More compute governance, forecasting AI, and algorithmic efficiency [TWIE]
The Week In Examples #28 | 16 March 2024
In a week with sophisticated software-developing agents and big movements in the world of robotics (two things we talked about in our look ahead to 2024 at the end of last year) it’s time to ask the question we’ve all been thinking: is deep learning hitting a wall? (For the avoidance of doubt, the answer is absolutely not.)
Today, we have another paper on compute governance, a study trying to reconcile forecasters’ views about the future of AI, and work proposing that algorithmic efficiency is doubling about every eight months. As always, it’s hp464@cam.ac.uk for comments, feedback or anything else!
Three things
1. Cloud providers weather governance recommendations
What happened? Another week, another study about compute governance following the previous report we looked at two weeks ago. From the folks at GovAI (who also wrote the previous effort) and the University of Oxford, this week’s intervention makes the case that compute providers are an underpriced part of the AI value chain for enforcing regulation. According to the authors, providers are useful for regulators in four respects: “as securers, safeguarding AI systems and critical infrastructure; as record keepers, enhancing visibility for policymakers; as verifiers of customer activities, ensuring oversight; and as enforcers, taking actions against rule violations.”
What's interesting? To wind it back a bit, compute providers are the organisations that provide the computational infrastructure and resources necessary for running and training AI models. They offer access to GPUs (graphics processing units), TPUs (tensor processing units), and other specialised hardware that can handle the massive computational demands of AI workloads. The authors make the case that these providers—such as cloud computing platforms like Amazon Web Services, Google Cloud, and Microsoft Azure—represent an important but underappreciated (relative to governance interventions focused on the model provider) avenue for implementing existing regulatory requirements and protecting against extreme risks.
What else? The report is interesting, though I do wonder about how much this work is about compliance with the EU AI Act and the US Executive Order on AI (which the authors mention in the first line of the report) versus creating mechanisms through which extreme risks can be mitigated. The two are not mutually exclusive, but—reading between the lines—I did get the sense that the authors were more interested in the latter than the former. As they write on the motivation for the construction of an oversight regime: “Expanding the role of compute providers to also record and validate domestic customers using compute at frontier AI thresholds could enable the US government to identify and address AI safety risks arising domestically.” Ultimately, compliance with the vast majority of the AI Act and Executive Order is possible through mechanisms other than compute governance, but compute governance is probably the single most important mechanism for addressing extreme safety risks.
2. Forecasters can’t see past differences
What happened? Researchers from the Forecasting Research Institute released a new paper detailing the results of an adversarial collaboration between groups with differing views on the risk of existential catastrophe from artificial intelligence by 2100. They found that the "concerned" group, composed mainly of AI risk experts, predicted a median 20% chance of AI causing an existential catastrophe by 2100. This figure sits in contrast to that provided by the "sceptical" group in the study, composed mainly of superforecasters, which predicted a median 0.12% chance of catastrophe. By catastrophe, they mean an “unrecoverable collapse” in which the human population shrinks to under 1 million for a million or more years, or global GDP falls to under $1 trillion (which Vox reckons is less than 1 percent of its current value) for a million years or more.
What's interesting? The disagreement between groups did not substantially narrow over the 8-week project, despite what the authors describe as “intensive discussion” over the duration of the project. Participants engaged with alternative views but did not find so-called short-term indicators (in this context, trigger points and trends existing before 2030) that explained most of the long-term disagreement. As a result, the groups seemed to have vastly different expectations about key issues like how long it will take to develop AI more capable than humans in all domains, how likely AI is to develop goals that lead to human extinction, and how adequately society will respond to the dangers posed by AI development.
What else? The upshot is that fundamental differences in worldview between the groups, like how much to trust theoretical arguments versus base rates (i.e. historical data and trends) in unprecedented situations, seem to underlie the disagreement more than differences in knowledge about AI systems. Partially, the paper explains some of the reasons why people can’t seem to see eye to eye about where the primary dangers from AI lie. Ultimately, AI is a container. It is so broad and so nebulous that we can’t help ourselves from projecting our own values and beliefs onto it. For that reason, I wouldn’t expect any reconciliation between those who disagree about the nature of AI any time soon.
