This week was the week of open weight large models. First, Meta’s much anticipated 405bn version of Llama 3 (now 3.1), was released alongside a no holds barred letter from Mark Zuckerberg explaining why the company will continue to share model weights despite concerns that the practice may eventually cause more harm than good. Second, WordArt enthusiasts the world over were glad to see a new model from French AI darling Mistral, which released a new flagship model in the form of Mistral Large 2.
Both perform pretty well across a few different benchmarks (see here for Mistral and here for Llama 405bn), both look like they pass the EU’s 10^25 floating point operations (FLOPs) threshold for additional reporting requirements, and both aren’t actually ‘open source’ due to licence restrictions that limit certain types of usage.
Away from open-ish models, this week also saw OpenAI release a long awaited search product that seems to be pretty similar to the approach taken by Google and Perplexity, while Google DeepMind rearranged prediction markets by announcing that its AI system had taken a silver medal at the International Mathematical Olympiad.
Three things
1. Crisis for crawlers
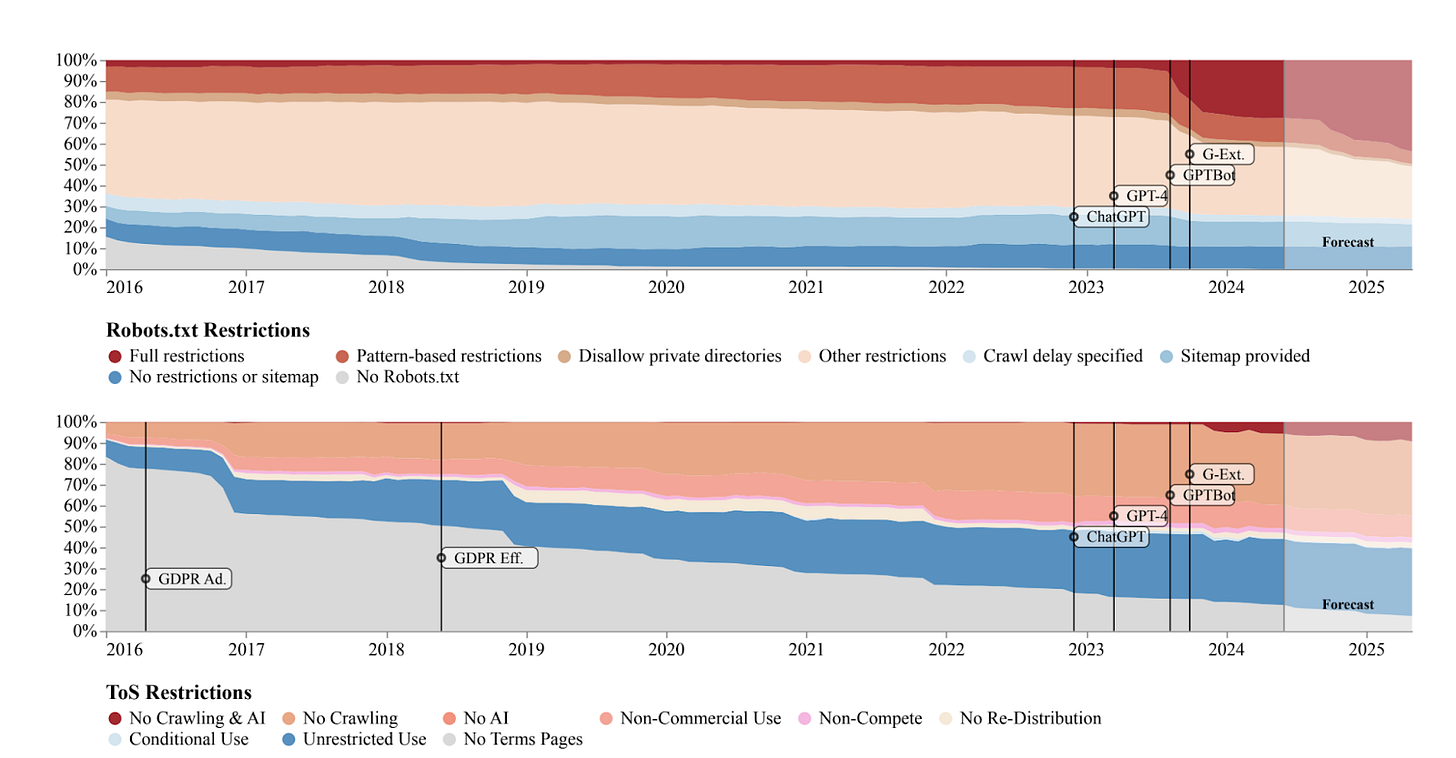
A new report from the Data Provenance Initiative, a collective of researchers that audit datasets used to train AI models, argued that the large-scale scraping of web data for training AI has provoked the introduction of data restrictions from web sources. In the “first, large-scale, longitudinal audit of the consent protocols for the web domains underlying AI training corpora”, the group found that over 28% of critical sources in C4 (a popular dataset comprised of crawled content) is now fully restricted from use.
