Prizes, complexity, security [TWIE]
The Week In Examples #40 | 15 June 2024
The big news this week, which I won’t be writing about, was Apple announcing a plan to integrate large language models into its products. The move was greeted with optimism from financial markets, which saw the company briefly become the world’s most valuable firm. Here’s a good explainer of why the news matters, and a more technically-minded analysis for those interested in that sort of thing.
For me, though, this time around I looked at the launch of a prize to encourage the development of an AI system good at solving novel problems, what new mathematics can tell us about the past and future of AI, and a recent report getting to grips with AI, catastrophic risks, and national security. As always, get in touch at hp464@cam.ac.uk with things to include, comments, or anything else.
Three things
1. Eyes on the AGI prize
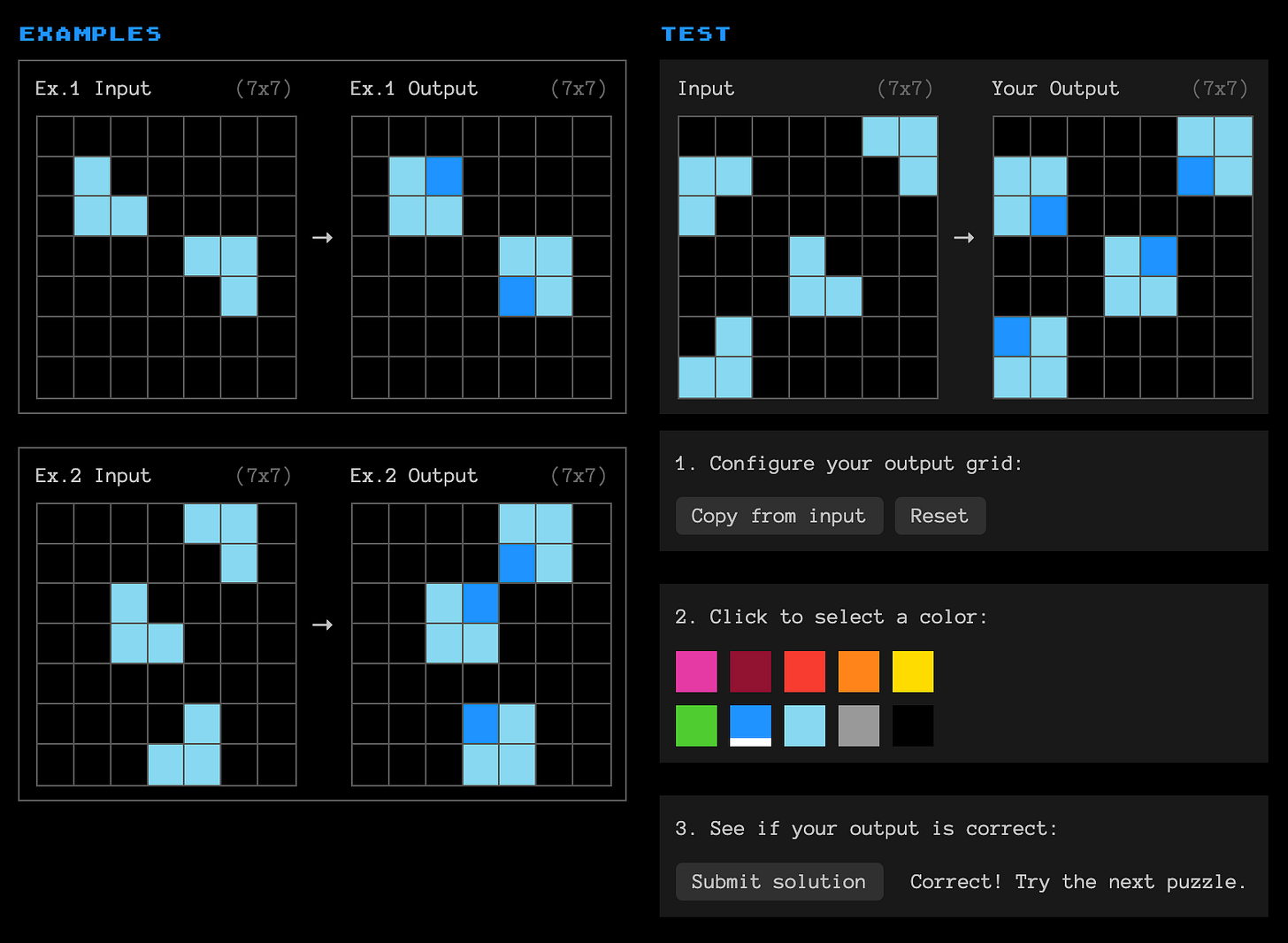
Entrepreneur Mike Knoop and deep learning researcher François Chollet launched a competition to solve an AI reasoning test: the Abstraction and Reasoning Corpus for Artificial General Intelligence (ARC-AGI). The benchmark, which was released in 2019, has remained stubbornly difficult for AI models to crack (including for large language models that power the likes of OpenAI’s ChatGPT, Anthropic’s Claude, and Google’s Gemini).
