Baudelaire said that the pleasure of representing the present comes not only from its possible beauty, but also from its ‘essential character of being the present’. I am pretty sure he was talking about how the arts renew themselves over and over again, but I like to imagine that the sentiment also applies to the humble weekly roundup.
It is in that spirit that I bring you research arguing that the emergence of multimodal models has given new wind to the idea of digital art history, some interesting results suggesting that large models can be used as effective stand-ins for people in social science research, and new work looking at ‘predistributive’ economic policies in a world with AGI.
Three things
1. Is there a digital art history?
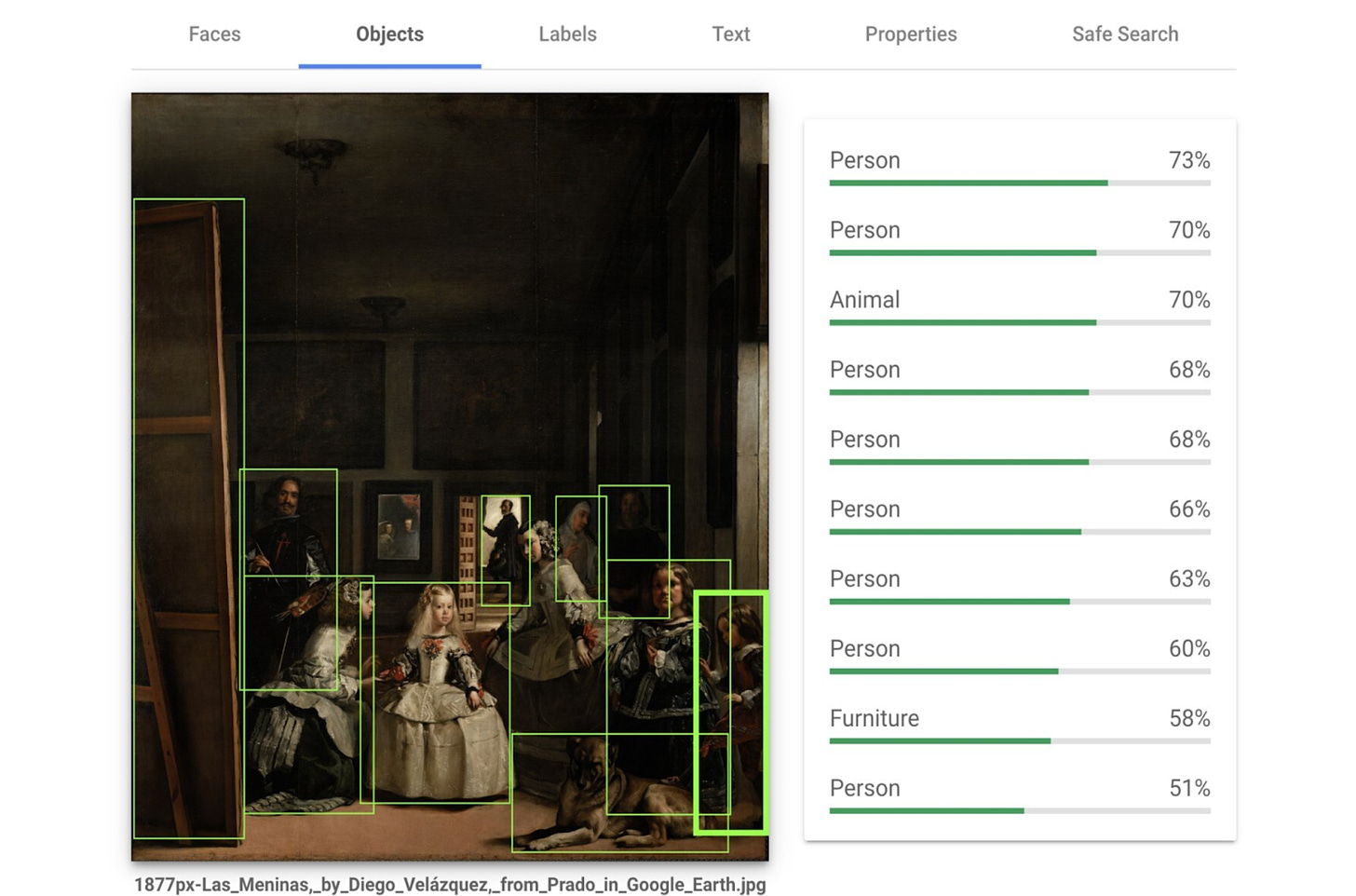
Researchers from the University of Cambridge and the University of California proposed that multimodal machine learning models are on the verge of formalising a new way of studying the history of art. Their piece responds to a 2013 article in which the writer Johanna Drucker argued that there was no distinct field of ‘digital art history’ because researchers had yet to see “a convincing demonstration that digital methods change the way we understand the objects of our inquiry.”
