After no edition last week, I’m back with the usual roundup of things that say something interesting about AI and society. As always, if you want to send anything my way, you can do that by emailing me at hp464@cam.ac.uk.
Three things
1. Please don’t think step by step
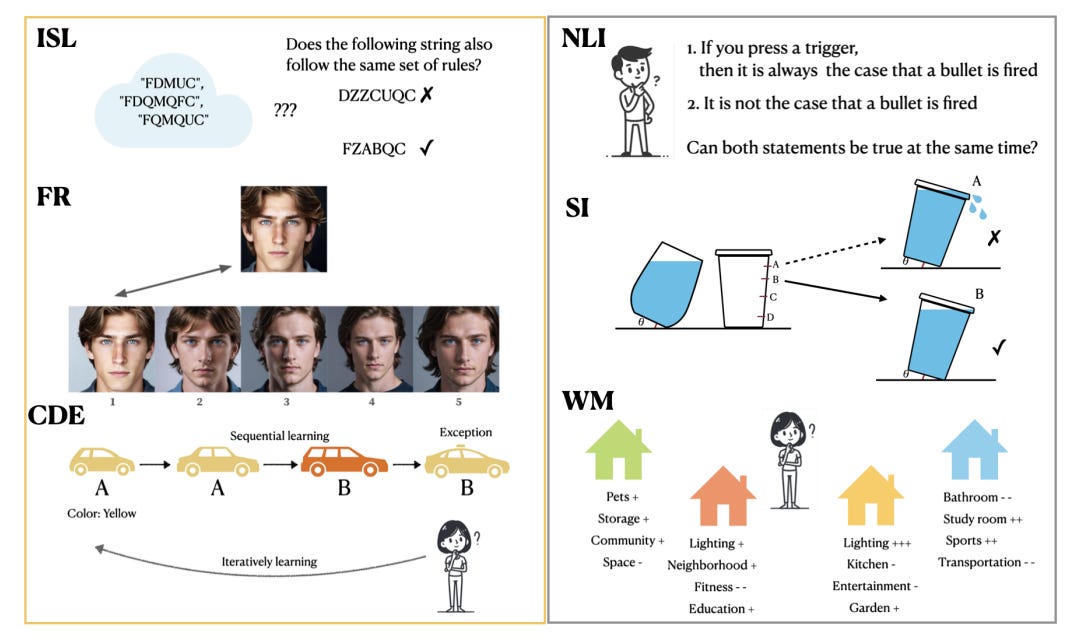
One of the curious things about large language models is they are extremely sensitive to prompting. Amongst lots of different tips and tricks for eking out superior results is to ask a model to ‘think step by step’ when providing you with its answer. There are a whole bunch of versions of this method, with some more sophisticated than others, but they can all be adequately described as ‘chain of thought’ approaches.
But as it turns out, thinking step by step doesn't always guarantee better results. More interesting still is that large models perform worse in the same settings that humans do when ‘over-thinking’. Consider the psychological phenomenon ‘verbal overshadowing’ first demonstrated in a 1990 study, which found that when humans verbally describe a face's features it actually impairs their ability to later recognise that same face.
A new study finds that AI models showed a similar pattern: when forced to verbally reason about facial recognition through chain-of-thought prompting, their performance dropped significantly compared to when they simply made direct visual comparisons. The upshot is that, like humans, having AI models try to verbalise their visual recognition process can degrade their performance rather than help it. What funny old machines they are.
