This week’s edition features calls for a National Transmission Highway Act based on the 1956 National Interstate and Defense Highways Act, new research looking at the safety implications of world models, and the first draft of the EU’s Code of Practice for AI. The usual plea: if you want to send anything my way, you can do that by emailing me at hp464@cam.ac.uk.
Three things
1. My way or the highway
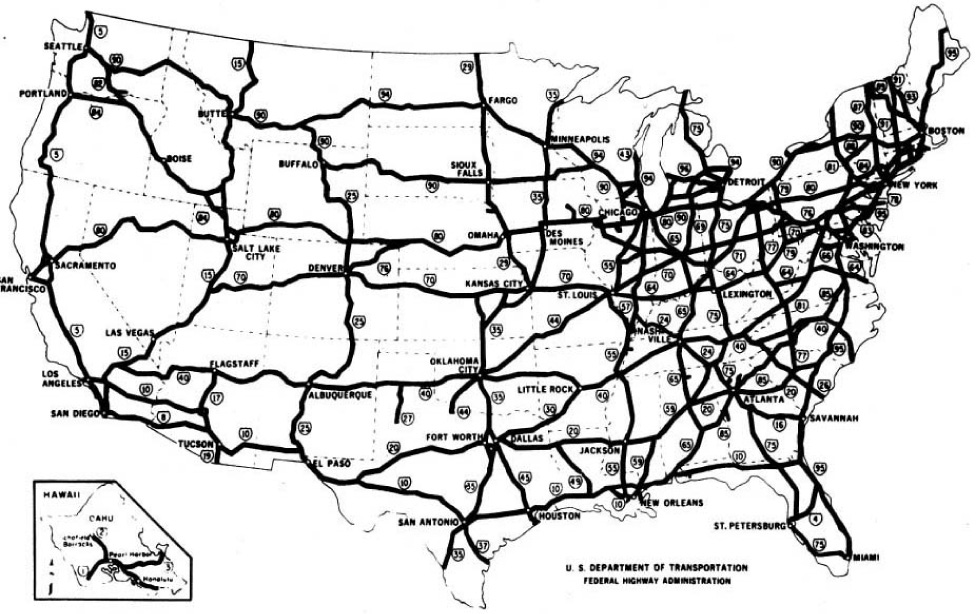
I wrote a bit about AI infrastructure in last week's edition in the context of the incoming Trump Administration. The long and short of it is that developers need clusters of GPUs and the energy to keep them buzzing, but it’s hard to build anything in much of the United States (and, of course Europe) due to a broken planning system. Now, according to reports, labs are pitching their own ideas for infrastructural reform to the US government.
There’s lots of interesting stuff in the report, but one element that stood out to me was a possible “National Transmission Highway Act” modelled after the 1956 National Interstate and Defense Highways Act in the United States. The idea is to create a web of ‘AI highways’ by building more power transmission lines, installing more fibre optic cables for data, and constructing natural gas pipelines for powering data centres.
The comparison to the 1956 National Interstate and Defense Highways Act, which established America's interstate highway system, is particularly telling. We’re talking about the largest public works project in U.S. history, which allocated over $215 billion in today’s money to construct 40,000 miles of highways across the country (primarily funded through a new Highway Trust Fund that collected taxes on gasoline and truck tyres from highway users).
An information highway is obviously not a real highway (though both have plenty of physical elements). The 1956 Act was about creating an efficient transportation network for civilian use, but it also sought to boost military mobility — including potential urban evacuation routes during the Cold War. At least in this sense, as the national security aspect of AI becomes more pronounced, a “National Transmission Highway Act” has something in common with its older counterpart.
