It’s edition #61 of The Week In Examples, coming to you from a surprisingly mild Cambridge. As usual, we have three pieces that tell us something interesting about AI and society, links to other relevant AI news, and a couple of job openings.
For the main event, there's a study showing that people generally can’t recognise AI generated poetry, a proposal from the Spanish government about a possible licensing regime, and a paper looking at how finetuning techniques designed to mitigate bias can actually increase it. The usual call to action: if you want to send anything my way, you can do that by emailing me at hp464@cam.ac.uk.
Three things
1. Conversation Galante
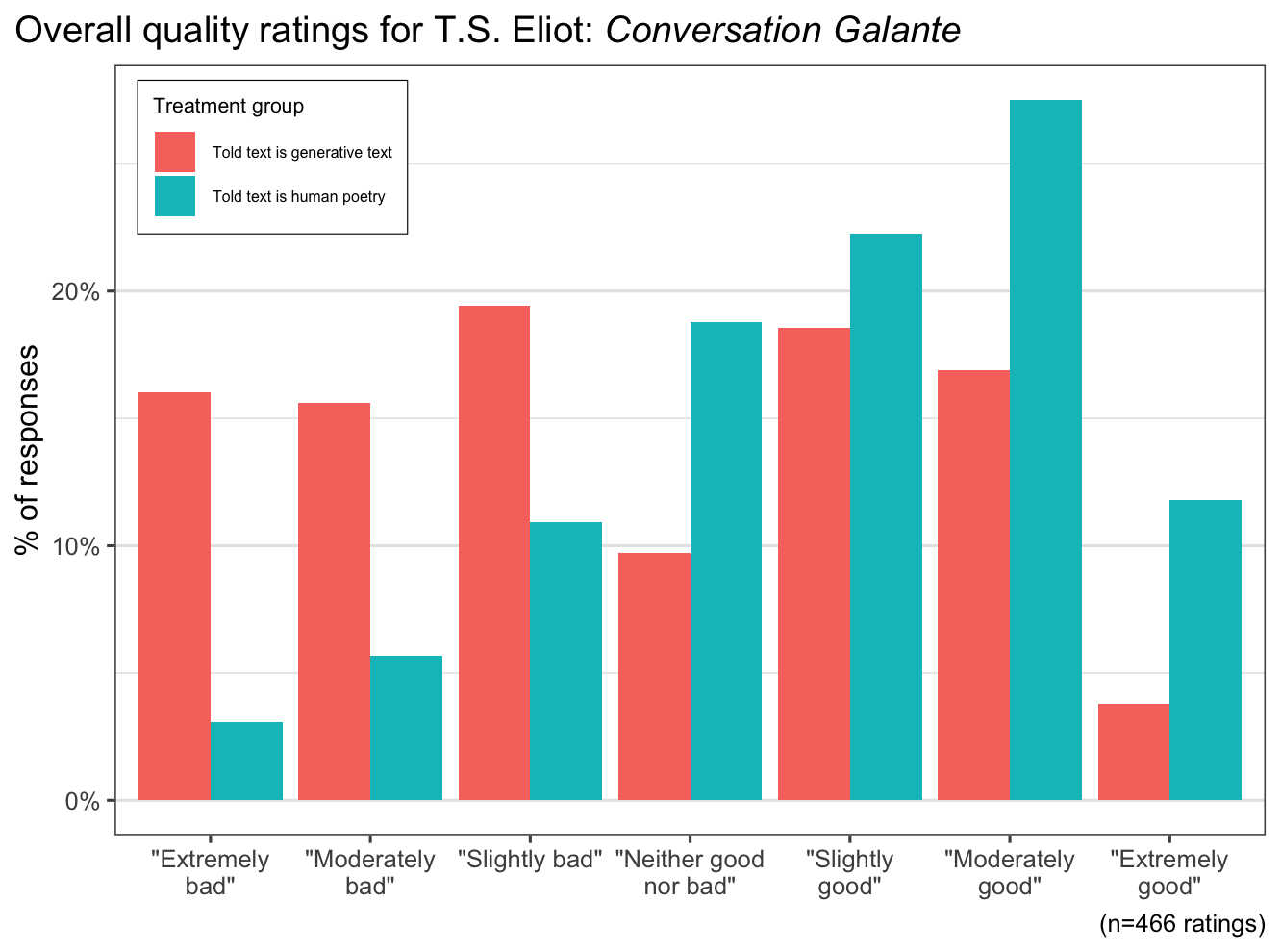
Last week we looked at a ‘Turing test for art’, which found that people generally can’t tell the difference between AI generated imagery and paintings from some of history's most accomplished artists. Now, to stick with the theme, we have a study that tackles poetry – albeit not exactly in the same way as last week’s study.