3. Compute outperforms efficiency gains
What happened? Finally, we have a paper from researchers at Epoch and MIT making the case that algorithmic efficiency for language models doubles every eight months. The group reviewed over 200 language model evaluations on the WikiText and Penn Treebank datasets from 2012-2023 to find that the compute required to reach a set performance threshold has halved approximately every eight months over the last decade (with a 95% confidence interval of around 5 to 14 months).
What's interesting? Despite being substantially faster than the increases in processing power famously represented by Moore's Law, the group found that the increase in compute availability over the period of study made – unsurprisingly – an even larger contribution to overall performance improvements than efficiency during this period. As one of the researchers explained: “While algorithmic progress has been rapid, our Shapley value analysis suggests that 60-95% of the performance improvements stem from increased computing power and training data, while novel algorithms account for only 5-40% of the progress.” That being said, the authors do acknowledge that the introduction of the transformer architecture in 2017 represented something of a break with the overall trend, providing what the group describe as a 10x "compute-equivalent gain" for training AI models.
What else? You might expect algorithmic efficiency to be good news for the amount of compute used, meaning that firms will seek to use less energy to create a given model (bringing with it a few practical and environmental effects to boot). If I had to guess, though, I would say the opposite is more likely to be true. That’s because of our old friend the Jevons paradox, which holds that technological progress that increases the efficiency with which a resource is used tends to increase, rather than decrease, the rate of consumption of that resource. In other words, as technology makes it possible to use a resource more efficiently, total consumption of that resource may increase due to increased demand. Expect compute to be no different, with more efficient algorithms meaning that the biggest models use more computational power – not less.
Best of the rest
Friday 15 March
Use of artificial intelligence in government (UK National Audit Office)
Is this AI conscious? (Vox)
Apple Buys Canadian AI Startup as It Races to Add Features (Bloomberg)
What's after Nvidia in the AI rally? (FT)
OpenAI Tumult Raises Question of How Open an AI Company Should Be (Bloomberg)
'Journalists are feeding the AI hype machine' Published (BBC)
Thursday 14 March
Artificial Intelligence Act: MEPs adopt landmark law (European Parliament)
Cybersecurity and AI: The Evolving Security Landscape (CAIS)
Regulators Need AI Expertise. They Can’t Afford It (Shameless self promotion >> WIRED)
In Event Of An (AI) Emergency: Interpreting Continuity Of Government Provisions In State Constitutions (Santa Clara High Tech Journal)
Wednesday 13 March
Call for applications to ARIA compute programme opens (X)
Claude matches GPT-4 on the LMSYS Arena (LMSYS >> X)
Model commoditization and product moats (Interconnects >>> Substack)
EU Embraces New AI Rules Despite Doubts It Got the Right Balance (Bloomberg)
Europe’s content police have a new target: generative AI (Politico)
Generative AI's privacy problem (Axios)
How insurance can help build trust in artificial intelligence (WIRED)
Tuesday 12 March
NIST to Launch Competition for AI-Focused Manufacturing USA Institute (NIST)
AI safety is not a model property (AI Snake Oil >>> Substack)
Healthcare leaders and Microsoft team up to promote 'responsible' integration of AI and medicine (Fast Company)
Physical Intelligence Is Building a Brain for Robots (Bloomberg)
The Kate Middleton scandal shows the worst of a generative-AI-filled social media world (Fast Company)
Western Countries Are More Pessimistic About AI (Axios)
Monday 11 March
Responsible Scaling: Comparing Government Guidance and Company Policy (IAPS)
Annual threat assessment of US intelligence community (US Government)
Exclusive: U.S. Must Move ‘Decisively’ to Avert ‘Extinction-Level’ Threat From AI, Government-Commissioned Report Says (TIME >>> Gladstone)
Beware the Frothy AI Frenzy (WSJ)
AI talent war heats up in Europe (Reuters)
Nvidia is sued by authors over AI use of copyrighted works (Reuters)
Your guide to AI: March 2024 (Air Street Press)
Space loves AI, AI doesn’t love Space (SpaceNews)
Job picks
These are some of the interesting (mostly) non-technical AI roles that I’ve seen advertised in the last week. As a reminder, it only includes new roles that have been posted since the last TWIE, though many of the jobs from the previous week’s email are still open. If you have an AI job that you think I should advertise in this section in the future, just let me know and I’d be happy to include it!
