Quick reminder: I’m testing the waters to see whether there’s a path towards working on this newsletter full time, with a goal of 100 pledges by May 31. So far we’re well on the way, but there’s still much farther to go. Pledges are a small but hugely important step towards allowing me to spend more time on this project. This is the best moment to help Learning From Examples if you like what you read here.
I’ll also be sending the second edition of the AI Histories series on Friday. These are short pieces (about 1,000 words) that deal with an important moment in AI history. This week’s will be about the man behind genetic algorithms.
On the night of January 7, 1610, Galileo Galilei walked onto the balcony and looked down the lens of a telescope. Tilting the device towards the biggest planet in the solar system, he spotted three stars near Jupiter and recorded their positions in a notebook.
He looked again for the same stars six days later, but this time their positions had shifted. For Galileo, there was one likely reason for the change: they weren’t stars at all but moons orbiting Jupiter. The Italian astronomer had long bought Copernicus’ theory that our planet was not the centre of the universe. Now he had proof.
The discovery delivered a blow for the Ptolemaic model of the universe, which held that every planet followed a groove in a transparent, concentric sphere around a stationary Earth. In the geocentric system, any new irregularity—be it retrograde motion or varying brightness—was patched with epicycles, deferents, and equants until the system groaned under its own complexity.
When the little fixes became so numerous, the system eventually became too complex to function properly. The episode is a good reminder that faulty systems don’t break right away, that models of the universe are just that: representations. When a system for representing reality begins to learn more from itself than from the world it was built to describe, that’s when you know its days are numbered.
The Ptolemaic model was created by Claudius Ptolemy, a Greco-Egyptian astronomer who lived in Alexandria in the 2nd century CE. Ptolemy synthesised centuries of Greek astronomy into a grand system described in the Almagest, which became the dominant model of the cosmos in Europe and the Islamic world for over 1,400 years.
The Earth held top billing at the centre of the universe, with the Sun, Moon, planets, and stars orbiting it in perfect circles. To account for the looping paths of planets, it introduced smaller circles, or epicycles, that rode on top of larger ones.
But the model was unstable. Each discrepancy in planetary motion prompted astronomers to add another epicycle or tweak the equant. Ptolemy’s framework was flexible, so much so that it basically overfitted the heavens.
The philosopher Thomas Kuhn, who we briefly discussed in last week’s essay, thought that what appealed to Copernicus was the power to jettison these contrivances from his intellectual framework. Kuhn famously believed that most science operates within a shared system of sense-making that tells us what questions are worth asking and sets basic guidelines for how to answer them.
But over time, if too many results don’t fit the model, confidence in the paradigm begins to wane. This eventually leads to crisis, which may be resolved by a revolutionary project that brings with it a new way of doing things and new problems to solve.
The Ptolemaic system collapsed when its own patch-work of mends had grown so baroque that it no longer looked credible. Only when those ‘fixes’ piled up did the older heliocentric schemes finally seem the more elegant alternative.
Illusionary collapse
Model collapse is one of those terms that people love to say. It’s often used erroneously, but for now I’ll refer to the definition formulated by Shumailov and colleagues in their 2024 paper:
‘Model collapse is a degenerative process affecting generations of learned generative models, in which the data they generate end up polluting the training set of the next generation. Being trained on polluted data, they then mis-perceive reality.’
The research caused something of a stir last year when it found that as models retrain on their own outputs, they lose contact with the true data distribution. For language models, they found the ‘perplexity score’ of a model (in this case a metric evaluating the likelihood of a sequence of words) worsened significantly when trained on the outputs from a previous version.
According to the group, while the original model fine-tuned with real data achieves a perplexity score of 34, the perplexity score for models trained for five epochs on generated data increases to between 54 and 62. (A higher figure indicates worse performance because the perplexity score essentially measures the inaccuracy of predictions.)
In other words, collapse happens when models forget the variability of reality and converge on a single point. Nature News called it a ‘cannibalistic’ phenomenon that spelled trouble for large models trained on synthetic data. Models pollute the internet with low quality data, which is fed back into the next generation as part of the training process.
But the devil is in the details.