2. Safe world models
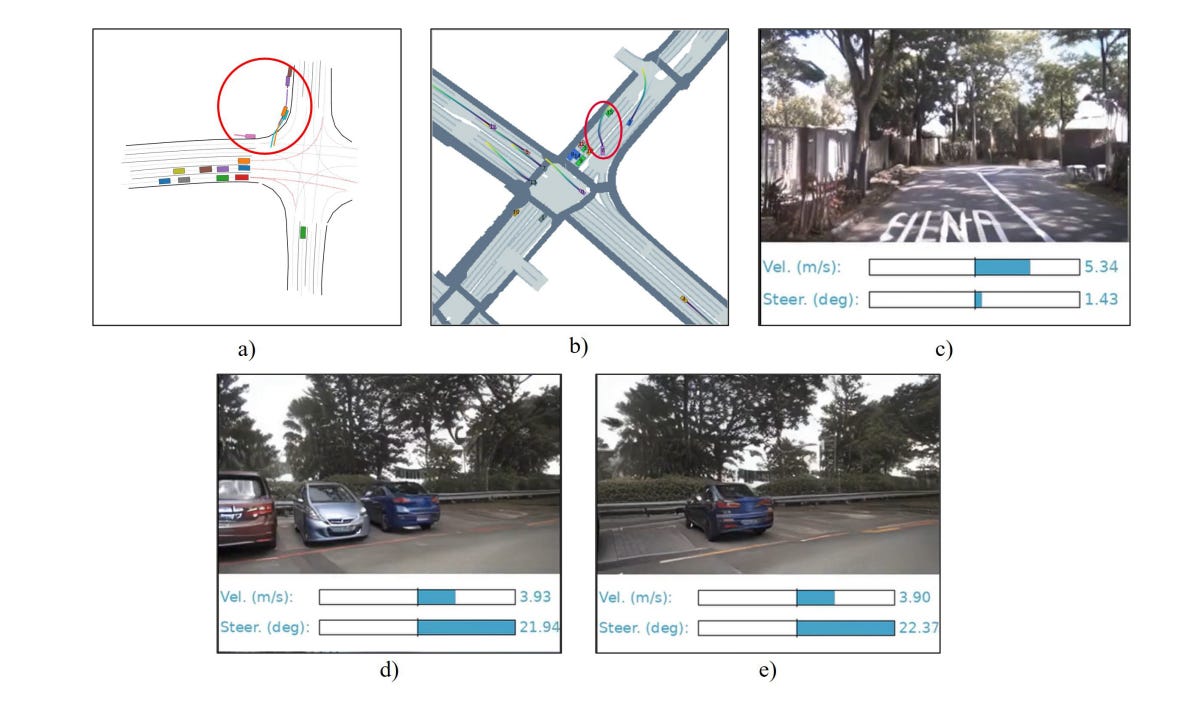
Researchers based in China, Germany, and France conducted a review of world models in AI systems, focusing on their safety implications for embodied AI agents like autonomous vehicles. In this context, a world model is an AI system's internal representation of how the world works and how it changes over time. We can broadly think of it as a model’s ability to predict what happens next based on what it observes (sort of like how humans use common sense to predict how situations will unfold). In autonomous driving, for example, a world model helps the car predict how other vehicles will move, how pedestrians might behave, or how road conditions affect driving.
Tracing the evolution of world models from games to real-world applications (in many ways the story of AI), the paper highlights a few prominent safety issues related to the systems. The group is primarily interested in world models for autonomous driving applications, looking at reasonable scenario generation (like vehicles placed outside drivable areas), temporal inconsistencies in generated videos (vehicles disappearing between frames), and unsafe behaviour of AI agents in interactive scenarios (when a model makes a dangerous mistake).
The group basically argues that these issues happen because world models don't truly comprehend the underlying rules of the real world in a way that humans do. This is one of the major criticisms faced by large language models like ChatGPT: they exist simply as large-scale pattern matching machines that can’t reason, reflect or understand. I don’t actually buy this interpretation of LLMs, so I do wonder how intractable these problems will prove to be over the long term for autonomous driving and beyond.
