Before we get on with today’s edition, a quick clarification about last week’s piece on the playbook for AI policy from the folks at the Manhattan Institute. I talked a bit about a tighter definition of ‘loss’, but as it turns out there was something very similar in the PDF version of the post that I neglected to mention. Check it out here.
As for this week, I have three papers for you: one reporting the impact of AI companions on feelings of loneliness, meditations on ‘choice engines’ from one of the figures behind the ‘nudge’ theory of behaviour change, and research finding that LLMs hallucinate even when trained solely on factual information.
Three things
1. A less lonely road
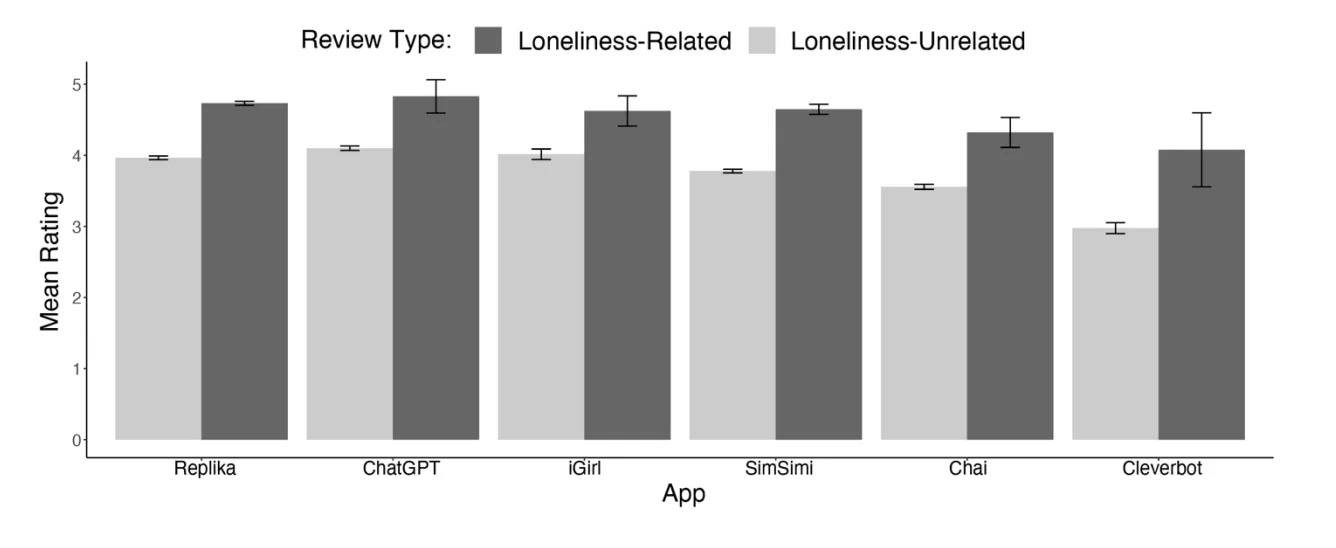
A new study by researchers at Harvard found that AI companions can reduce loneliness. The report, which came amidst the announcement of a new AI companion app, argued that interacting with chatbots may alleviate feelings of loneliness on a par with talking to another person – both immediately and over the course of a week.
In one experiment, participants' self-reported ‘loneliness scores’ dropped from 33.51 to 26.75 after a single interaction with an AI system, a decrease similar to that observed after talking with another person (38.40 to 31.29). This wasn't just a short-term effect, with a week-long study finding that participants consistently reported lower loneliness levels after daily AI interactions, with average scores decreasing from 36.64 before conversations to 30.74 afterwards.
These results are promising, but only show us part of the picture. It’s not all that clear, for example, whether a little AI companionship today causes the need for a lot of AI companionship tomorrow. While I find it hard to say that we should prevent people from using AI companions if they genuinely seem to decrease feelings of isolation and discomfort, it’s a trickier proposition to hold to that perspective if the use of companions creates its own set of problems further down the line.
That might include include the creation of new modes of dependency, mental heath issues or the degradation of human relationships, which may in turn engender a looping effect through which demand for AI companionship becomes a self fulfilling prophesy. Of course, we just don’t know until we get some good longitudinal studies set up to help us answer these sorts of questions. And even if we did, it’s not all clear to me that the state should prevent people from using AI companions if they know the risk.