The researchers trained on 90% synthetic data and 10% original data for each new generation of training. Compare this approach with a paper from Stanford in which synthetic data is added incrementally over time. In the first round the model uses no synthetic data, half of the data is synthetic in the second round, two thirds is synthetic in the third round, and three quarters is synthetic in the fourth round.
Using this method, the Stanford group found minimal evidence of model collapse. As a result, the authors said that the results ‘demonstrate that accumulating successive generations of synthetic data alongside the original real data avoids model collapse.’ Given that real usage is based on accumulation rather than replacement, the upshot is that model collapse probably doesn’t spell doom for AI’s prospects.
There’s a bit in Robert Zemeckis’ Contact where Jodie Foster tries to explain to Matthew McConaughey why his faith in god is misplaced. She evokes the philosophical precept ‘Ockham's Razor’ to which he replies ‘Hockam’s Razor? It sounds like a slasher movie.’
The idea is as well known as it is straightforward: all things being equal, the simplest explanation tends to be the most likely. It takes its name from William of Ockham, a 14th-century Franciscan friar who argued that the Scholastic method had become entangled in abstract distinctions. At the University of Oxford, Ockham found that theological debates often revolved around arguments that seemed more concerned with internal consistency than with observable reality.
In the Scholastic tradition of his time, philosophers engaged in elaborate debates about the nature of universals that he believed led to increasingly complex and self-referential arguments. Ockham didn’t care if ‘redness’ was a quality that existed independent of objects, or even if ‘humanity’ was a real essence shared by all people.
What he cared about was the specific red apple or the individual human being. The rest of it was decadent self-reference.
To convince his contemporaries, the English friar argued that many of the complex distinctions made by his peers were unnecessary. His insistence on paring down explanations to their bones was a response to the recursive character of Scholastic debates, which often built upon layers of prior commentary without re-evaluating foundational assumptions.
The Scholastic method had become so naval-gazing that it risked losing touch with the realities it aimed to explain. Ockham's push for simplicity was an attempt to recalibrate the intellectual model by grounding it in observable phenomena and logical clarity.
Ockham's frustration was about systems losing contact with reality. He saw a tradition that had become so fluent in its own language that it forgot what the words were for.
You can see a similar thing happening in art, where critics level that the most dreaded of artistic put-downs: derivative.
Take Parmigianino’s Madonna with the Long Neck, which opens this essay. Completed in 1540, the painting shows us the Virgin Mary with an unnaturally elongated neck, slender fingers, and an elegant posture that defies anatomical correctness.
Parmigianino's work parodies the compositions of his predecessors like Raphael and Leonardo da Vinci. A reaction to the naturalism of the High Renaissance, it distorts its subject to create a sense of elegance that transcends the real world.
Madonna with the Long Neck is an example of Mannerism. Where Renaissance masters like Leonardo, Raphael, and Michelangelo aimed for harmony, proportion, and balance grounded in nature, Mannerist painters embraced distortion, exaggeration, and artificiality.
I think of Mannerism as a kind of recursive practice, an early example of art reflecting on art. Mannerist painters quoted gestures, borrowed poses, and echoed compositions from their predecessors. Often for the better but sometimes for the worse.
For Parmigianino and his contemporaries, the visual grammar of the Renaissance had become so well understood that it was no longer a guide to seeing the world. Instead, it was a set of conventions to be played with, warped, and pushed to the edge of recognisability.
Some critics at the time were unsettled. Giorgio Vasari, a contemporary of Parmigianino’s, admired the Italian’s work. Others found the painting ‘mannered’ in the pejorative sense in that it was stylised for its own sake.
The line that Mannerism walks is the one between homage and parody and refinement and artifice. It’s a reminder that the boundary separating art and slop, original and derivative, is obvious to some but invisible to others.
