human-level AI within the next five years).
Test time compute
I briefly mentioned ‘test time compute’ above, but let’s begin by explaining what exactly it is and how it relates to the OpenAI o3 model that aced the ARC-AGI benchmark. In a nutshell, there are two types of compute associated with today’s large models: ‘training time’ compute and ‘inference’ or ‘test time’ compute. The former deals with the computational resources expended to train a model by updating the strength of internal connections called weights (totalling trillions in the biggest models). The latter, meanwhile, is about leveraging compute to do something once it has already been trained.
The significance of o3 is that OpenAI has found a way to increase the effectiveness of a model in tandem with the amount of compute expended at inference time. That might not sound like a massive deal, but it represents probably the single-biggest step jump in capability seen since the release of GPT-2 five years ago (with the caveat that no one has been able to actually try the model for themselves). No one outside of OpenAI is 100 per cent sure what is going on under the hood, but the general consensus is that the model is creating ‘chain of thought’ responses in which a task is broken down into steps that include descriptions of what the system is ‘thinking’ at each step. These steps are in turn used to guide the model further along the chain until it arrives at the correct answer.
The results are impressive. As well as the ARC-AGI benchmark I mentioned above, OpenAI also described massive increases on coding and maths challenges (as well as significant increases on other benchmarks). Test time compute becoming much, much more important wasn’t something I thought was likely to happen this year (more fool me for not recognising the power of thinking out loud). This dynamic was something that I changed my mind about in so far as it wasn’t really on my radar for 2024.
As well as the obvious importance of the paradigm, the thing I’ve changed my mind about is probably not so much the time it will take to build human-level AI — but rather the trajectory that labs are taking to get there. It seems pretty much certain to me that a) inference costs will come down (though margins may remain slim), b) inference will get faster, and c) with perhaps a couple of orders of magnitude larger models (like the ones being planned by every major lab) we should expect something more or less human-level AI shaped by 2030.
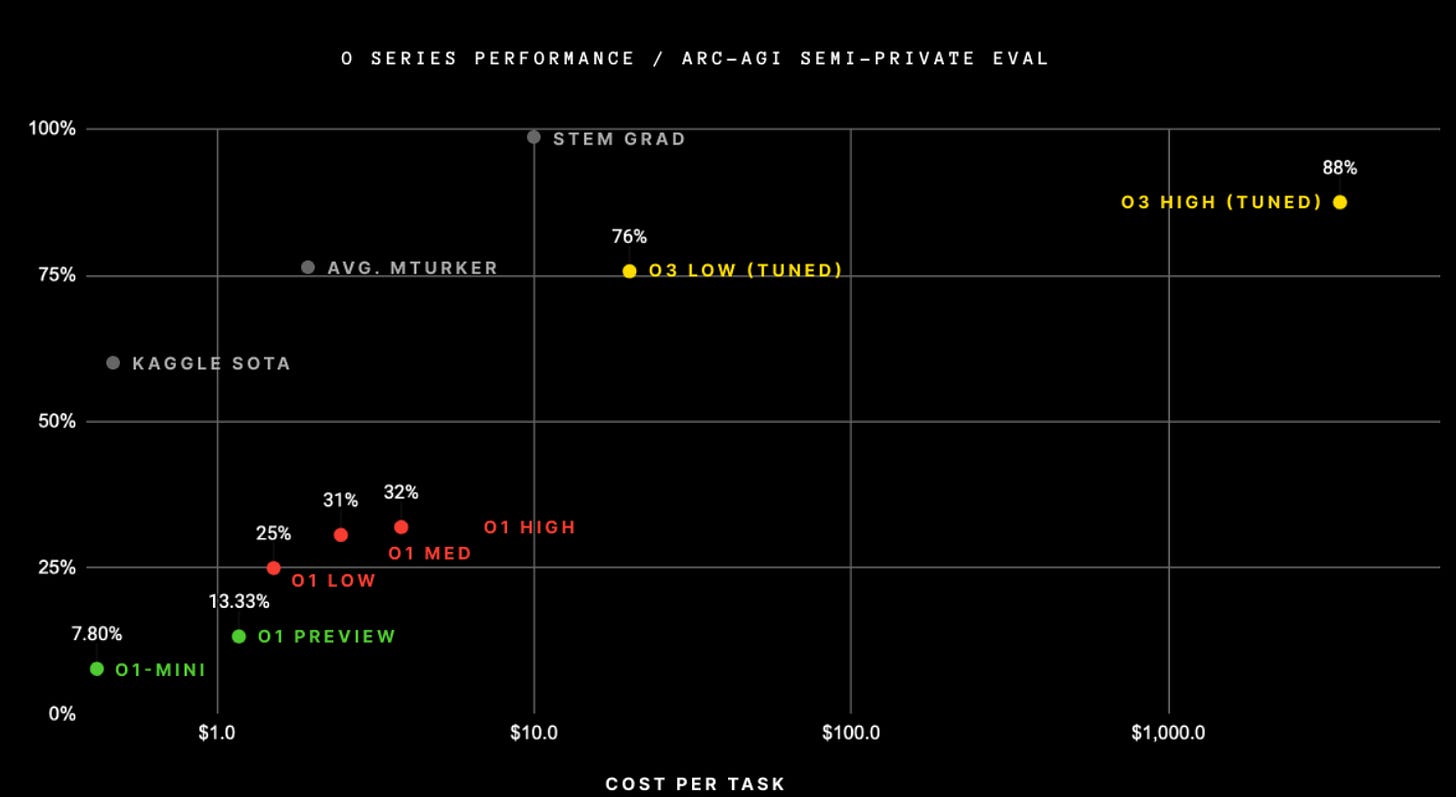
Relationship between access and safety
I’ll begin this one by saying I’m not completely sure how I feel, but certainly I’m now less convinced that widespread proliferation will always lead to the worst possible outcome. This perspective begins with the observation that the emergence of successful avenues for eking superior performance out of models, like the test time compute paradigm I describe above, means that human-level AI is likely to be much cheaper to create than had been assumed in the past.