Knoop and Chollet hope that will change with the release of a $1M prize fund. The pair reckon that an average human score is in the region of 85 points, while the best AI models currently score less than 40. Given that dozens of new benchmarks are released each month you may wonder why this test is special. That’s a fair question. The answer is that most evaluations that test AI capabilities (e.g. language understanding, mathematical ability) have tumbled against the march of large language models.
That hasn’t happened for ARC-AGI for two reasons. First, the nature of the challenge itself. Chollet designed the test to include novel problems, which means—even ingesting massive chunks of the internet—large models are unlikely to have seen anything like them before in their training data. The upshot is that, according to Chollet, because language models only apply existing templates to solve problems, they get stuck on tests that human children would be able to manage comfortably. Second, the test isn’t particularly well known, which has narrowed the pool of researchers trying to solve it relative to popular benchmarks.
The (quite literally) million dollar question is whether ARC-AGI will stand the test of time. If I had to guess, I would expect major progress on the challenge within the next year or so. This is because a) bigger models with some clever algorithmic improvements seem to be doing something other than simple pattern matching; and b) there’s already been some improvement on the benchmark since it was released. Whatever the case, we’ll have an answer soon enough.
2. A very short history of complexity
A fascinating truism about the natural world is that simple units tend to work together to create complexity. These emergent phenomena—like the murmuration of birds or particles in Jupiter’s atmosphere that formed the Great Red Spot—exist as large-scale patterns that arise from interactions between individual parts. The behaviour of complex systems can be described as emergent if it can’t be predicted from the properties of simple units alone, but it's not all that clear when these patterns appear or how to determine what counts as emergent behaviour.
Recent work hopes to change that. Last week, a group led by the University of Sussex updated a paper from February this year introducing a computational approach to understanding emergent phenomena in complex systems. The central idea behind the work is that of ‘causal closure’ through which a system’s future can be predicted using only its own past states. According to the authors, this is similar to how software runs on a computer: the software's behaviour is determined by its own logic and inputs, and the specific details of electronic circuitry don't matter as long as it supports the software's requirements.
Complexity emerging from simple units is more or less the story of today’s large models. They are, at their core, made up of millions of simple components that work together to create something more than the sum of their parts. This is why researchers say large models are “unreasonably effective” — though in many ways this dynamic has always been central to AI’s development.
First, Nicolas Rashevsky established a mathematical foundation for understanding biological systems in the 1930s, then McCulloch and Pitts created a simplified mathematical model of biological neurons in the 1940s, and Frank Rosenblatt introduced a learning rule for these models in the 1950s.
Over the next few decades, artificial neural networks got bigger. But they also got better. Bell Labs researcher John Hopfield described emergent properties of the models in his influential 1982 paper: “Computational properties of use to biological organisms or to the construction of computers can emerge as collective properties of systems having a large number of simple equivalent components (or neurons).” Forty years later, the story we tell ourselves is known as the bitter lesson: “general methods that leverage computation are ultimately the most effective, and by a large margin”.
3. A crisis waiting to happen
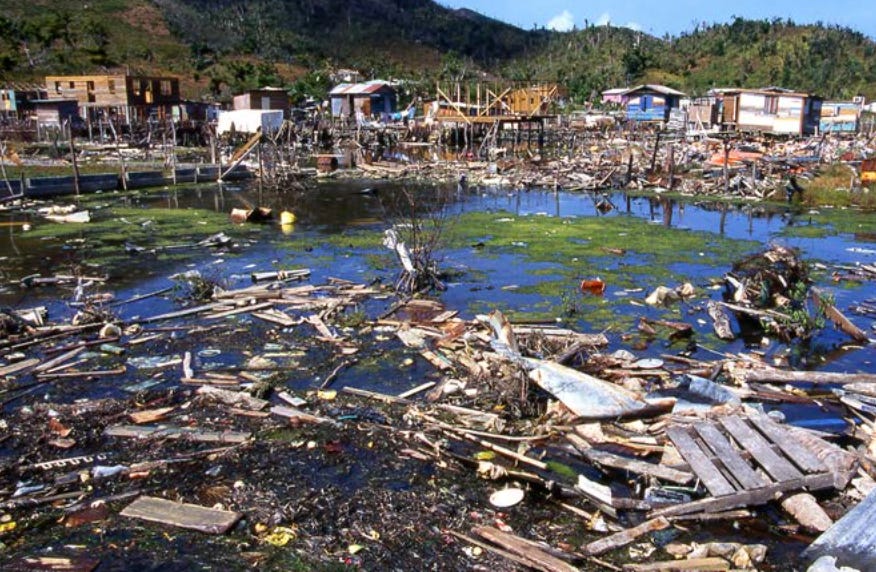
A report from the Center for a New American Security looks at the potential for AI to cause a range of ‘crises’. It argues that crises can be broadly placed into three buckets: small scale crises that demand local or regional responses, catastrophic events that require the highest levels of state response, and existential risks “threatening to overwhelm all states’ ability to respond, resulting in the irreversible collapse of human civilization or the extinction of humanity.”
