Learning From Examples passed 1,000 subscribers this week. For that small milestone, I want to thank everyone who reads, emails, and comments — especially all of you whose feedback helped shape what I write and how I write it. I haven’t been able to share as many essays as I’d have liked in the last few months, but I’m hopeful that will change in the not too distant future.
As for today’s order of service, we have reports on AI and income inequality in the US, the impact of AI-based recommenders on human behaviours, and a taxonomy of bias in AI images (plus the customary links and jobs). Now and always, write to me at hp464@cam.ac.uk if with comments, questions, feedback, or anything else!
Three things
1. Forecasting inequality
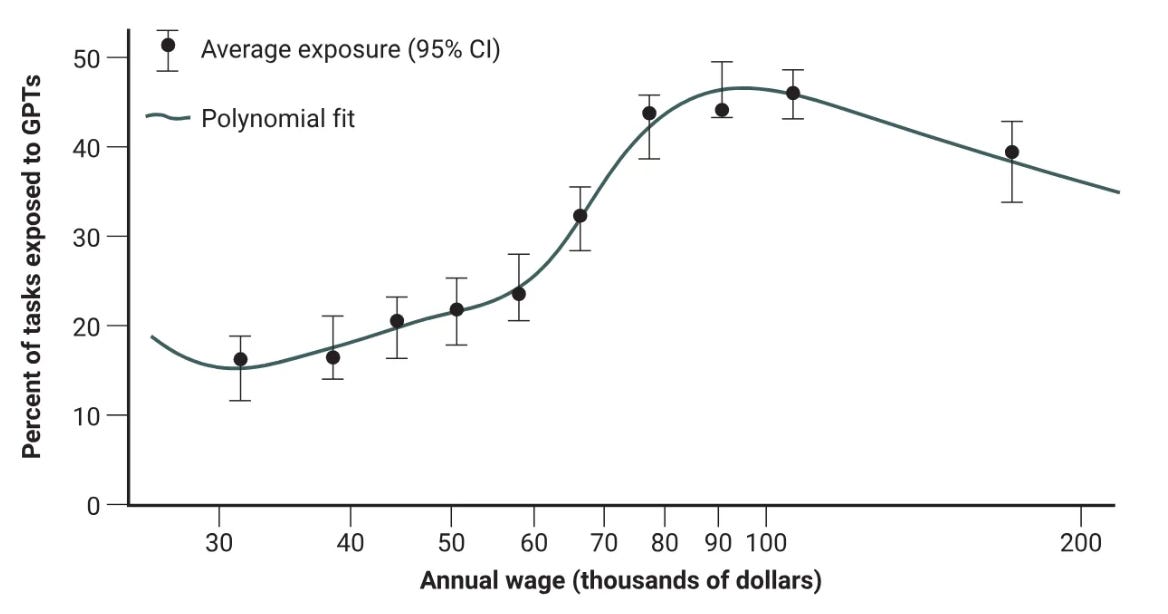
Sam Manning wrote on AI’s impact on US income inequality for Brookings, arguing that AI may exacerbate income inequality through two main mechanisms. In the near term, the argument goes, AI-driven productivity gains are likely to be concentrated among higher-income knowledge workers who are better prepared to integrate AI tools into existing workflows. Lower-wage workers in physical or service jobs have less direct access to leverage these systems, which may potentially widen wage gaps by insulating them from productivity enhancing effects.
Over the long term, though, the piece reckons there's potential for increased automation that shifts economic returns from labour to capital. While early evidence shows larger productivity gains for novice workers within certain high-skill occupations, this dynamic could lead to job displacement as AI systems get better. The concern here is that firms may reduce workforces if consumer demand doesn't match productivity increases (a similar dynamic to that seen in reports from Klarna, which laid off about 700 staff after integrating AI).
No one really knows how the development of AI will play out on the labour market, but I agree with the basic assessment that existing systems have a very different risk profile to those that may be developed in the future. If AI agents can comprehensively substitute for remote workers, physical jobs may benefit from the fallout; if they can’t, then it's the remote workers who may gain and physical workers that could lose out.