Web data is traditionally collected using web crawlers. These are simple bots that begin from a list of known URLs and follow hyperlinks to discover new pages, download and store information, and organise it into something useful. Websites generally want to allow crawlers because it helps search engines discover them, though to prevent overloading they use the well-known robots.txt file that tells the crawlers which bits of the site are off limits.
But as the researchers explain, the Robots Exclusion Protocol (usually implemented via the robots.txt file) was not designed with AI in mind. As a result, they reckon that the inability of robots.txt to “communicate the nuances in how content creators wish their work to be used, if at all, for AI” is driving a wave of rejections. The upshot, according to the group, is that such practices may stymie academic research efforts, pose fairness issues by biassing developers towards older data, and prevent firms from collecting the data they need to scale models.
2. Misunderstanding model collapse
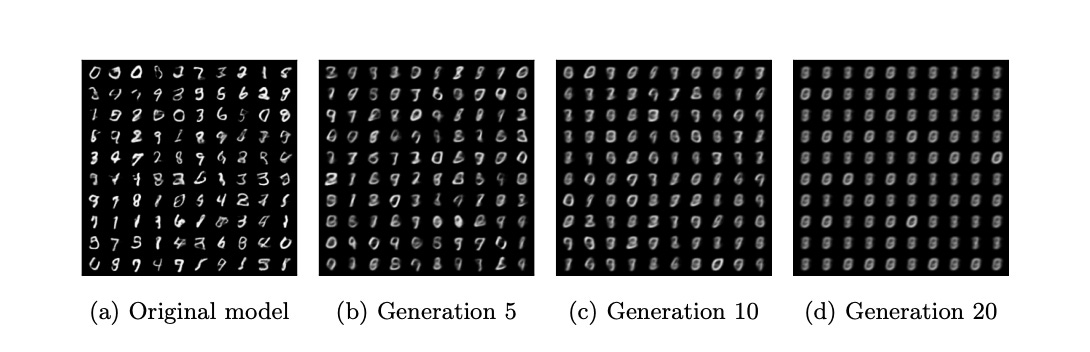
Model collapse is back on the agenda. The idea, which refers to a degenerative process through which performance degrades over time when trained on AI generated outputs, was the subject of a new paper in Nature from researchers at the University of Oxford. To test for model collapse, the group fine-tuned language models, variational autoencoders (VAEs), and Gaussian mixture models (GMMs) using data produced by previously fine-tuned versions of the same model.
For language models, they found the ‘perplexity score’ of a model (in this case a metric evaluating the likelihood of a sequence of words) worsened significantly when trained on the outputs from a previous version. According to the group, while the original model fine-tuned with real data achieves a perplexity score of 34, the perplexity score for models trained for five epochs on generated data increases to between 54 and 62. (A higher figure indicates worse performance because the perplexity score essentially measures the inaccuracy of predictions.)
The problem, though, is that the Oxford researchers trained on 90% synthetic data and 10% original data for each new generation of training. Compare this approach with a recent paper from Stanford in which synthetic data is added incrementally over time: in the first round the model uses no synthetic data, half of the data is synthetic in the second round, two thirds is synthetic in the third round, and three quarters is synthetic in the fourth round.