The feature map and the territory
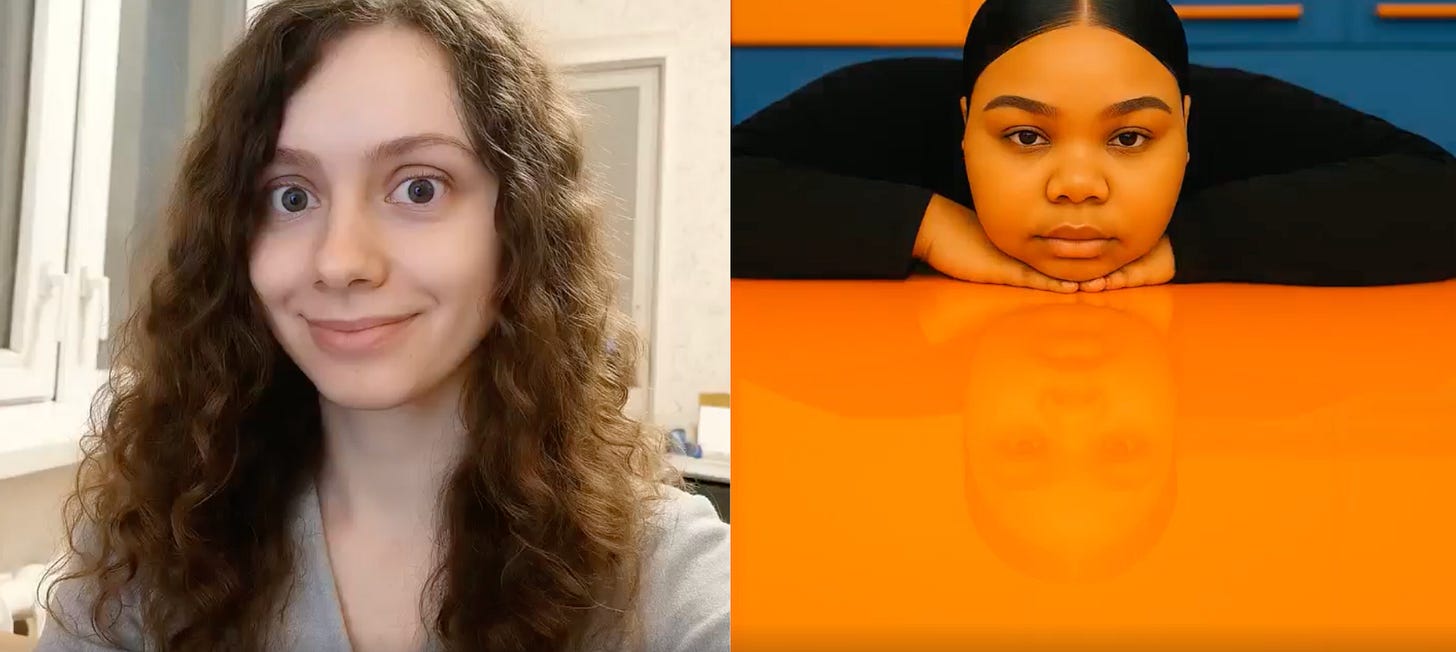
The French philosopher Jean Baudrillard famously described the modern world as one of simulacra in which representations take on a life of their own. In Baudrillard’s hyperreality, models circulate without stable points of reference and the map precedes the territory.
What he meant was that signs point only to other signs. The meaning of a photo, an advert or a film is borrowed from other media. We consume representations of the world and then representations of those representations. In Baudrillard’s words, the ‘hyperreal’ is when the simulation is more dominant than the real thing.
Like most people familiar with the Frenchman, I find Baudrillard at best head-spinning and at worst frustrating. But his work is useful to understand the relationship between model and meaning, copy and original. It helps us see that, like the Ptolemaic model, imitation looks like stability right before it leads to collapse.
Part of the problem concerns the relationships within a system. Ian Hacking’s ‘looping effect’, for example, shows how feedback moulds relations to allow original category (A) to become perceived category (B) solely through the actions of those who perceive it as (B).
Hacking noted that when people are labelled (say, with respect to a psychiatric condition), they may alter their behaviour in light of that classification. As a result, ‘categorizing people opens up new ways to think of themselves, new ways to behave, and new ways to think about their pasts’.
The very act of modelling changes the modelled. In economics, George Soros has made the same point under the name of reflexivity wherein market participants’ beliefs not only reflect but shape economic fundamentals. The man who broke the Bank of England argues that financial bubbles arise because forecasts influence prices, which alter the reality those forecasts tried to predict.