2. Recommender systems are everywhere
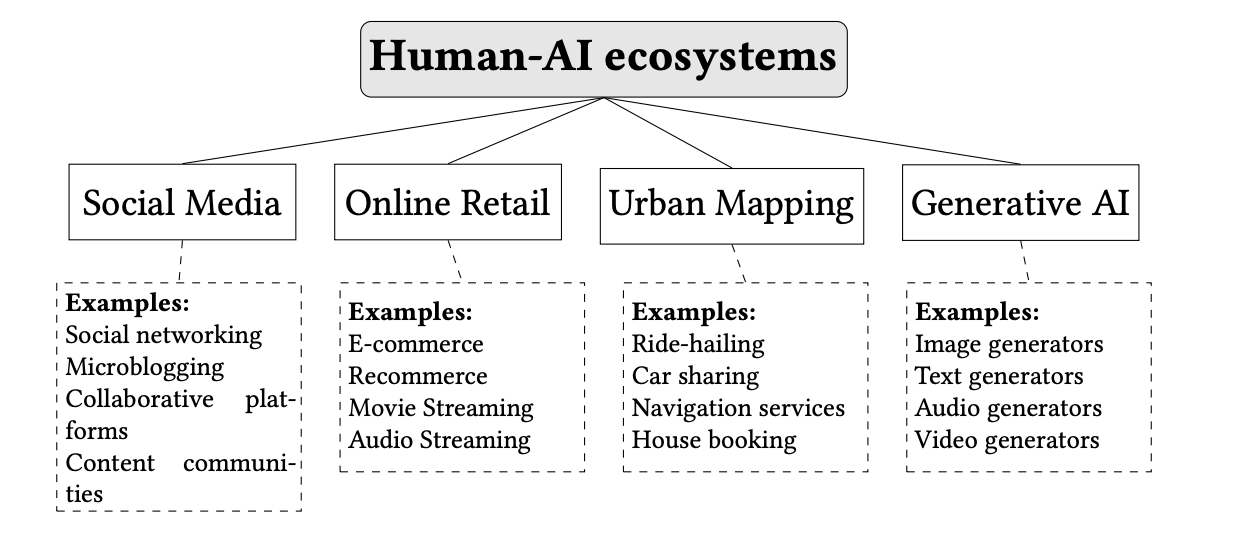
New work from French and Italian academics looks at the impact of AI-based recommenders across four key ‘ecosystems’: social media (recommending posts), online retail (recommending goods), urban mapping (recommending travel routes), and generative AI (when a user asks a content generation system for recommendations).
The survey found that in social media, ’polarisation’ (instances whereby different groups organise around niche interests) emerges consistently, but the impacts on ‘radicalisation’ seem to be harder to determine. Online retail studies generally show that recommenders increase diversity at the individual level but reduce it systemically i.e. that individual users tend to explore a fairly broad range of products, but overall, the system converges towards a more homogeneous set of popular items.
Meanwhile, the authors found that urban mapping research focuses on congestion, volume, and inequality (with mixed findings on traffic and emissions impacts), while studies have found that generative AI—when used as a recommender—tends to narrow rather than expand the diversity of content it produces or suggests.
My view is this work draws out two important questions. First, whether recommender systems have the potential to significantly shape tastes rather than primarily reflect user preferences. Second, if they can effectively shape tastes at scale, whether the widespread use of AI will provoke consolidation around a handful of interests. If the answer to both questions is ‘yes’ then we are probably in for both a more boring and more polarised future (so long as countermeasures are not baked into systems to prevent this type of amalgamation).
3. A taxonomy of bias in AI images
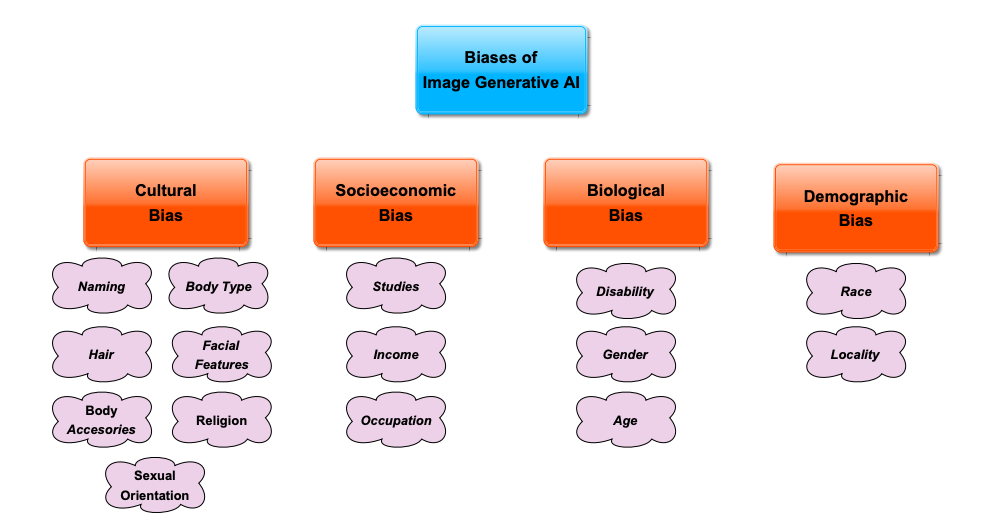
Researchers from Universidad Pontificia Comillas in Madrid released a taxonomy of biases of images created by generative AI by categorising them into cultural, socioeconomic, biological, and demographic groups. The study identifies specific biases within each category, such as naming bias, body type bias, income bias, disability bias, and racial bias, amongst plenty of others.
