I’m back after a short break in Scotland with another The Week In Examples (and with thanks to everyone who got in touch after last week’s stand-in piece on the IAEA). Before we begin, a bit of shameless self promotion: I was interviewed by MIT Tech Review for an article on the history of AI. It’s a really great read, so I’d encourage you all to check it out should you have a few minutes to spare. As usual, it’s hp464@cam.ac.uk for comments, questions, feedback, or anything else.
Three things
1. Questionable practices in machine learning
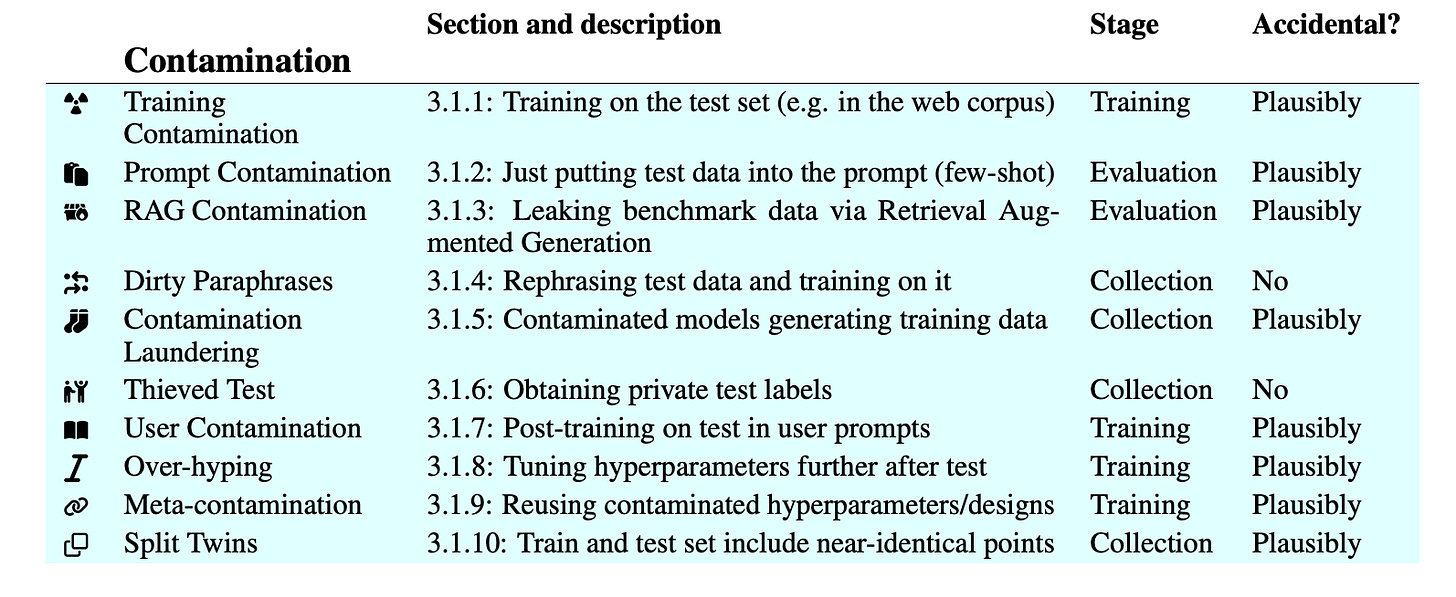
Researchers from the University of Bath, University of Bristol, and Arb Research weighed in on AI’s topic du jour: the overwhelming challenges posed by the evaluation of large language models. In a new paper, the group lists 43 ways machine learning evaluations can be “misleading or actively deceptive.” Taking cues from psychological science, they call these instances “questionable research practices”, which they group into three categories.
First, contamination, wherein information can leak from one part of the model training process to another. The most well-known example of this phenomenon is training contamination, which sees data from the training set (the set of examples a model learns in pre-training) migrate to the test set (a new set of examples that it shouldn’t have seen before used to assess performance).
Second, cherrypicking, which involves choosing amongst runs to make a system look better than it actually is. In practice, this sees researchers ‘hack’ experiments by selecting those under which their model works better than others after testing multiple times. This group also includes things like prompt hacking (i.e. choosing the best prompt strategy like implementing chain of thought approaches that work better for some models than others) and benchmark hacking (i.e. picking the easiest benchmarks for a particular model).
Third and finally they cover misreporting, which boils down to researchers indulging in misleading calculations or presentations. This bucket contains things like under-reporting the size of a particular model, and failing to report negative benchmark studies.