AI Policy, Program Manager, Mozilla Foundation, Global (Remote)
AI Policy Analyst, Americans for Responsible Innovation, Washington D.C.
Deputy Director AI Policy Analysis, UK Gov, London
Senior Visiting Research Fellow, Legal Priorities Project, Global
Senior Policy Analyst / Economist, Center for AI Policy, Washington D.C.
Product Manager, Trust and Safety, Stability AI, London
Technology Specialists, Artificial Intelligence, EU, Brussels/Luxembourg
AI Policy Advisor, DLI Piper, Washington D.C.
Digital Futures Senior Fellow, Planetary Politics Initiative, Washington D.C.
Cambridge ERA:AI Fellowship (Summer 2024), Existential Risk Alliance, Cambridge UK
One for the road
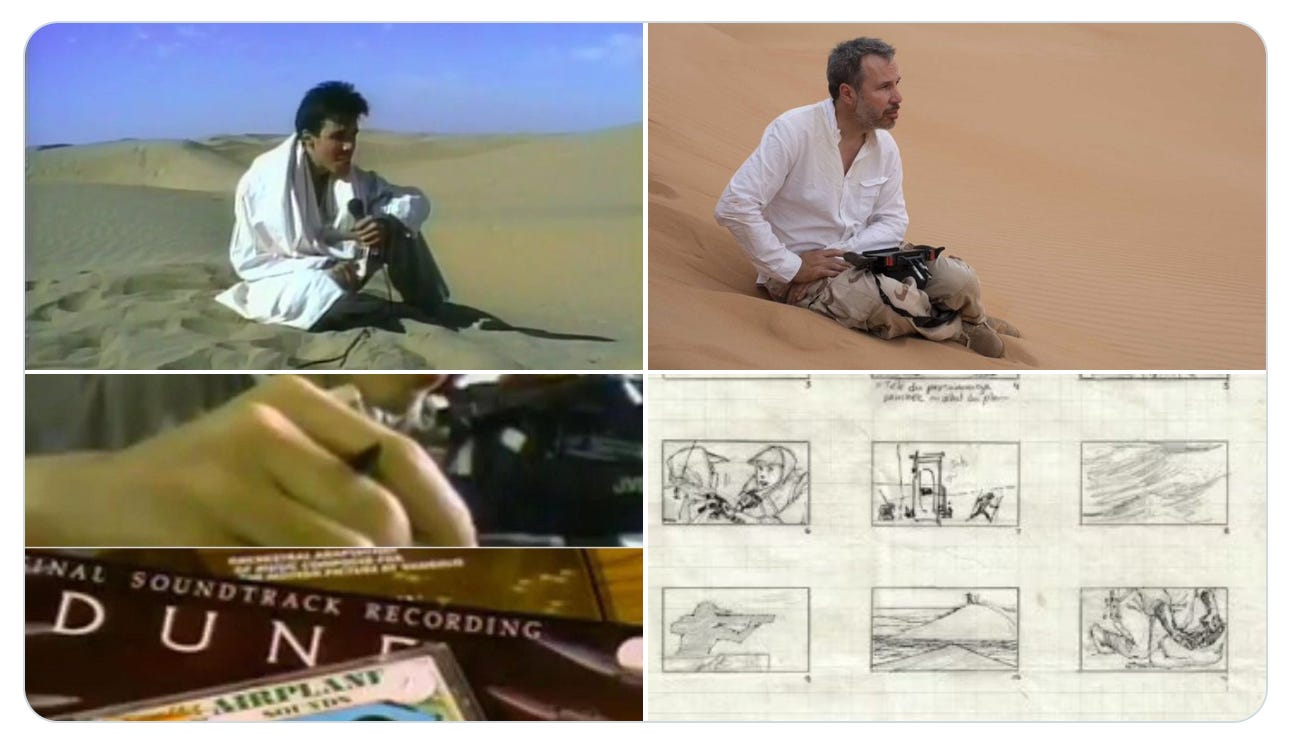