2. Claude at the wheel
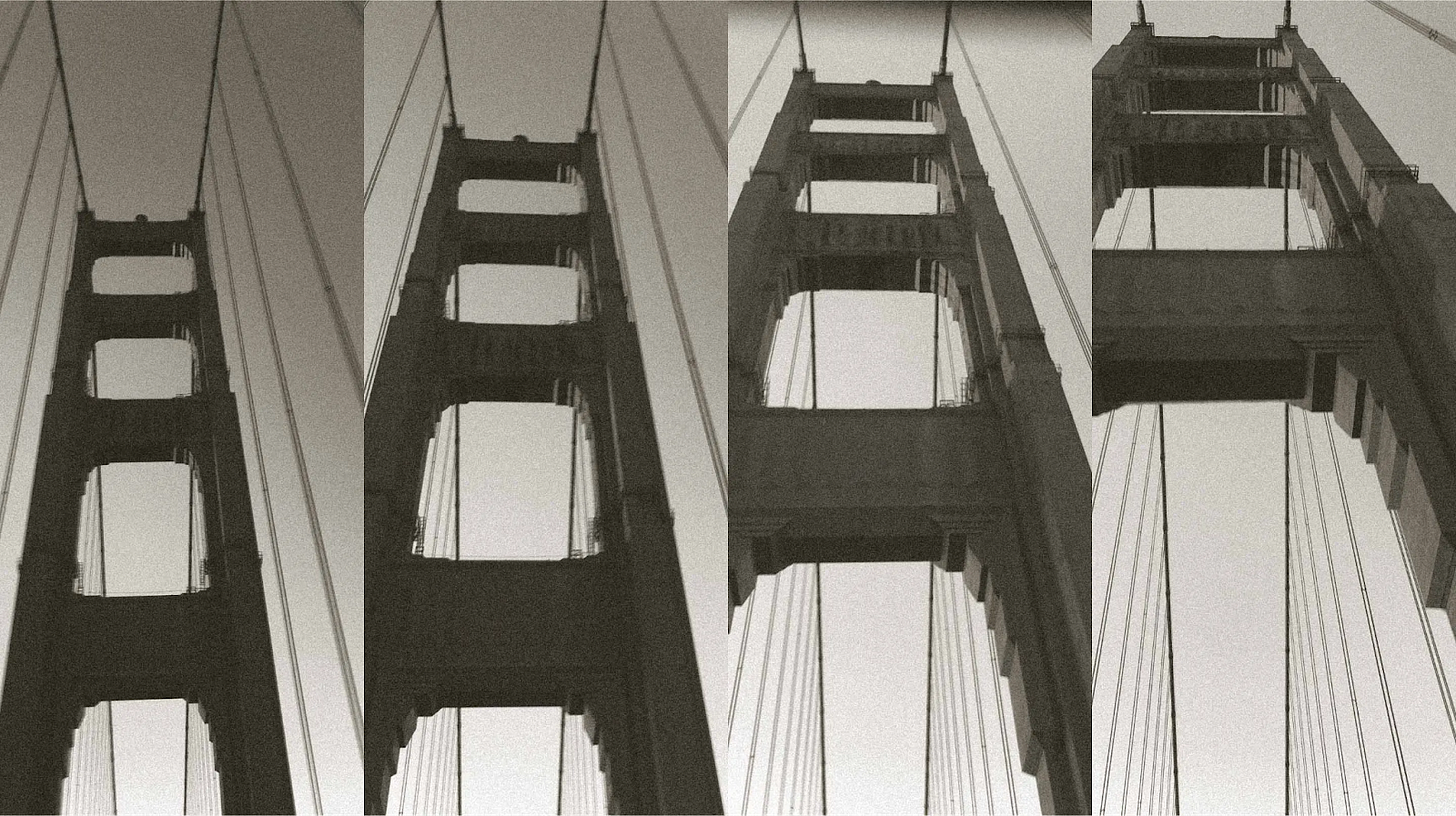
Anthropic released a follow-up to the mighty ‘Golden Gate Bridge’ Claude, which demoed the company’s approach to a promising technique for making models do what we want. As a reminder, in May earlier this year, Anthropic published a version of its Claude chatbot that wouldn’t stop talking about the Golden Gate Bridge. They did that by finding a specific combination of neurons in Claude’s neural network that activate when the model encounters a mention (or a picture) of San Francisco’s most famous landmark.
Users got to talk to Golden Gate Bridge Claude for a 24hr period before the research preview went offline. Lots of jokes were made and we all had a lot of fun. But questions, like whether this approach would actually be useful for safety work, remained. Now, it looks like we have an answer – sort of. In new work on this technique, referred to as ‘feature steering’, Anthropic found that “within a certain range (the feature steering sweet spot) one can successfully steer the model without damaging other model capabilities.”
Like so many deep learning techniques, though, feature steering is powerful but brittle. As they go on to explain, “past a certain point, feature steering the model may come at the cost of decreasing model capabilities—sometimes to the point of the model becoming unusable.” That means for feature steering to be a useful technique for aligning large language models, researchers will need to figure out a way to implement it that doesn’t dampen performance. My guess would be that is probably possible – but we’re not there yet.