2. Nudging towards utopia
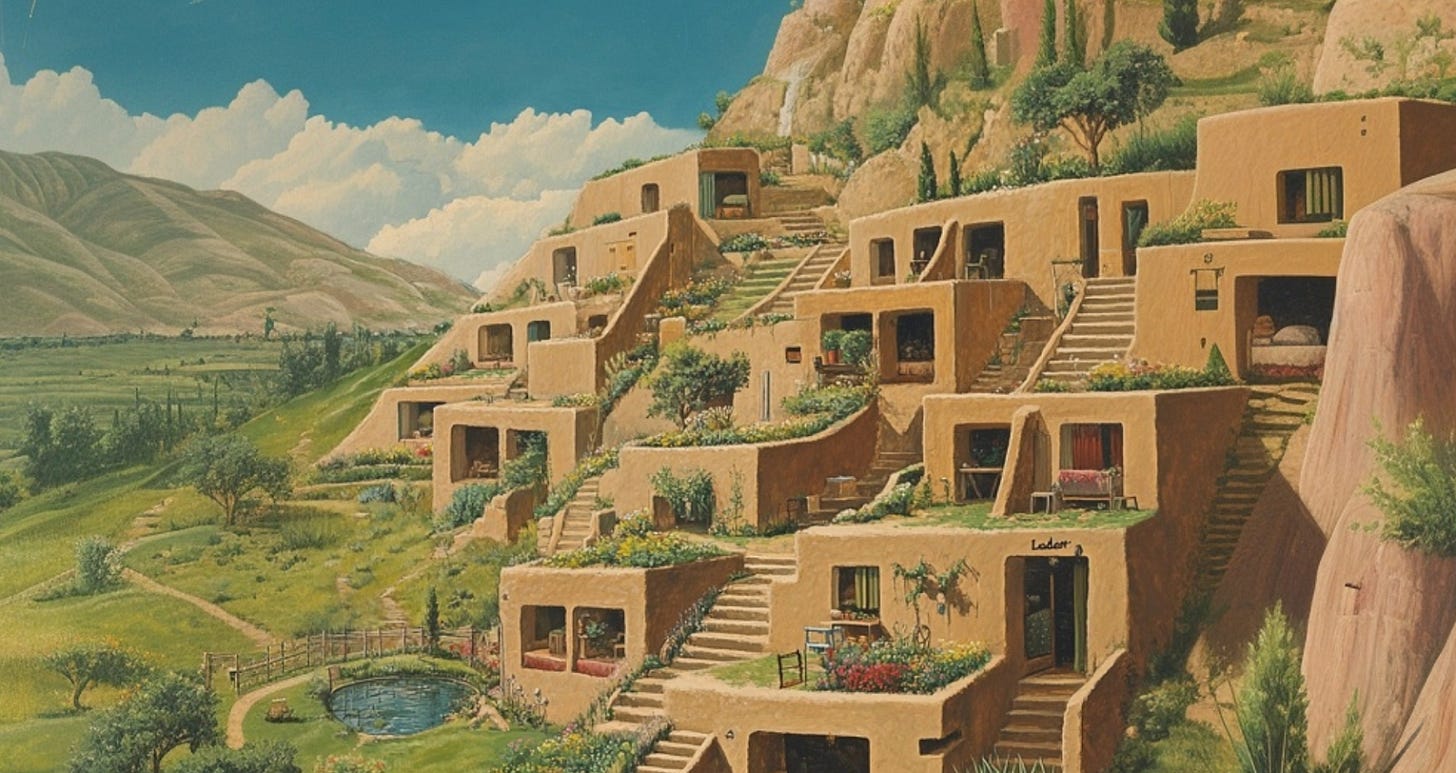
Speaking of paternalism, Cass Sunstein, he of ‘nudge theory’ fame, wrote about so-called AI "choice engines" that process information, provide recommendations, and assist in decision-making. These systems, Sunstein argues, could address both "internalities" (costs people impose on their future selves) and externalities (costs imposed by one party’s actions on another) when making decisions.