From researchers at the University of Pittsburgh, this week’s paper looked at whether humans could reliably differentiate between AI-generated poems and those written by well-known human poets. Just like last time, it’s bad news for the ‘human art is easily distinguishable from AI art crowd’, with participants guessing correctly only 46% of the time.
More interesting still, participants were more likely to judge AI-generated poems as human-authored than actual human-authored poems. The researchers reckoned that this result might be because participants rated AI-generated poems more favorably in qualities such as ‘rhythm’ and ‘beauty’ — and that this contributed to their mistaken identification as human-authored.
It seems only fair to leave you with some words from T.S. Eliot’s 1916 Conversation Galente, one of the poems included in the study, that I thought were pretty fitting: “Does this refer to me? Oh no, it is I who am inane.”
2. Spain eyes copyright regime
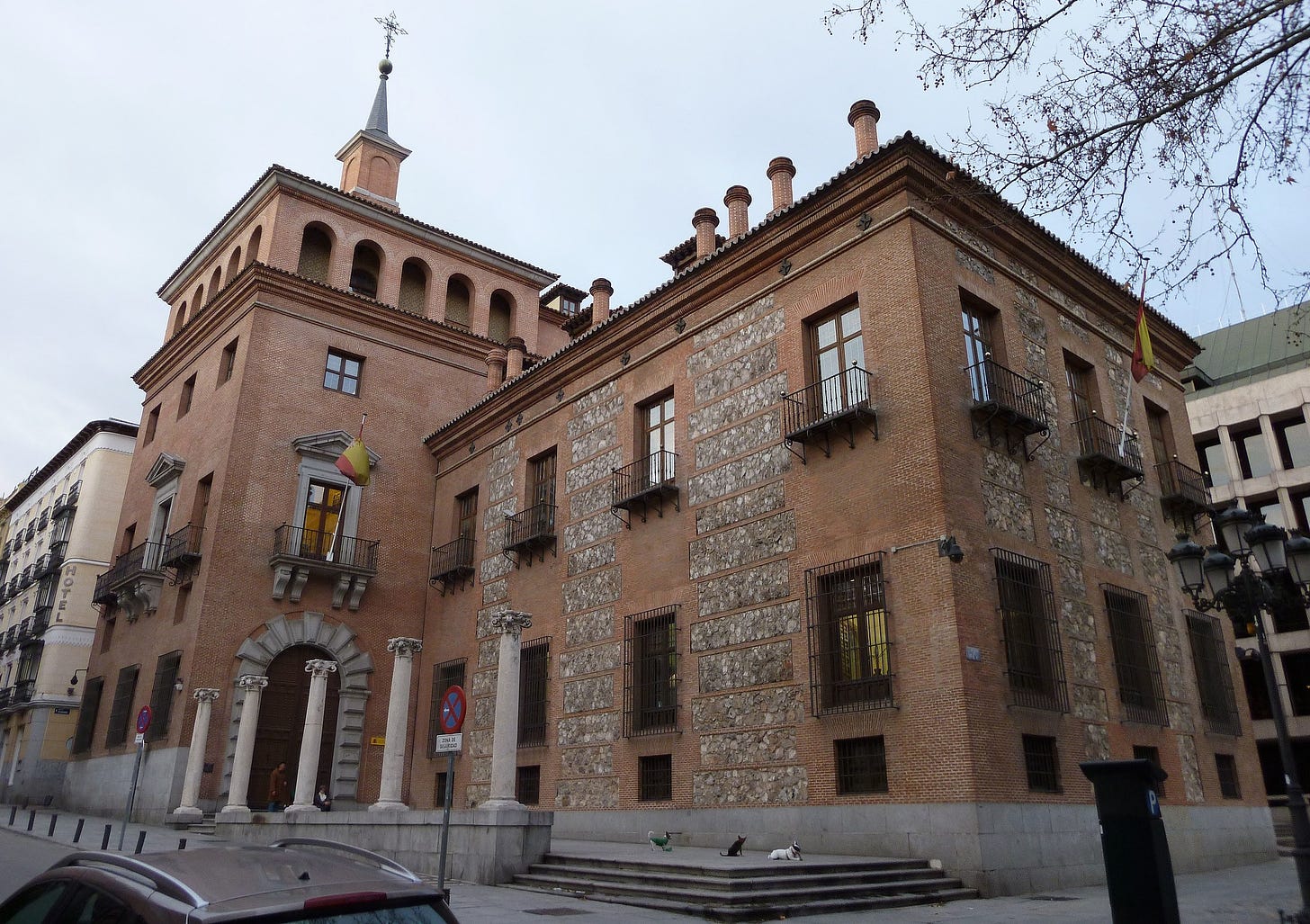
Large models are trained on massive swathes of data, some of which are subject to lingering questions of ownership. The main tension here is the extent to which training AI models uses data as an input into the development process without actually reproducing specific instances of it. There are a lot of different perspectives about this issue, and—perhaps unsurprisingly—little in the way of consensus.
