Today’s post is the first entry in a new series looking at important moments in the history of AI and the fields that influenced it. These pieces will be shorter than the weekly essays and will land in your inbox every Friday.
This week also marks the start of reader pledges. I’m testing the waters to see whether there’s a path towards working on this newsletter full time, with a goal of 100 pledges by May 31. The response has been even better than I had hoped, so thank you to everyone who has kindly pledged so far. For anyone considering supporting this project, this is the best moment to help it grow.
Ramón y Cajal loved to sketch.
As a boy, he was gripped by a self-described ‘irresistible mania’ to draw. Whenever he saw a white wall, Cajal had no choice but to scribble on it — much to the chagrin of his parents who considered young Ramon’s interests in the arts to be something of an inconvenience.
The Spaniard used to cobble together enough pennies to buy art supplies and sneak out of the house. Sitting on a bank at the side of the road, he drew carts, horses, villagers, and anything else that took his fancy.
Born in 1852 in Petilla de Aragón in northeastern Spain, he grew up in a country still grappling with the aftershock of the Napoleonic Wars that dominated the opening decades of the century.
Napoleon famously called the Iberian campaign the ‘Spanish ulcer’. It was a wound that festered long after the French withdrawal, one that only showed signs of healing in the wake of the ‘Glorious Revolution’ of 1868 which ended the tumultuous reign of Queen Isabel II.
Spanish science picked up just as Cajal began to get a taste for medical practice thanks to his father. The senior Ramón was a barber-surgeon. It was a profession that, since medieval times, tended to be the natural choice for minor surgeries and bloodletting.
Over his father’s shoulder, Cajal learned anatomy through dissecting and drawing cadavers. It was a grisly experience, but one that put him on the path to a medical career.
Following a stint as an army doctor that included a tour during Cuba’s first war of independence, the surgeon returned to Spain in 1877 to pick up a doctorate from the Complutense University of Madrid.
After a decade that included a bout with a nasty strain of tuberculosis and entry into the Caballeros de la Noche Masonic lodge, Cajal moved to the Faculty of Medicine at the University of Barcelona.
In Catalonia, the Spaniard first saw early plates of neurons treated with colouring compounds that looked like ‘Chinese ink on transparent Japanese paper.’ What had been an ‘inextricable network’ when stained with carmine and hematoxylin became ‘simple, clear, and unconfused.’
Gripped by what he saw, Cajal did what he liked most of all: he drew.
In May 1888 he used the staining process and his sketch pad to argue that brain tissue was not composed of continuous connections. This idea, which rejected the view favoured by Italian physician Camillo Golgi, relied on Cajal’s ability to observe discrete neurons rather than nerves and brain tissue.
When Golgi used the cell staining technique, he interpreted the black-reaction silhouettes as evidence that neuronal processes fuse into a single protoplasmic web—a ‘reticulum’ of continuity—rather than remaining distinct cells that happen to touch. But Cajal, who tweaked the recipe to use younger brain tissue, noticed tiny breaks between the cells.
The disagreement was bad tempered. When both Cajal and Golgi received a joint Nobel Prize in 1906, Golgi used the spectacle of the moment to drum up support for his position.
History sided with Cajal. Later confirmed by electron microscopy, the neuron doctrine eventually became the foundation of modern neuroscience.
The neuron doctrine in AI
AI is not neuroscience, and artificial neural networks are not brains. But that doesn’t mean that, throughout history, AI researchers haven’t looked to neuroscience for inspiration to make sense of their creations.
Cajal’s work allowed Nicolas Rashevsky, a Russian-American biologist who was instrumental in creating the field of mathematical biology, to establish a mathematical foundation for understanding neurons.
Throughout the 1930s, he developed equations to articulate the processes by which neurons interact with each other at the University of Chicago, describing the functioning of nerve cells in the language of mathematics.
Using mathematical terms was a watershed moment: if real neurons could be described through equations, it followed that versions of their biological processes might be represented in artificial structures.
That moment came in 1943 with the introduction of the McCulloch-Pitts neuron, which the pair developed by directly building on Rashevsky’s work. In their blockbuster paper, ‘A Logical Calculus of the Ideas Immanent in Nervous Activity,’ Warren McCulloch and Walter Pitts (a student of Rashevsky) created a mathematical model that acted as a simplified abstraction of a biological neuron.
These ideas eventually led to the development of Frank Rosenblatt’s famous perceptron algorithm, the rise of parallel distributed processing techniques in the 1980s, and ultimately the giant neural nets that make ChatGPT, Claude, and Gemini tick.
But Cajal’s influence on the development of artificial neural networks wasn’t only indirect. Even in the 1980s, prominent research groups were publishing AI papers complete with Cajal’s drawings. Writing in 1986, researchers from Bell Labs explained that:
‘circuit considerations will probably limit digital computer cycle times to a few tenths of a nanosecond, speeds that are within an order of magnitude of today's fastest machines. The way to further increase computing power is through parallel computation.’
To make their case, the authors reproduced a rendering of a neuron drawn by Cajal. They reasoned that neurons receive input signals from other neurons through their dendrites, sum those signals together (weighing each based on the strength of its synaptic connections), and fire an output signal whose strength is determined by these input signals.
Such outputs cause the neuron's firing rate to increase proportionally for moderate input sums but saturate at maximum or minimum levels for extremely high or extremely low sums. When bundled into very large networks, the researchers argued, it is this computational structure that provides the complex information processing capabilities of the brain.
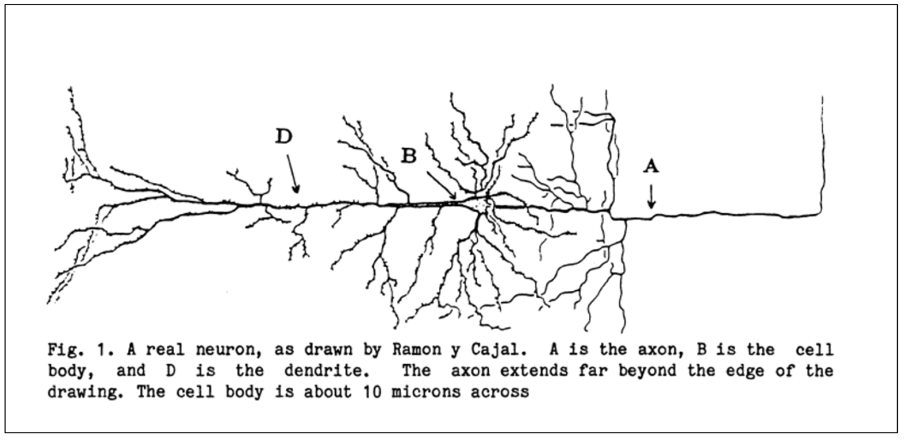
The weight of evidence eventually proved Cajal right, but he also told the best story by painting the best picture. The ink lines in his notebooks were persuading colleagues long before electron beams could.
Cajal reminds us that discovery is not just a matter of seeing but of showing. All things being equal, the scientist who can make plain truths pretty tends to be the one that wins.