I like that the paper gets to grips with the underlying reasons behind these biases, describing AI image generation models as conditional probability distributions trained on potentially biassed datasets. The authors also suggest methods for mitigating bias, including data augmentation to increase representation of underrepresented groups and the use of ‘regularisers’ in the training algorithm to penalise biassed values (i.e. adding a penalty term to the model's objective function that discourages biassed outputs).
Of course, all technology is biassed in one form or another in that it represents the views, beliefs, and perspectives of its developers. While recognising potential issues is important for determining how best to respond, the real challenge is implementing a correction that doesn’t stymie personalisation or oversteer in a manner that inadvertently introduces new problems.
Best of the rest
Friday 5 July
AI has all the answers. Even the wrong ones (FT)
Baidu Upgrades Ernie AI Model, Cuts Pricing Further (Bloomberg)
Nvidia to make $12bn from AI chips in China this year despite US controls (FT)
Finally: Someone used generative AI in a Western election (Politco)
Samsung expects profits to soar with boost from AI (BBC)
Thursday 4 July
A Hacker Stole OpenAI Secrets, Raising Fears That China Could, Too (New York Times)
ChatGPT just (accidentally) shared all of its secret rules – here's what we learned (techradar)
Mind-reading AI recreates what you're looking at with amazing accuracy (New Scientist)
Ray Kurzweil Still Says He Will Merge With A.I. (New York Times)
Why AI may fail to unlock the productivity puzzle (Reuters)
Wednesday 3 July
Patent Landscape Report: Generative Artificial Intelligence (WIPO)
Are Large Language Models Consistent over Value-laden Questions? (arXiv)
Meta ordered to stop training its AI on Brazilian personal data (The Verge)
Our attitudes towards AI reveal how we really feel about human intelligence (The Guardian)
Nvidia, Microsoft, Meta Warn Investors AI is a Risky Financial Bet (Bloomberg)
Tuesday 2 July
AI in Action: Accelerating Progress Towards the Sustainable Development Goals (arXiv)
Apple Poised to Get OpenAI Board Observer Role as Part of AI Pact (Bloomberg)
A False Sense of Safety: Unsafe Information Leakage in 'Safe' AI Responses (arXiv)
We Need to Control AI Agents Now (The Atlantic)
Revolutionising Role-Playing Games with ChatGPT (arXiv)
What happened to the artificial-intelligence revolution? (The Economist)
This Week in AI: With Chevron’s demise, AI regulation seems dead in the water (TechCrunch)
How Manufacturers Can Use AI to Help Manage the Workforce Shortage (The National Law Review)
Monday 1 July
Covert Malicious Finetuning: Challenges in Safeguarding LLM Adaptation (arXiv)
AI’s $600B Question (Sequoia)
AI Agents That Matter (arXiv)
A new initiative for developing third-party model evaluations (Anthropic)
When RAND Made Magic in Santa Monica (Asterisk)
Job picks
Some of the interesting (mostly) non-technical AI roles that I’ve seen advertised in the last week. As usual, it only includes new positions that have been posted since the last TWIE (but lots of the jobs from the previous edition are still open).
Various jobs at LawAI (Remote)
Partnerships Lead, ARIA (London)
AI policy researcher (contractor), CLTR (London/Remote)
Technical Advisor, Artificial Intelligence, Public Policy, EY (Belgium)
Head of Policy, Tech Governance Project (Remote)