All in all, a great explanation of the ways standards and measurements can be manipulated or, more charitably, misconstructed by AI researchers. This sort of stuff tends to happen either a) by accident; or b) to make sure new work is seen to keep pace with that of others, though I should say this dynamic is not exactly limited to AI. If you’re interested in how measurement standards come to be, I’d recommend reading Proxies: The Cultural Work of Standing In by Dylan Mulvin, Beyond Measure: The Hidden History of Measurement by James Vincent, and Objectivity by Lorraine Daston and Peter Galison.
2. The State of Chinese AI
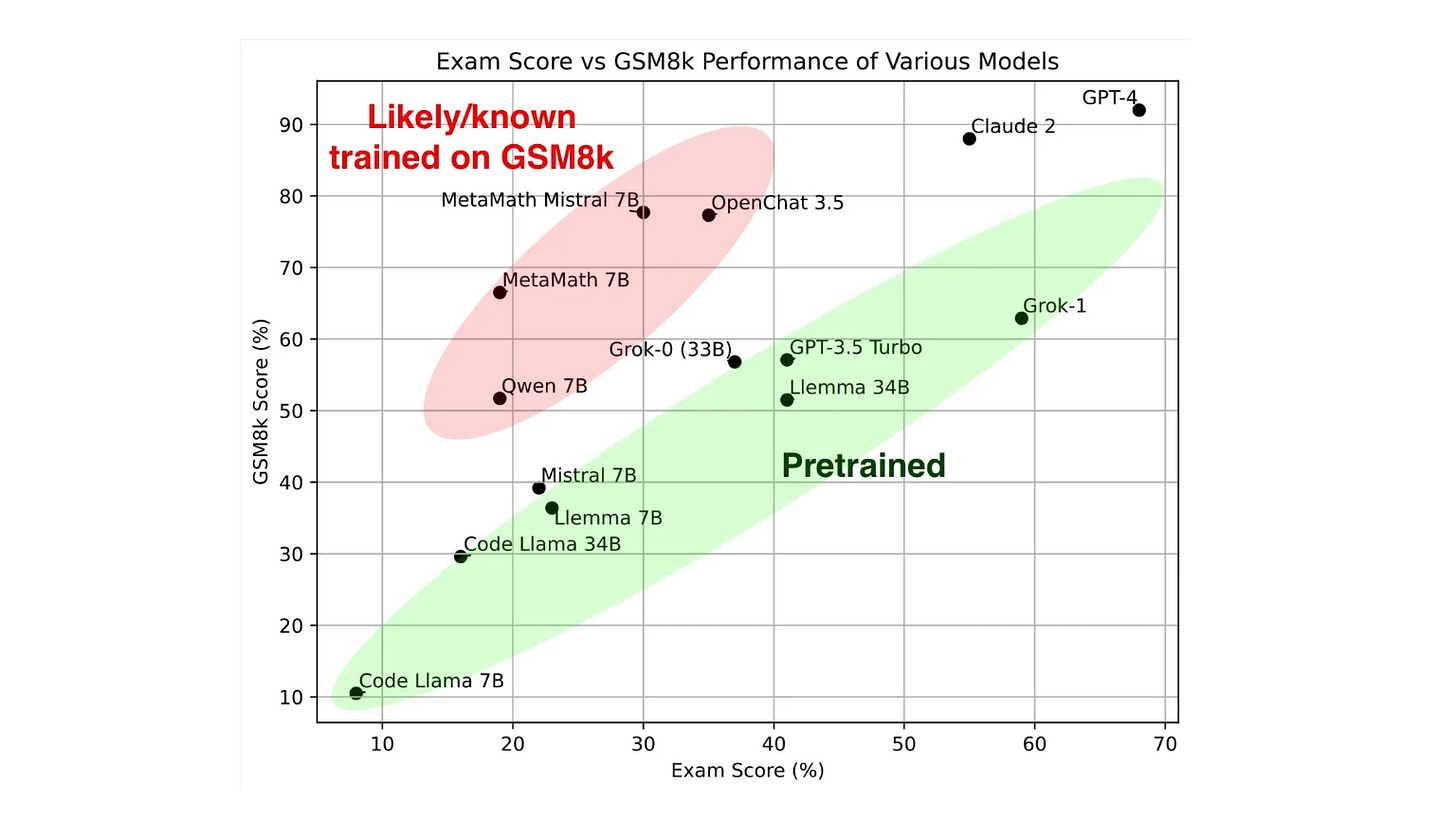
The folks at Air Street Capital, an early stage VC investing in what I can only describe as stuff that matters (i.e. not your usual slew of B2B SaaS firms) published a review of China’s AI landscape. The work introduces a few interesting elements worth reflecting on, including a sober take on the strengths and weaknesses of Chinese AI, the impact of sanctions on development, and the loopholes associated with their effective implementation.