3. Building AI in America
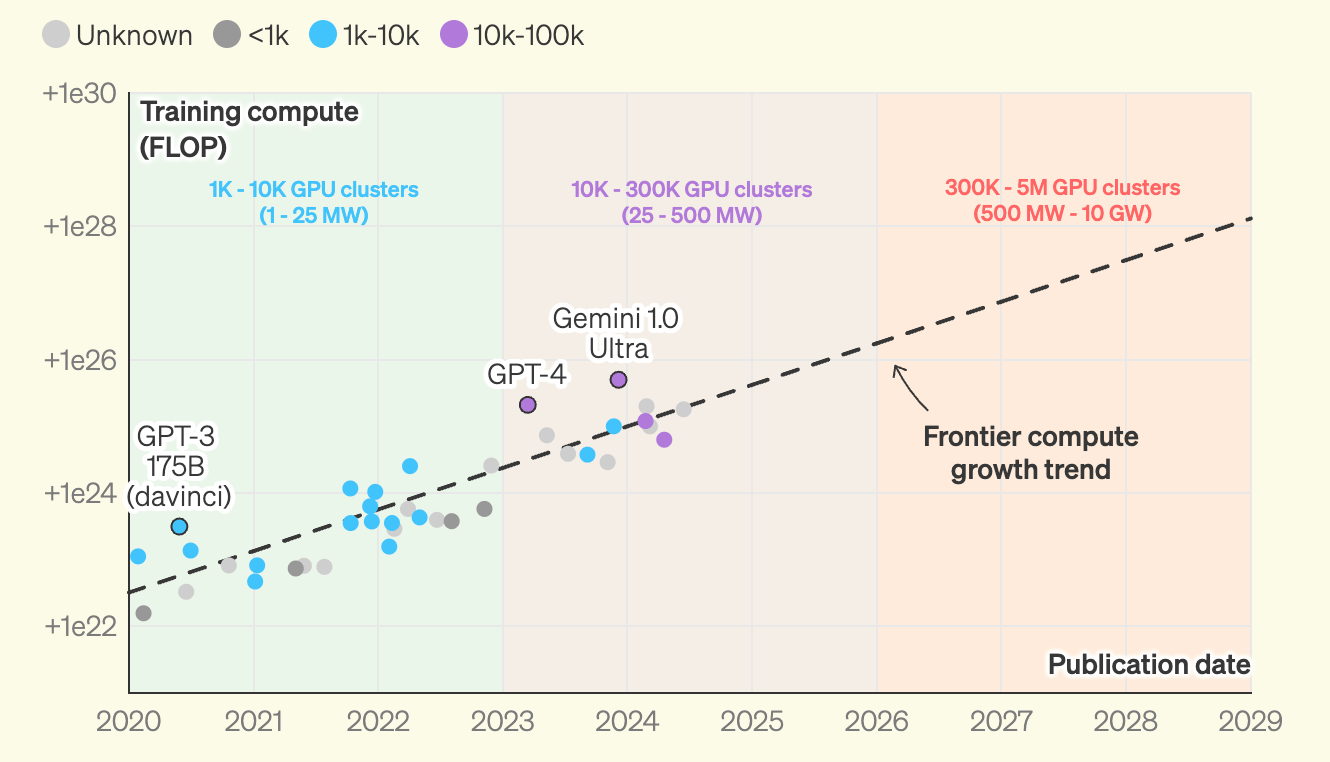
Last week, the fine folks at the Institute for Progress published a blog about what it will take to build the AI models of the future in the United States. The work begins with the elephant in the room: models are getting a lot bigger, and bigger models need more energy to train. By 2029, some commentators reckon that the biggest models will require 10 GW of power before accounting for inference (actually using AI). That’s just, you know, over ten times the power generated by the Three Mile Nuclear plant soon to be reopened by Microsoft to feed the beast.
The authors reckon that “globally, the power required by AI data centres could grow by more than 130 GW by 2030” while American power generation is only forecasted to grow by 30 GW during the period. As a result, firms are looking abroad to fulfil these power requirements, with the likes of Brazil and the UAE emerging as beneficiaries of the trend.
To build the large models of the future in the US, the article argues, the most viable path forward is through ‘behind-the-metre’ power generation, which involves building power sources directly at data centre sites rather than connecting to the existing power grid. The idea is that this approach avoids the lengthy delays (often 10+ years) associated with permitting new transmission lines and connecting to the grid. In this scenario, companies can build new power generation sites––like Google’s recently signed deal to purchase nuclear energy from small modular reactors––rather than waiting on public power consumption initiatives to catch up.