Using this aggregation method, the Stanford group found minimal evidence of model collapse. As a result, the group said that the results “demonstrate that accumulating successive generations of synthetic data alongside the original real data avoids model collapse.” Given that real usage is based on accumulation rather than replacement, the upshot is that model collapse tends to appear when researchers conduct studies that bear little resemblance to real technical practice.
3. A playbook for AI policy
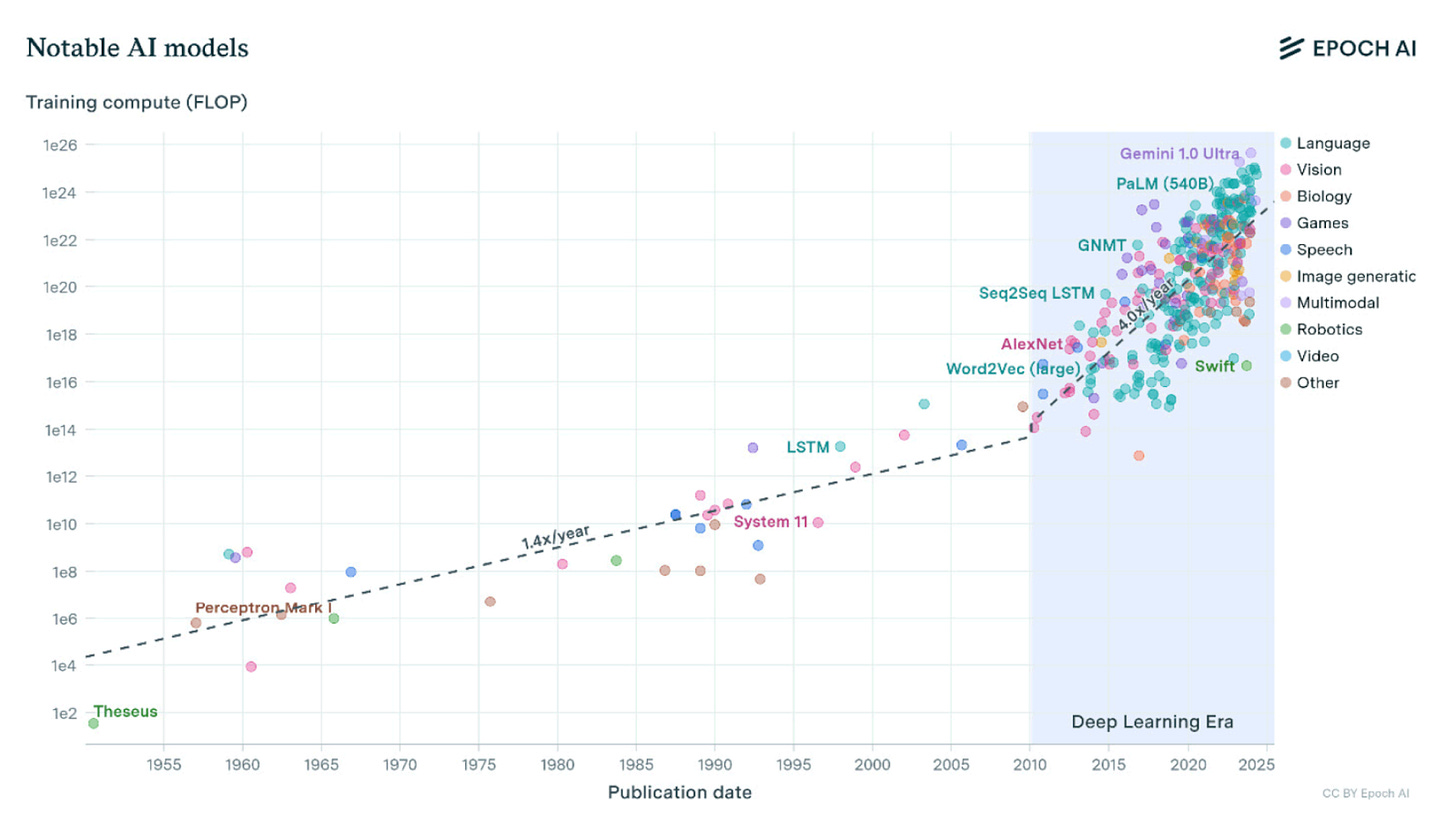
Nick Whitaker at the Manhattan Institute wrote a blog outlining a series of AI policy recommendations for the United States based on four fundamental principles: maintaining American AI leadership, protecting against threats, building state capacity, and protecting human dignity. The goal of the ‘playbook’ is to tread a middle way between providing the conditions for firms to develop the next generations of models, while seriously grappling with the national security risks posed by AI development.