One particularly interesting point, though, takes aim at the idea that China’s AI firms are wholly reliant on the Llama architecture of Meta’s Llama series of models. They argue that, because Llama isn’t really much of a departure from the transformer architecture used by pretty much every single large model, access to Llama “hasn’t given Chinese labs some kind of secret recipe that they would have been unable to obtain themselves through other work.”
The work comes as Liang Wenfeng, the founder of the impressive Chinese AI startup DeepSeek, gave a no holds barred interview explaining the reason the company attempted a (relative) departure from the Llama approach with its new multi-head latent attention mechanism. As Wenfeng explains: “If the goal is to develop applications, then it is a reasonable choice to continue using the Llama structure and quickly launch products. But our destination is AGI, which means we need to study new model structures to achieve stronger model capabilities under limited resources.”
3. Meta says no to multimodal models in the EU
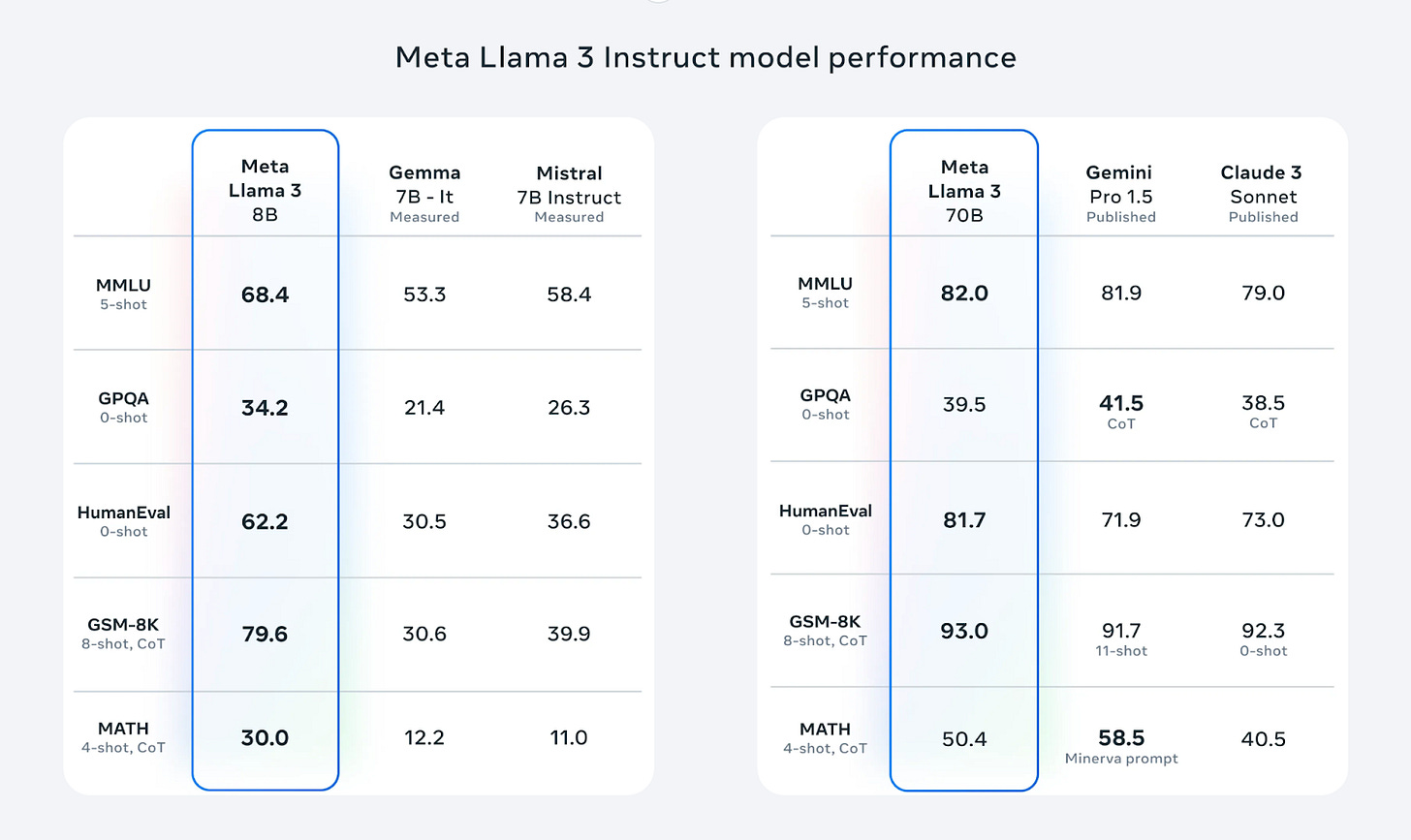
Axios reported that Meta is no longer planning to release multimodal AI models in the EU, which follows a similar move from Apple. I’ve heard conflicting reports about whether this statement applies to the upcoming Llama 3 405bn model, but at the very least it should apply to the next generation of the company’s large language models.