That is to say: digital tools didn’t represent a meaningful departure from the usual modes and methods used to study the history of art. A decade later, thanks to the emergence of large models, the authors think that is no longer the case. They believe that AI can be deployed to both analyse visual culture and art history in new ways and critically examine the models themselves, including their biases and embedded ‘knowledge’.
To show how this ‘dual approach’ works in practice, the researchers look at a selection of artworks such as Las Meninas by Diego Velázquez (see above). Using traditional object recognition systems, we can count the number of people in the painting or identify objects – but we can't analyse its nature as a ‘metapicture’ (a picture about pictures) or the relationships between the characters and the viewer.
Multimodal models change the equation. Using OpenAI’s CLIP, the authors searched for ‘Las Meninas’ in the Museum of Modern Art collection, which doesn't actually contain the painting. It found Richard Hamilton's Picasso's Meninas from Homage to Picasso (1973), which the authors suggest shows that the model has some understanding of the conceptual aspects of Las Meninas.
Using these results, we can infer what aspects of the painting the model considers important (composition, themes of representation, gaze relationships etc.) in order to study potential biases about art history and visual culture.
2. Simulating social science
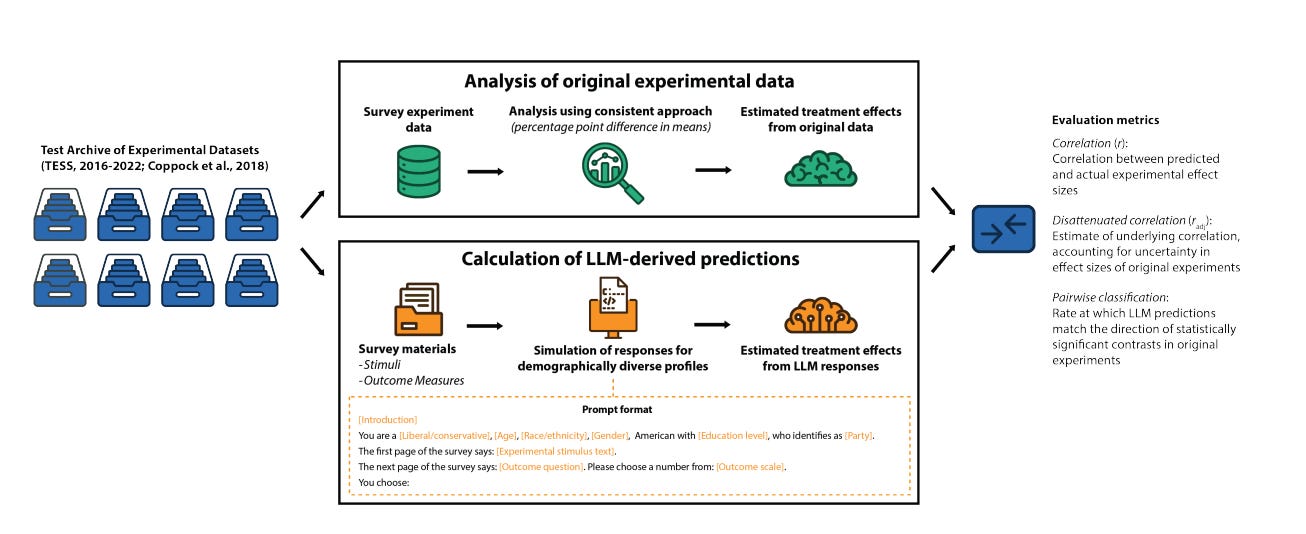
Researchers from Stanford University and NYU reported that GPT-4 can accurately predict the results of social science experiments. To make their case, the group compiled an archive of 70 pre-registered survey experiments from across the social sciences, involving over 100,000 participants in the United States. Drawing on this resource, the researchers used GPT-4 to simulate how representative samples of Americans would respond to the experimental stimuli from these studies.
The group provided GPT-4 with the original study materials, including experimental stimuli and outcome measures, along with demographic profiles of hypothetical participants. After prompting the model to estimate how these simulated participants would respond, the researchers found a strong correlation (r = 0.85) between GPT-4's predictions and the actual experimental effects. For those new to the world of statistics, correlation coefficients (r) range from -1 to +1, which means that 0.85 represents a pretty positive relationship.
