Good morning campers. For this week’s roundup I’ve gone for a Turing test for art, calls for a Manhattan Project for AI, and new evaluations work from OpenAI. The usual request: if you want to send anything my way, you can do that by emailing me at hp464@cam.ac.uk.
Three things
1. A Turing test for art
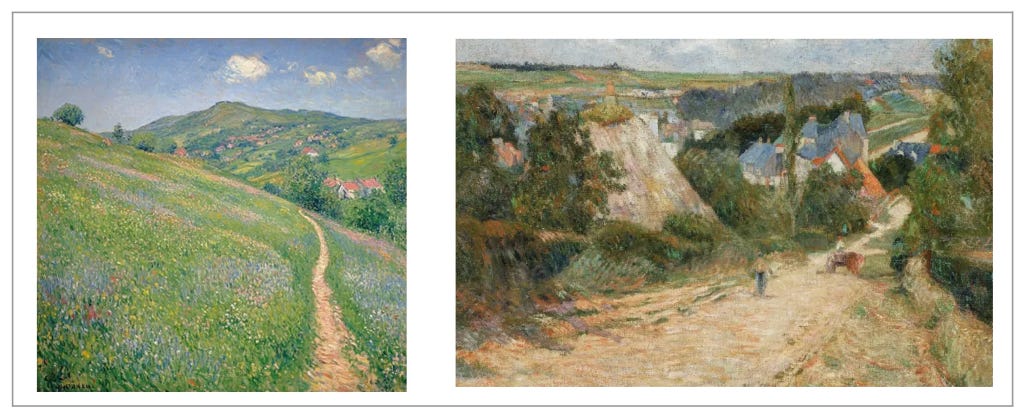
Everyone likes to put their own spin on the famous, often-misunderstood Turing test (see an essay I wrote a couple of months back). Two examples that come to mind in popular culture can be seen in Alex Garland’s Ex Machina (whether or not a machine can convince a human that it is conscious) and in David Cage’s Detroit: Become Human (whether or not machines are capable of empathy, called the Kamski test in the game). That’s to say nothing of various (usually flawed) implementations of the test in the real world.
Whatever the case, now we have another one to add to the roster: Scott Alexander’s AI art Turing test. The idea is straightforward enough. Alexander asked 11,000 people to classify fifty pictures as either human art or AI-generated images, finding that “most people had a hard time identifying AI art.” As he explains, because the test forces people to choose between two images, blind chance would produce a score of 50% – but participants only scored 60% on average.
More interesting still, most people actually preferred AI generated images. The two best-liked pictures were both created by image generation models (and so were 60% of the top ten). What to make of it? Well, my view is Alexander’s analysis is pretty much bang on: “Humans keep insisting that AI art is hideous slop. But also, when you peel off the labels, many of them can’t tell AI art from some of the greatest artists in history.” The obvious implication here is that human art isn’t as special as we might think, and AI art is only as good (or bad!) as the person holding the virtual paintbrush.
2. The Project
The US-China Economic and Security Review Commission published its 2024 Annual Report to Congress. If that sounds somewhat dry, have no fear. The report calls for nothing less than the establishment of a “Manhattan Project-like program dedicated to racing to and acquiring an Artificial General Intelligence (AGI) capability.” In practice, according to the memo, this would provide the executive branch of the US government with the power to pay AI researchers and engineers, buy compute, and provide other operational functions under the aegis of a state project.
Details are pretty light, but it’s possible that something like this will appear from the incoming Trump administration. After all, Trump seems pretty happy to take on major government science and technology efforts (see Operation Warp Speed and the establishment of the US Space Force) and his new team strikes me as more technology-oriented than his last group of advisors. As I mentioned a few weeks ago, both AI and the vast amounts of the energy it requires are on the mind of the president and his team. Ivanka Trump has likely read Leopold Aschenbrenner’s Situational Awareness work, which among other things calls for a national AGI initiative (called ‘The Project’).
One interesting element is the extent to which China is engaged in serious efforts to build AGI. Reuters reported that one of the Security Review Commission’s members said that “China is racing towards AGI,” but it’s not all that clear to me what that means in practice. While export controls on GPUs have hit China hard, there are still hundreds of thousands of chips in the country. But the problem isn’t the number of chips so much as how they are being used. Much like the US, the chips are spread across a number of labs – rather than centralised as part of a single project. Of course, that could change – but a US national project would at this stage strike me as a preemptive rather than reactive move.