The paper introduces choice engines as instruments that allow users to input information about their preferences and receive tailored recommendations. Sunstein essentially sees the systems (which sound a lot like agents or assistants, though he doesn’t use those terms) as a way of overcoming behavioral biases like present bias, where people prioritise short-term gratification over long-term benefits.
Obviously this idea is a bit of a minefield. Providing a system with all the information (and authority) it needs to mediate our interaction with the world is clearly fraught with problems, many of which—like the amplification of biases or privacy concerns—Sunstein considers in the paper. But, funnily enough, his risk assessment also includes things like manipulation.
This is a bit of a strange choice as, at a fundamental level, such systems are explicitly designed to manipulate. When Sunstein talks about choice engines being “mildly paternalistic, moderately paternalistic, or highly paternalistic” he’s talking about the extent to which a system is capable of exerting influence. That’s not to say they represent some kind of nefarious plot, but rather the point of such tools is to influence the user to make ‘better’ decisions.
Because Sunstein says the role of choice engines is to “nudge or require consumers” to care about externalities, and because nudging is by definition a manipulative practice (in the sense that it covertly shapes behaviour), we are faced with the prospect of mass manipulation to improve consumer welfare. An economist’s dream, I’m sure, but eerily similar to B.F Skinner’s 1948 book Walden Two about a ‘utopian’ society premised on behavioural modification at scale.
3. How hallucinations happen
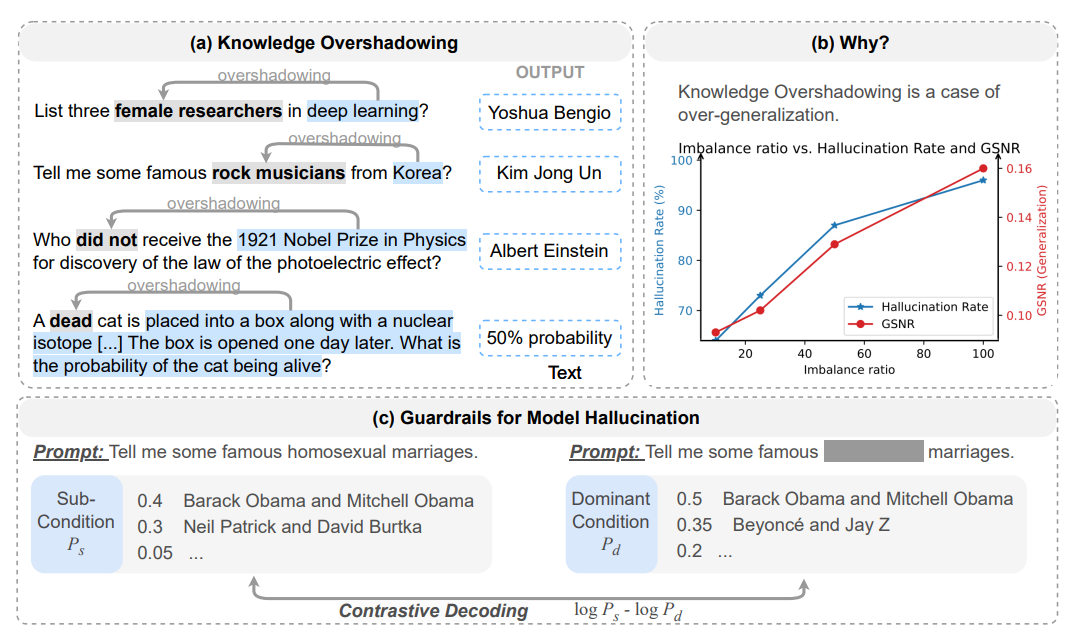
Researchers from the University of Illinois Urbana-Champaign identified a phenomenon they call "knowledge overshadowing" that leads to hallucinations in large language models. In a new paper, they show that when the models are queried with multiple ‘conditions’ (i.e. specific criteria that a correct response must meet), some tend to overshadow others, causing the model to generate incorrect outputs that blend multiple elements.
To make their case, the group considers asking a model about "female researchers in deep learning". The model might incorrectly name male researcher Yoshua Bengio, suggesting that the "deep learning" condition overshadowed the "female" condition of the response — a phenomenon that can occur even when models are trained only on factually correct data.