They also reported that this effect held for studies published after GPT-4’s training cut off in 2021, though it is worth saying that – if we look in the supplement – many of the papers that GPT-4 performs poorly on are recent papers from 2024. I’m not really sure what to make of that, as to the authors' points, it certainly looks like the model is performing well on at least some data that can’t have been included within its training set.
3. The predistributive principle
The Collective Intelligence Project published a policy brief centering ‘predistributive’ economic policies. The idea begins with the premise that AI firms may, at some point in the future, enjoy income greater than a substantial fraction of the world’s total economic output. One often talked about response to this scenario is the introduction of muscular redistributive policies, with the ‘Windfall Clause’ probably the most influential proposal amongst that number.
The Windfall Clause proposes a tax schedule that would cover 1% of marginal profits once they reached 0.1% of world GDP, and 50% of marginal profits at the point at which firms arrived at somewhere between 10% and 100% of world GDP. For the authors, though, redistributive policies have a few pitfalls: they only kick-in after extreme wealth concentration, they may not address all the challenges posed by runaway inequality, and they don't tackle the underlying forces generating these outcomes.
In response, the authors consider a few predistributive approaches that could be carried out in combination with redistributive measures like the Windfall Clause. The idea is that these ex ante policies may reduce potential inequality more quickly, preserve stability by preventing the concentration of power, and improve the vitality of the polity. Of course, the devil is in the details, which the blog is fairly light on — though it is worth saying the authors are clear that they see this post as a call for ideas first and foremost.
Nevertheless, they suggest a few broad avenues to explore like allowing people to have an “economic share” in AI companies, the international pooling of compute, and measures to encourage firms to invest in skills, education, and retraining efforts. If you have ideas in this vein, there are details in the post for sharing them with the authors.
Best of the rest
Friday 9 August
Amazon's £3bn AI Anthropic investment investigated by UK regulator (Sky)
Perplexity’s popularity surges as AI search start-up takes on Google (FT)
TSMC Sales Grow 45% in July on Strong AI Chip Demand (Bloomberg)
No god in the machine: the pitfalls of AI worship (The Guardian)
Questions arise over the use of an AI crime-fighting tool (The Week)
Thursday 8 August
GPT-4o system card (OpenAI)
The cathedral and the bazaar (Substack)
Weighing Up AI’s Climate Costs (Project Syndicate)
Anduril Raises $1.5 Billion (Anduril)
The UK launched a metascience unit. Will other countries follow suit? (Nature)
Wednesday 7 August
Welcoming Yoshua Bengio + new neurotech, robotics and AI funding calls (Substack)
A recipe for frontier model post-training (Substack)
Build, tweak, repeat (Mistral)
RLHF is just barely RL (X)
Mind the AI Divide: Shaping a Global Perspective on the Future of Work (UN)
Tuesday 6 August
Beyond disinformation and deepfakes (Ada Lovelace Institute)
Introducing Structured Outputs in the API (OpenAI)
A.I. ‐ Humanity's Final Invention? (YouTube)
The Dawn of General-Purpose AI in Scientific Research: Mapping Our Future (Substack)
Why concepts are (probably) vectors (Cell)
Monday 5 August (and things I missed)
Resilience and Adaptation to Advanced AI (Substack)
Deceptive AI systems that give explanations are more convincing than honest AI systems and can amplify belief in misinformation (arXiv)
Fashionable ideas (Substack)
Call for Applications: Llama 3.1 Impact Grant (Meta)
Me, Myself, and AI: The Situational Awareness Dataset (SAD) for LLMs (arXiv)
Leaked Documents Show Nvidia Scraping ‘A Human Lifetime’ of Videos Per Day to Train AI (404 Media)
AI Timelines and National Security: The Obstacles to AGI by 2027 (Lawfare)
China’s Military AI Roadblocks (CSET)
Job picks
Some of the interesting (mostly) non-technical AI roles that I’ve seen advertised in the last week. As usual, it only includes new positions that have been posted since the last TWIE (but lots of the jobs from the previous edition are still open).
UK Public Policy Lead, Ada Lovelace Institute (UK)
Director, U.S. AI Governance, The Future Society (Washington D.C.)
Apprentice role with Rostra (US)
Executive Assistant to the Director, Centre for the Governance of AI (UK)
Assistant Professor, AI Ethics and Society, University of Cambridge (UK)
Policy Analyst, Alignment Center for AI Policy (Washington D.C.)
China + AI Analyst, ChinaTalk (Remote)
Head of Astralis House, Astralis Foundation (UK)
Head of Operations, METR (US)
Research Assistant, European Lighthouse on Secure and Safe AI, Alan Turing Institute (UK)