This means that there will not be one country or one company in control of a human-level (or, quite possibly, superhuman-level) system. The upshot is that, over the medium term, pretty much everyone should expect to have access to the technology. If you had told me that two years ago, I would have said that we need to do all we can to consolidate the technology in a manner that allows a handful of actors to exist as trusted custodians. Now, while I accept it is quite possible that there is no way to manage the safe proliferation of advanced AI, I think that extreme proliferation may offer its own rewards.
It’s straightforwardly true that more agents means more bad actors can try to cause harm, and that more systems exist with the potential to be badly misaligned. But it’s also true that more agents means models can act as guardians and more exist to counter rogue elements. If you have one superintelligent model and it goes haywire you’re in trouble, if you have millions and one loses the plot it's (quite literally) not the end of the world.
Distributed resilience doesn't eliminate the need for safety measures, but it suggests that widespread access might contribute to, rather than detract from, overall system stability. Past a certain point, the most effective approach may be to embrace proliferation by developing frameworks that can harness the protective potential of multiple independent systems while mitigating the risks of malicious exploitation or misalignment. It’s a tough tightrope to walk, but not an impossible one.
Importance of AI practitioner histories
Finally, something a bit more niche. As I mentioned at the beginning of this post, I’m spending the vast majority of my time writing about the history of AI across my research at the University of Cambridge and as part of a book project. Since I began to spend more time reading and writing histories of AI—and, perhaps more importantly, interviewing active participants in the field’s history—I’ve started to alter my views about the role of ‘practitioner’ or researcher perspectives about the past.
To break this down a bit, the history of AI is generally considered to be a mess by historians of science and technology. This is no doubt true, not least because the two major branches of AI development (symbolic methods and the machine learning approaches that power today’s models) have been squashed together in stories about the field’s past. Another problem is the lack of ‘outsider’ accounts about the field, with the most influential histories (like Nils Nilsson’s The Quest for Artificial Intelligence) written by people who were active participants in the history of AI.
In general, the view from modern historians is that these practitioner accounts aren’t particularly valuable because a) we can’t trust the author to write about their own field with impartiality; and b) they focus far too much on technical elements at the expense of underlying forces. A year ago I would have said this statement is 90% right. Now, to stick with a vibes-based qualitative assessment, I’d assign about a 50% favorability rating to this idea. My problem lies with seeing these technical stories solely as proximate factors to be contextualised, rather than an essential part of a broader story about the influences brought to bear on AI development.
Yes, there are social and material forces that condition scientific practice. That much is obvious when you stop and think about the perspectives, values, and beliefs imbued within today’s large models by those who build them. But sociomaterial forces aren’t the only things that matter when dealing with the mess that is AI historiography. Someone who accounts for both sorts incredibly well is Margeret Boden. If you want to read an excellent history of AI, it doesn’t get much better than Mind As Machine. Fair warning: at 1,600 pages long, it is not for the faint of heart.
Wrapping up
That brings me to the end of my postmortem. I've tried to be honest about where I got things wrong, while also highlighting those moments where some of my rough ideas about the year panned out. Looking ahead to 2025 and beyond, agents feel closer than ever — and I suspect we'll finally start seeing AI's economic impact show up in ways that economists can actually measure.
Test-time compute approaches are only going to improve, and I would expect that the other labs will follow OpenAI’s lead by releasing models of their own. Whatever the case, I will be covering AI policy, history, and anything else that interests me in Learning From Examples in the year ahead. Thanks for reading and see you then.
Incredible work. You have convinced me about o3. The one additional voice of support I needed to hear. I fully endorse your work as practitioner historian. You are going to continue to do amazing work in the needy couple years! Thanks for this piece.