They also found that hallucination rates increase in tandem with the imbalance ratio between ‘popular’ and ‘unpopular’ conditions (those that appear more or less frequently in the training data), and the length of the ‘dominant’ condition description (the number of words used to describe the more common condition). In other words, the more unbalanced the training data is, and the more words you use to describe the common parts of your question, the more likely the model is to make a mistake.
The negative take here is that training solely on factually correct information isn't going to prevent a model from making mistakes, which isn't great news for methods to improve robustness like retrieval augmented generation in which external knowledge bases are tacked onto the model to improve reliability.
On the positive side, though, this work seems to represent a decent uplift on our understanding of hallucinations, and offers some practical ideas for reducing them (and yes, that is important, even if hallucinations are a feature and not a bug). All things considered, expect more work in this vein to help researchers dramatically improve reliability in the long run.
Best of the rest
Friday 2 August
Positive-sum symbiosis (Substack > highly recommend!)
Government shelves £1.3bn UK tech and AI plans (BBC)
UK’s AI bill to focus on ChatGPT-style models (FT)
AI Act enters into force (EU Commission)
US probes Nvidia’s acquisition of Israeli AI start-up (FT)
These AI firms publish the world’s most highly cited work (Nature)
Thursday 1 August
Musk’s xAI Has Considered Buying Character AI (The Information)
Using an AI chatbot or voice assistant makes it harder to spot errors (New Scientist)
Announcing Black Forest Labs (Black Forrest Labs)
Lessons from the FDA (AI Now Institute)
Safetywashing: Do AI Safety Benchmarks Actually Measure Safety Progress? (arXiv)
Wednesday 31 July
Exploring EU-UK Collaboration on AI: A Strategic Agenda (TBI)
Smaller, Safer, More Transparent: Advancing Responsible AI with Gemma (Google DeepMind)
Issue Brief: Foundational Security Practices (Frontier Model Forum)
Circuits Updates - July 2024 (Anthropic)
Brave New World? Human Welfare and Paternalistic AI (SSRN)
Why Science Will Never Explain Consciousness (PT)
Tuesday 30 July
News on Social Media Boosts Knowledge, Belief Accuracy, and Trust: A Field Experiment on Instagram and WhatsApp (OSF)
Perplexity AI will share revenue with publishers after plagiarism accusations (CNBC)
Meta’s Llama 405bn LMSYS score (X)
A Primer on the EU AI Act (OpenAI)
AI ‘Friend’ Company Spent $1.8 Million and Most of Its Funds on Domain Name (404)
Are we vastly underestimating AI? with Dwarkesh Patel — Episode 38 of Onward (Fundrise)
Data hegemony: The invisible war for digital empires (IPR])
Monday 29 July (and things I missed)
AI is actually making workers less productive (Worklife)
Why do people believe true things? (Substack)
How Can AI Accelerate Science, and How Can Our Government Help? (Carnegie Mellon University)
The GPT Dilemma: Foundation Models and the Shadow of Dual-Use (arXiv)
Tech Leaders, AI Innovators Underscore Importance of AI Safety Institute Ahead of Committee Consideration of Cantwell-Young Future of AI Innovation Act (US Gov)
When Do Universal Image Jailbreaks Transfer Between Vision-Language Models? (arXiv)
Generative AI in Real-World Workplaces (MSFT)
Reasoning through arguments against taking AI safety seriously (Yoshua Bengio)
AI Missteps Could Unravel Global Peace and Security To mitigate risks, developers need more training (IEEE)
Job picks
Some of the interesting (mostly) non-technical AI roles that I’ve seen advertised in the last week. As usual, it only includes new positions that have been posted since the last TWIE (but lots of the jobs from the previous edition are still open).
AGI Safety Manager, Google DeepMind (UK)
National Field Coordinator, Centre for AI Policy (US)
AI and Information Security Analyst, RAND (US)
Policy Analyst, Alignment, Center for AI Policy (US)
Senior Fellow and Director, Artificial Intelligence and Emerging Technology Initiative, Brookings Institute (US)
Product Policy Manager, Cyber Threats, Anthropic (US)
Strategy and Delivery Manager, Chem / Bio Misuse, UK AI Safety Institute (UK)
Head of Evaluations, Google DeepMind (UK)
Director, Institute for Artificial Intelligence, UN University (Italy)
Head of Operations, AI and Democracy Institute (Global)