3. Practice makes perfect
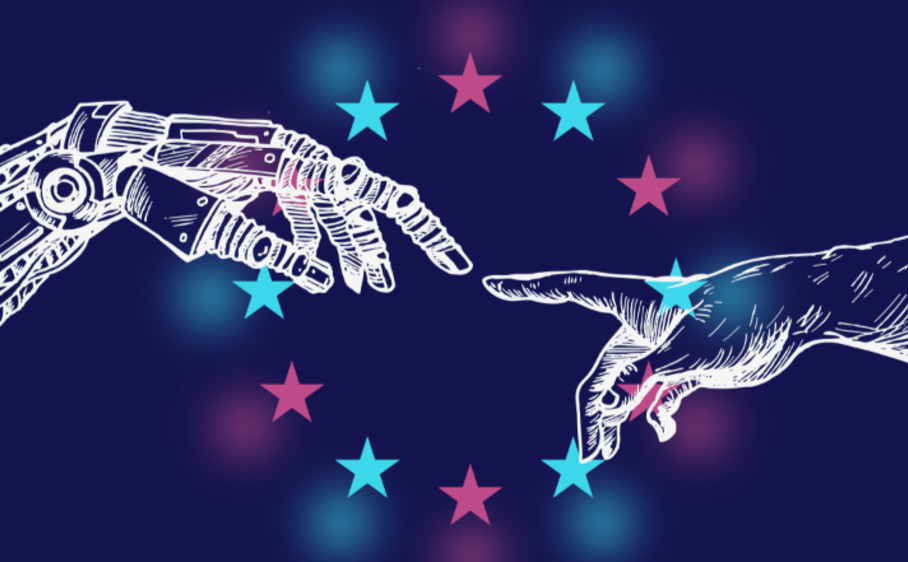
Stakeholders everywhere rejoice. The EU has released the first draft of its General-Purpose AI Code of Practice, a framework developed as part of the mighty EU AI Act. This draft, released earlier in the week, establishes guidelines for providers of general-purpose AI models, with special focus on those presenting ‘systemic risks’ (i.e. risks to society writ large). The Code of Practice, which will eventually determine how the AI Act is implemented, is the output of four working groups focusing on transparency, risk assessment, technical risk mitigation, and governance risk mitigation.
The draft makes for interesting reading (providing you’re into lawyerly writing about AI). It introduces a formal taxonomy of AI systemic risks, establishes requirements for continuous risk assessment and mitigation, and creates new documentation requirements including Safety and Security Frameworks (SSFs) and Safety and Security Reports (SSRs). The framework emphasises proportionality: requirements scale with both the size of the provider and the severity of potential risks. As they put it in the text, “obligations applicable to providers of general-purpose AI models should take due account of the size of the general-purpose AI model provider and allow simplified ways of compliance for SMEs and start-ups.”
At a high level, it looks like the Code of Practice is a bit more lenient than the text of the AI Act itself. This probably shouldn’t be that surprising given that in the time since the AI Act was originally tabled, the EU Commission has engaged in a bit of soul searching. The Draghi report, for example, made it clear that senior EU figures accept that if Europe wants to remain competitive globally—and even think about closing the gap with the US—it needs to do more than just regulate.
Best of the rest
Friday 15 November
When AI assistants leak secrets, prevention beats cure (The Register)
When it comes to AI, maybe we’re not as screwed as we think we are (The Drum)
Good analysis of whether scaling is slowing down (X)
People prefer AI-generated poems to Shakespeare and Dickinson (New Scientist)
Thursday 14 November
Scaling realities (Substack)
First Draft of the General-Purpose AI Code of Practice published, written by independent experts (EU)
Reasons that AI Can Help with AI Governance (Substack)
Here's What I Think We Should Do (Substack)
Wednesday 13 November
The AI Safety Institute reflects on its first year (UK AISI)
Dwarkesh Patel interview with Gwern (X)
The EU AI Act is a good start but falls short (arXiv)
Apollo is adopting Inspect (Apollo Research)
OpenAI to present plans for U.S. AI strategy and an alliance to compete with China (CNBC)
Announcing Inspect Evals (UK AISI)
Tuesday 12 November
Rapid Response: Mitigating LLM Jailbreaks with a Few Examples (arXiv)
AI Policy Considerations for the Trump Administration: Part I - Navigating Innovation and Safety (Substack)
Fair Summarization: Bridging Quality and Diversity in Extractive Summaries (arXiv)
A parody of venture (Substack)
A Social Outcomes and Priorities centered (SOP) Framework for AI policy (arXiv)
Monday 11 November (and things I missed)
A Collection of AI Governance Research Ideas (Markus Anderljung)
How Foundation Models Could Transform Synthetic Media Detection (CFI)
AI protein-prediction tool AlphaFold3 is now open source (Nature)
Percy Liang on truly open AI (Substack)
Review I wrote of ‘Neural Networks’ book (H-Net)
Key questions for the International Network of AI Safety Institutes (IAPS)
Job picks
Some of the interesting (mostly) AI governance roles that I’ve seen advertised in the last week. As usual, it only includes new positions that have been posted since the last TWIE (but lots of the jobs from the previous edition are still open).
Policy Officer, Emerging Technologies Governance, Paris Peace Forum (Paris)
Associate Editor, Tech Policy Press (Global)
Senior Associate, AI Governance, The Future Society (Global)
Operations Associate, Center for AI Safety (San Francisco)
Heron Program for AI Security (Global)