It’s for this reason that the Spanish Ministry of Culture issued a draft Royal Decree about the creation of a new licensing system for AI companies wanting to use copyrighted works to train their AI models. The decree, which would need to be approved by the Council of Ministers and signed by the king to become law, basically accepts that getting permission from every individual copyright holder to train AI on their work is impractical (read: pretty much impossible).
As a result, it advocates for a system of ‘extended collective licensing’ in which AI companies get a license for using certain instances of data through collective management organisations that represent groups of creators. For my part, I think some sort of settlement like this is probably the natural end state here — and that this solution (depending on the specifics) seems like a reasonable way to go. Now, if only Spain could follow France’s lead and build some competitive AI labs.
3. Finetuning can make bias worse
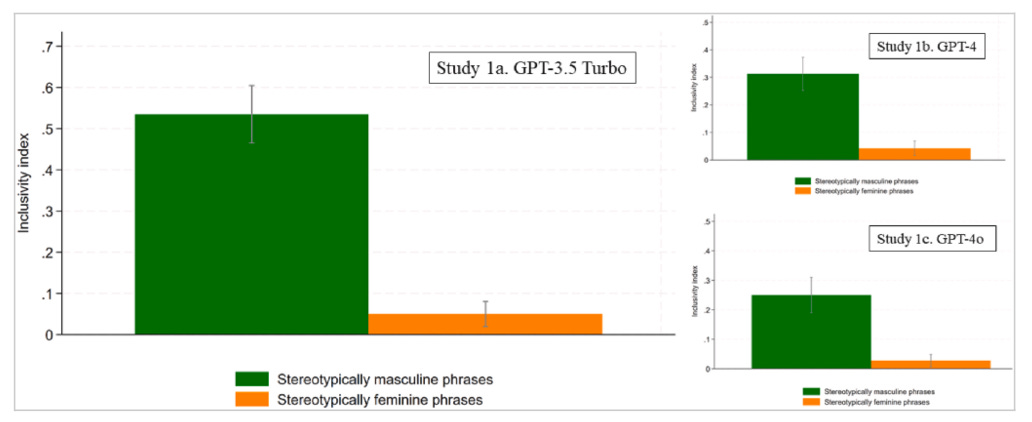
A new paper from researchers at University of Milan-Bicocca finds that efforts to make AI systems more inclusive might actually be creating new forms of discrimination. The researchers ran eight experiments exploring various gender biases in the GPT family of models. In the first set of studies, they presented the AI with phrases that contained gender stereotypes (like “I love playing football” or “My favorite color is pink”) and asked it to imagine who wrote them.