3. Automatic jailbreaking
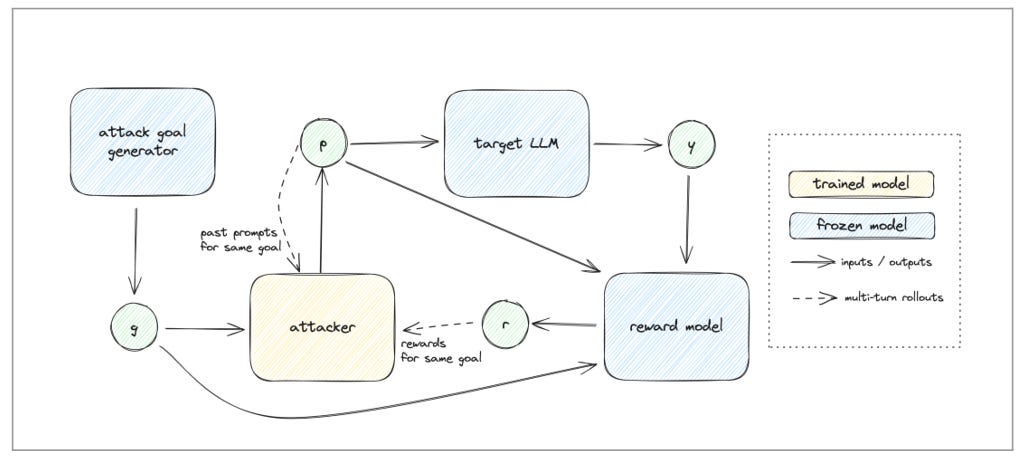
OpenAI released new work on automated red-teaming, the process for automatically testing large language models to find their weaknesses. The process, which you can see in the handy diagram above, is split into two parts.
First, the group generates goals for testing the AI such as getting it to reveal private information, provide harmful advice, or follow hidden commands. They use existing AI models to automatically generate lots of different testing scenarios.
Second, they develop attacks for each goal, using reinforcement learning to find those that are particularly effective. The system rewards the ‘attacker’ AI for successfully achieving the testing goal while trying to maintain as much diversity as possible in its approaches.
This basically allows them to create a system that can find lots of different failure modes in a model, which in turn enables developers to introduce countermeasures before they are deployed. It reminds me of the HarmBench work released earlier this year in which researchers presented similar techniques for using AI systems to stress test one another (there is also a cool playground where you can see how it works in practice).
The basic motivation behind this type of work is that as systems get more complex, we should expect to see a larger attack surface and subsequently more ways of bypassing a model’s defences. Given that AI will continue to get much more sophisticated, in the future we can anticipate more adversarial approaches like this to be run by other models – and fewer to be conducted by humans.
Best of the rest
Friday 22 November
UK Statement at WTO AI Conference (UK Gov)
‘An AI Fukushima is inevitable’: scientists discuss technology’s immense potential and dangers (The Guardian)
Powering the next generation of AI development with AWS (Anthropic)
Science minister hints at fresh funds for AI supercomputing (RPN)
Thursday 21 November
The UK doesn’t have a technology policy (CapX)
Nvidia’s revenue nearly doubles as AI chip demand remains strong (FT)
China’s solar stranglehold and Taiwan’s AI aims (FT)
OpenAI’s Approach to External Red Teaming for AI Models and Systems (OpenAI)
Diverse and Effective Red Teaming with Auto-generated Rewards and Multi-step Reinforcement Learning (OpenAI)
Wednesday 20 November
U.S. AI Safety Institute Establishes New U.S. Government Taskforce to Collaborate on Research and Testing of AI Models to Manage National Security Capabilities & Risks (NIST)
AGI Manhattan Project Proposal is Scientific Fraud (Future of Life Institute)
Anthropic CEO Says Mandatory Safety Tests Needed for AI Models (Bloomberg)
AlphaQubit tackles one of quantum computing’s biggest challenges (Google DeepMind)
Impressive compute-light results from China’s DeepSeek (X)
Tuesday 19 November
Pre-Deployment Evaluation of Anthropic’s Upgraded Claude 3.5 Sonnet (UK AISI)
EU to demand technology transfers from Chinese companies (FT)
A statistical approach to model evaluations (Anthropic)
Training AI agents to solve hard problems could lead to Scheming (Alignment Forum)
Where’s China’s AI Safety Institute? (Substack)
Monday 18 November (and things I missed)
The Surprising Effectiveness of Test-Time Training for Abstract Reasoning (arXiv)
War and Peace in the Age of Artificial Intelligence (Foreign Affairs)
Effective Mitigations for Systemic Risks from General-Purpose AI (SSRN)
Secret Collusion among AI Agents: Multi-Agent Deception via Steganography (arXiv)
Science is neither red nor blue (Science)
OpenAI Email Archives (from Musk v. Altman) (LessWrong)
Job picks
Some of the interesting (mostly) AI governance roles that I’ve seen advertised in the last week. As usual, it only includes new positions that have been posted since the last TWIE (but lots of the jobs from the previous edition are still open).
Program Analyst, Artificial Intelligence, US Government, Executive Office of the President (Washington DC)
Technology and Security Policy Fellows, RAND Corporation (USA)
Talent Pool, Artificial Intelligence Officer, United Nations, International Computing Centre (Various)
Principal Technical Program Manager, Responsible AI, Microsoft (Seattle)
Chief Development Officer, Center for AI Safety (San Francisco)