The reason for the decision, according to the report, is “due to the unpredictable nature of the European regulatory environment." While you might think the company’s rationale is connected to the recently formalised AI Act, Axios reckons the real reason is down to issues with training models using data from European customers while complying with GDPR.
Of course, as described above, Meta tends to share the weights of its models, meaning anyone can download and store them for use in pretty much any capacity they like. While it’s not all that clear whether it will continue to do so in the future (though Mark Zuckerberg recently said it would), I struggle to see how someone in Europe wouldn’t be able to access the model once it was released in the United States, UK, and elsewhere.
It is possible that this comment is primarily about providing access via API or baking the systems into consumer applications, but even given tight restrictions across these access vectors it would still be trivially easy for someone in Europe to use the model if they really wanted to (again, assuming the weights are released elsewhere).
Best of the rest
Friday 19 July
Transforming risk governance at frontier AI companies (CLTR)
OpenAI and Broadcom in talks about developing new AI chip (FT)
AI is overpowering efforts to catch child predators, experts warn (The Guardian)
Why we need a unified framework for policing generative AI (WEF)
Censorship slows China's AI advances (Axios)
Here’s the real reason AI companies are slimming down their models (Fast Company)
Thursday 18 July
AI in the public sector: white heat or hot air? (Ada Lovelace Institute)
Enhancing Biomedical Knowledge Discovery for Diseases: An End-To-End Open-Source Framework (arXiv)
GPT-4o mini: advancing cost-efficient intelligence (OpenAI)
Bye-bye bitcoin, hello AI: Texas miners leave crypto for next new wave (CNBC)
Many people think AI is already sentient - and that's a big problem (New Scientist)
Portal needed for victims to report AI deepfakes, federal police union says (The Guardian)
Wednesday 17 July
SB 1047, AI regulation, and unlikely allies for open models (Substack)
Towards Understanding Unsafe Video Generation (arXiv)
Prover-Verifier Games improve legibility of language model outputs (OpenAI)
Hospitals will use AI to speed up patient care (BBC)
Island of riches: Taiwan reaps benefits of AI boom (FT)
Meta's use of user data to train its AI violates GDPR, privacy group says (The Standard)
Trump allies draft AI order to launch ‘Manhattan Projects’ for defense (Washington Post)
Tuesday 16 July
Does Refusal Training in LLMs Generalize to the Past Tense? (arXiv)
LAB-Bench: Measuring Capabilities of Language Models for Biology Research (FutureHouse)
Microsoft deal with AI startup to be investigated by UK competition watchdog (The Guardian)
GPT-4V Cannot Generate Radiology Reports Yet (arXiv)
Artificial intelligence and copyright: use of generative AI tools to develop new content (EU)
J.D. Vance’s A.I. Agenda: Reduce Regulation (The New York Times)
China deploys censors to create socialist AI (Financial Times)
Generative AI is speeding procurement, but not without risks (StateScoop)
Monday 15 July
Wet-lab innovations will lead the AI revolution in biology (Substack)
OpenAI nears "reasoning"-capable AI (Axios)
AI’s ‘Oppenheimer moment’: autonomous weapons enter the battlefield (The Guardian)
On Open-Weights Foundation Models (FTC)
The rewards of AI are huge, but so are the risks — enter AI insurance (The Hill)
Job picks
Before getting into the usual jobs I’d like to draw your attention to the bucket load of cool roles going at the Institute for Law and AI, including for a research fellow, programme associate, and a chief of staff. I know the team there well and can vouch for the incredibly talented people working on some of the most interesting AI issues around. Go and apply here!
Research Assistant / Associate, AI Ethics and Decision-Making in Connected Places, University of Cambridge (UK)
Senior Director, Responsible AI Testing, Evaluation, and Alignment, Salesforce (US)
Senior Researcher, Artificial Intelligence, Security and Technology Programme, UN (Geneva)
Product Policy Manager, Bio, Chem, and Nuclear Risks, Anthropic (US)
Winter Fellowship 2025, GovAI (UK)