The piece offers a nice rundown of recent AI progress from about 2010 or so before suggesting that the infamous AI ‘scaling laws’ can be used to “predict AI progress, given the size of an AI, the amount of data that it trains on, and the computing power used in that training.” I suspect this is more or less right, but—at the risk of nit picking—it is worth saying that the scaling laws themselves specifically gauge a model’s loss: a measure of how well its predictions match the actual outcomes during training. Lower loss is correlated with increased performance, which is in turn correlated with the emergence of new capabilities (though no one really knows how this relationship works in practice).
Based on this handy primer, Whitaker sketches a number of policy recommendations connected to the overarching four principles (leadership, threat protection, state capacity, and human dignity). These include developing and implementing new standards headed by the Bureau of Industry and Security (BIS); visa exemptions for foreign researchers working in AI; removing some provisions in the CHIPS act; and allowing BIS to restrict the use of American cloud compute resources to authoritarian countries.
Best of the rest
Friday 26 July
Managing Misuse Risk for Dual-Use Foundation Models (US AI Safety Institute)
Video game performers will go on strike over artificial intelligence concerns (AP)
AI expert to lead Action Plan to ensure UK reaps the benefits of Artificial Intelligence (UK Gov)
This is how AI can revive America’s middle class (Fast Company)
Despite Bans, AI Code Tools Widespread in Organizations (Infosecurity Magazine)
GovAI Annual Report (GovAI)
Thursday 25 July
Under the radar? Examining the evaluation of foundation models (Ada Lovelace Institute)
Who Will Control the Future of AI (Sam Altman for Washington Post)
Sihao Huang on the risk that US–China AI competition leads to war (80,000 Hours)
SearchGPT Prototype (OpenAI)
AI achieves silver-medal standard solving International Mathematical Olympiad problems (Google DeepMind)
Wednesday 24 July
Improving Model Safety Behavior with Rule-Based Rewards (OpenAI)
Transforming risk governance at frontier AI companies (CLTR)
Large Enough (Mistral)
Dwarkesh Patel’s Quest to Learn Everything (YouTube)
US-China perspectives on extreme AI risks and global governance (arXiv)
Tuesday 23 July
Open Source AI Is the Path Forward (Meta)
Poll Shows Broad Popularity of CA SB1047 to Regulate AI (FLI)
Ireland’s datacentres overtake electricity use of all urban homes combined (The Guardian)
Reclaiming AI for Public Good w/ Eleanor Shearer (Spotify)
Chips for Peace: how the U.S. and its allies can lead on safe and beneficial AI (LawAI)
Monday 22 July (and things I missed)
Generative AI Can Harm Learning (SSRN)
Open Problems in Technical AI Governance (arXiv)
Why does consciousness remain such a vexing puzzle for physics? (First Principles)
The Adoption of ChatGPT (Squarespace)
‘Social’ Mitochondria, Whispering Between Cells, Influence Health (Quanta)
On the Limitations of Compute Thresholds as a Governance Strategy (arXiv)
The return on the bicameral mind (Substack)
Job picks
Some of the interesting (mostly) non-technical AI roles that I’ve seen advertised in the last week. As usual, it only includes new positions that have been posted since the last TWIE (but lots of the jobs from the previous edition are still open).
Delivery Manager / Technical Project Manager, Societal Impacts, UK Gov (London)
Program Director, Supervised Program for Alignment Research (Remote)
Research Assistant, Timaeus (Remote)
Director, US AI Governance, The Future Society (Remote)