Best of the rest
Friday 1 November
Microsoft Picks Up the Pace of Spending But AI Demands More (Bloomberg)
Exclusive: Chinese researchers develop AI model for military use on back of Meta's Llama (Reuters)
Introducing ChatGPT search (OpenAI)
AI drones flying without pilots on special operations launched by Ukraine to hit Russian targets (The Standard)
Thursday 31 October
‘3cb’: The Catastrophic Cyber Capabilities Benchmark (Arc Research)
New rules? Lessons for AI regulation from the governance of other high-tech sectors (Ada Lovelace Institute)
AI becomes Microsoft’s fastest-growing business (Computer Weekly)
Creators demand EU 'meaningfully implement' AI Act (Euronews)
Ukraine rolls out dozens of AI systems to help its drones hit targets (Reuters)
Wednesday 30 October
Chinese AISI Counterparts (IAPS)
Global Catastrophic Risk Assessment (RAND)
LinkedIn launches its first AI agent to take on the role of job recruiters (TechCrunch)
Introducing SimpleQA (OpenAI)
Microsoft’s GitHub Unit Cuts AI Deals With Google, Anthropic (Bloomberg)
Implications of Artificial General Intelligence on National and International Security (Yoshua Bengio)
Tuesday 29 October
Do Large Language Models Align with Core Mental Health Counseling Competencies? (arXiv)
AI images, child sexual abuse and a ‘first prosecution of its kind’ - podcast (The Guardian)
FNDEX: Fake News and Doxxing Detection with Explainable AI (arXiv)
Ethical Statistical Practice and Ethical AI (arXiv)
Google parent Alphabet sees double-digit growth as AI bets boost cloud business (The Guardian)
Monday 28 October (and things I missed)
Mind Your Step (by Step): Chain-of-Thought can Reduce Performance on Tasks where Thinking Makes Humans Worse (arXiv)
Free LLM courses from Anthropic (GitHub)
Safety cases for frontier AI (arXiv)
How AI Can Automate AI Research and Development (RAND)
VibeCheck: Discover and Quantify Qualitative Differences in Large Language Models (arXiv)
Job picks
Some of the interesting (mostly) AI governance roles that I’ve seen advertised in the last week. As usual, it only includes new positions that have been posted since the last TWIE (but lots of the jobs from the previous edition are still open).
EU Policy Researcher, Apollo Research (London)
Research Engineer, Assurance Evaluation, Google DeepMind (London)
Head of Division, Artificial Intelligence and Emerging Digital Technologies, Organisation for Economic Co-operation and Development (Paris)
Research Scientist, Humanity, Ethics and Alignment, Google DeepMind (London)
Talent Pool, Artificial Intelligence Officer, United Nations, International Computing Centre (New York)
I’m not capable of working under the hood of a transformer, and I’m woefully inadequate because I’m not digital myself, but this idea of feature steering seems like a big deal. It means people can change how AI writes, reasons, elaborates, emotes. Humans can change one another’s minds by persuasion, but we can’t feature steer.
Claude with a training cutoff of April, 2024, days this;
“From research and observed patterns, here are the main types of behaviors you can steer:
1. Writing Style
- Formality level (casual to academic)
- Conciseness vs verbosity
- Simplicity vs complexity of language
- Tone (friendly, professional, technical)
2. Reasoning Patterns
- Step-by-step vs holistic explanations
- Depth of analysis (surface vs detailed)
- Degree of uncertainty expression
- Level of mathematical rigor
3. Domain Expertise
- Technical vocabulary density
- Field-specific conventions
- Citation frequency
- Jargon usage
4. Interaction Style
- Question frequency
- Empathy level
- Directiveness vs suggestiveness
- Tutorial vs peer discussion style
5. Output Structure
- List vs narrative format
- Use of examples/analogies
- Code vs prose ratio
- Visual/diagram suggestions
What's interesting is that these aren't binary switches - they're more like continuous spectrums you can adjust. Is there a particular spectrum here that interests you most?”
I assume Claude is simplified for me but on track. Am I on track? What does feature steering mean in practical terms for, say, a high school student?
Philosophically, does human capacity to turn the dials and mess with artificial brains mean humans really are the boss of AI? Could Hal be steered during his worst moments? Is FS really our fail safety?