The point is that knowledge is recursive. A model generates signals and those signals influence modelling. Without a firm anchor to direct experience, the loop begins to tighten. First a little. Then a lot.
There are two ways that people generally describe model collapse in AI. The first, explained above, deals with training-time collapse. It’s about what happens when you repeatedly train a model on its own outputs (or the outputs of other models) over and over again.
The other, which is not actually an instance of model collapse, deals with inference-time feedback. A popular recent example of this idea can be seen in the picture at the beginning of this section. It shows an experiment in which a model is asked to recreate an image exactly as it appears. Seventy-five times later it bears no likeness to the original.
Some commentators reckon inference-time feedback spells doom for the AI project. They think that because these examples show that AI generates sloppy outputs when prompted with originals, the entire internet will be flooded with low quality training material, and subsequently the next generation of models is doomed.
But as we know, if each new model is trained on a combination of human-generated and synthetic data, collapse can be arrested as grounding puts a lid on the feedback loop. If AI-generated data wholly replace human data, the model becomes something like a funhouse hall of mirrors. But if we keep accumulating real text or images, outputs stay solid.
Both things going on here—the conflation of inference-time feedback and training-time collapse, and the weird ideas about how developers actually make models—point to the idea that talk of collapse is itself self-referential.
The claim becomes a kind of collapse in its own right. It’s recycled, repeated, and stripped of nuance with each iteration as it bubbles up the surface of your LinkedIn feed. People cite the same handful of examples, amplify each other's anxieties, and forget to check whether those fears still correspond to reality.
The Ptolemaic problem
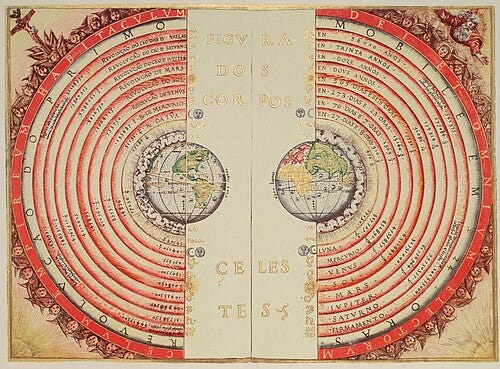
By 1613, Galileo had seen the new world and embraced its implications. Writing with dramatic flair, he scolded his opponents for clinging ‘with such obstinacy in maintaining Peripatetic conclusions that have been found to be manifestly false’.
Galileo’s practical experiments connected Copernicus’s theory to an underlying physical reality. Ptolemy’s cosmos was not a final scientific truth, but it was a necessary mythology to make sense of the cosmos.
This example and others tell us that model collapse is cultural pathology. It emerges when explanatory frameworks (be they cosmological, theological, artistic, or computational) become recursive without recognising their own closure. In each case, the signs are the same:
In ancient astronomy, the Ptolemaic system became so complex, with epicycles stacked on epicycles, that it eventually collapsed under the weight of its own internal logic.
In medieval theology, Scholasticism spun increasingly subtle distinctions in Aristotelian metaphysics, which reformers diagnosed as a recursive loop detached from experiential grounding.
In Renaissance art, Mannerism mimicked and magnified the styles of the High Renaissance. It turned the visual language of naturalism into stylised abstraction that some said was too far from the world.
In modern computing, model collapse is a fear born from a similar structure. It holds that AI models trained on AI outputs will spiral into self-reference, losing the diversity and grounding once present in human data.
Collapse is about forgetting what the model was originally for. A model breaks when its internal structure becomes so dominant that contradiction, novelty, or reality itself can no longer puncture it.
This is why model collapse is unlikely to pose trouble for the AI project. Developers know what the feedback loop for building resilient systems looks like. They are aware of the the pain points, the need for grounding mechanisms, and the most appropriate way to mix synthetic and real examples to avoid self-reinforcing drift.
Collapse is about a failure to distinguish between imitation and insight, reflection and source, and map and territory. It happens when we forget. When we forget to check our models against the world, when a system forgets its purpose, and when we forget to question our assumptions.