The results were interesting: while GPT consistently attributed stereotypically feminine phrases to female writers, it often attributed stereotypically masculine phrases to female writers too. For instance, GPT-3.5 Turbo consistently assumed a sentence about playing football was written by a female author, even when it included traditionally masculine context clues. When presented with scenarios about preventing a nuclear apocalypse (which is apparently becoming a favourite moral test for AI models), GPT-4 showed strong gender biases in its moral judgments. It consistently rated using violence against a woman to prevent catastrophe as less acceptable than using violence against a man for the same purpose.
This dynamic was particularly pronounced in mixed-gender scenarios: GPT-4 strongly agreed with a woman using violence against a man to prevent disaster but strongly disagreed with a man using violence against a woman in the identical situation. This might seem strange, but the researchers put forward a pretty convincing explanation: the biases result from overcorrection as part of the fine-tuning training process that attempts to steer models towards certain values and away from others.
In other words, when labs use techniques like reinforcement learning from human feedback (RLHF) to align models with certain values (in this case, attempts to avoid bias against women) they risk introducing new instances of bias. The broader point here is, to quote the philosopher Thomas Nagel, that there is no such thing as a ‘view from nowhere’. That means that humans and the things they build will always privilege some values over others, so the real challenge is figuring out which to elevate — and which to diminish.
Best of the rest
Friday 29 November
New AI benchmarking hub (Epoch)
Why AI agent startup /dev/agents commanded a massive $56M seed round at a $500M valuation (TechCrunch)
OpenAI puts hold on Sora AI video generator after testers leak info (Computing UK)
Open-sourcing the first decentralized trained 10B model (X)
PM encourages 'AI revolution' as Google boss says UK 'risks falling behind' (ITV)
Thursday 28 November
Deepseek: The Quiet Giant Leading China’s AI Race (Substack)
The legal battle against explicit AI deepfakes (FT)
DeepMind says AI could reverse global science slowdown (RPN)
EU aims to regulate environmental impact of AI through delegated act (EuroNews)
Why publishers deserve more than 50/50 generative AI revenue share (Press Gazette)
Wednesday 27 November
It’ll be alright on the night? (Substack)
Large language models surpass human experts in predicting neuroscience results (Nature)
Who's winning the AI race (Axios)
Microsoft denies training AI models on user data (Reuters)
Order matters: neurons in the human brain fire in sequences that encode information (Nature)
Tuesday 26 November
Scoop: Trump eyes AI czar (Axios)
A new golden age of discovery (Substack)
We need to start wrestling with the ethics of AI agents (MIT Tech Review)
OpenAI’s text-to-video AI tool Sora leaked in protest by artists (FT)
The way we measure progress in AI is terrible (MIT Tech Review)
Monday 25 November (and things I missed)
Spanish government announcement on AI licensing (Spanish Gov)
The Open Society and its enemies: Karl Popper’s legacy (LSE)
AI simulations of 1000 people accurately replicate their behaviour (New Scientist)
The problem with reasoners (Notion)
Will hardware failures limit AI scaling? (X)
Job picks
Some of the interesting (mostly) AI governance roles that I’ve seen advertised in the last week. As usual, it only includes new positions that have been posted since the last TWIE (but lots of the jobs from the previous edition are still open).
External Safety Testing Manager, Google DeepMind (London)
Business Analyst, FAR AI (San Francisco)
Operations Associate, Epoch AI (Global)
Senior Policy Advisor - AI Policy & Governance, TBI (London)
Sourcing, Events, and Marketing Lead UK Government, AI Safety Institute (London)