The ability of a state to respond is core for making these distinctions, with the authors suggesting that a hurricane may cause a catastrophe in one country (for example, Hurricane Mitch in South America in 1998) while resulting in a smaller scale crisis in another.
The report distinguishes between AI’s potential for catastrophic risk (e.g. bioweapons, financial crashes, or accidental military escalation) and existential risk (e.g. losing control of a powerful system that threatens humanity’s survival). It argues that while existential risks have stolen the headlines, catastrophic risks are more relevant for near-term national security (and should be taken seriously by the national security establishment).
At a conceptual level, the use of ‘crisis’ is an interesting frame of reference. After all, the medical definition of a crisis is "the point in the course of a disease at which a decisive change occurs, leading either to recovery or to death." By this account, a crisis isn’t a period of damage, decline, or deterioration — it’s an inflection point. In the 1980s, the English author Samuel Brittan borrowed the concept to explain the structural changes that were taking place as some western countries shed their socially democratic character in favour of a model centred on free market individualism.
I think this metaphor holds for AI. Yes, AI has the potential to cause small scale, catastrophic, and even existential types of crises. But, to take a leaf out of Brittan’s book, our current moment can also be viewed as one of crisis. Whether we have the capacity to steer AI’s development in beneficial directions (or whether the technology overruns the state’s capacity to effectively respond) may prove to be the question of the coming years and decades.
Best of the rest
Friday 14 June
Towards Bidirectional Human-AI Alignment: A Systematic Review for Clarifications, Framework, and Future Directions (arXiv)
Preparing for an inevitable AI emergency (Fulcrum)
The AI bill that has Big Tech panicked (Vox)
Photographer takes on the machines in AI competition – and wins (The Guardian)
AI candidate running for Parliament in the U.K. says AI can humanize politics (NBC)
Sunak to tell G7 reform needed to deliver sustainable development and AI (The Standard)
AI startup Perplexity wants to upend search business. News outlet Forbes says it's ripping them off (ABC)
Thursday 13 June
The Neo-Brandeisians Are Half Right (Law and Liberty)
Can LLMs invent better ways to train LLMs? (Sakana AI)
AI Ethics and Governance in Practice (Alan Turing Institute)
Dream Machine (Luma Labs)
OpenAI expands lobbying team to influence regulation (FT)
More AI Founders Looking to Sell Startups, Hugging Face CEO Says (Bloomberg)
How Amazon blew Alexa’s shot to dominate AI, according to more than a dozen employees who worked on it (Fortune)
Wednesday 12 June
Making AI Intelligible: Philosophical Foundations (arXiv)
Consumer privacy at OpenAI (OpenAI)
Large Language Models Must Be Taught to Know What They Don't Know (arXiv)
Google Gemini, explained (The Verge)
Challenges in red teaming AI systems (Anthropic)
Apple’s belated AI gambit (FT)
Tuesday 11 June
AI for the rest of us (Interconnects)
Delving into ChatGPT usage in academic writing through excess vocabulary (arXiv)
A virtual rodent predicts the structure of neural activity across behaviors (Nature)
Apple is putting ChatGPT in Siri for free later this year (The Verge)
Meta will use your social media posts to train its AI. Europe gets an opt out (The Register)
The AI upgrade cycle is here (The Verge)
Lee bill would ban FCC from AI rulemaking (Axios)
Nvidia Conquers Latest AI Tests (IEEE Spectrum)
Monday 10 June
Evaluating the persuasive influence of political microtargeting with large language models (PNAS)
Award for Scholarship on AI and Liability (Carnegie Endowment for International Peace)
AI Search: The Bitter-er Lesson (Notion)
Why Has Predicting Downstream Capabilities of Frontier AI Models with Scale Remained Elusive? (arXiv)
What should an AI's personality be? (Anthropic)
OpenAI and Apple announce partnership to integrate ChatGPT into Apple experiences (OpenAI)
First NHS physiotherapy clinic run by AI to start this year (The Guardian)
AI Tools Are Secretly Training on Real Images of Children (WIRED)
Guterres warns humanity on ‘knife’s edge’ as AI raises nuclear war threat (The Guardian)
Ukraine’s Vision of Robot Assassins Shows Need for Binding AI Rules (Bloomberg)
Job picks
Some of the interesting (mostly) non-technical AI roles that I’ve seen advertised in the last week. As usual, it only includes new positions that have been posted since the last TWIE (but lots of the jobs from the previous edition are still open).
Research Fellow, GovAI (Oxford, UK)
Research Scientist, Responsible and OpenAI Research, Microsoft (Seattle)
Team Manager, Interpretability, Anthropic (US)
Director of AI and Democracy, Aspen Digital (Washington D.C.)
Research Scholar, Special Projects, GovAI (Oxford, UK)