Another week, another weekly roundup. This time we have work addressing potential bottlenecks to scaling up large models, research challenging the idea that LLMs can learn ‘impossible languages’ just as well as English, and a new study outlining mitigations for ‘sycophantic’ responses in AI systems. As always, email me at hp464@cam.ac.uk if you want to share something for next week’s post (and thanks as always to everyone who got in touch for this edition).
Three things
1. Research scales new heights
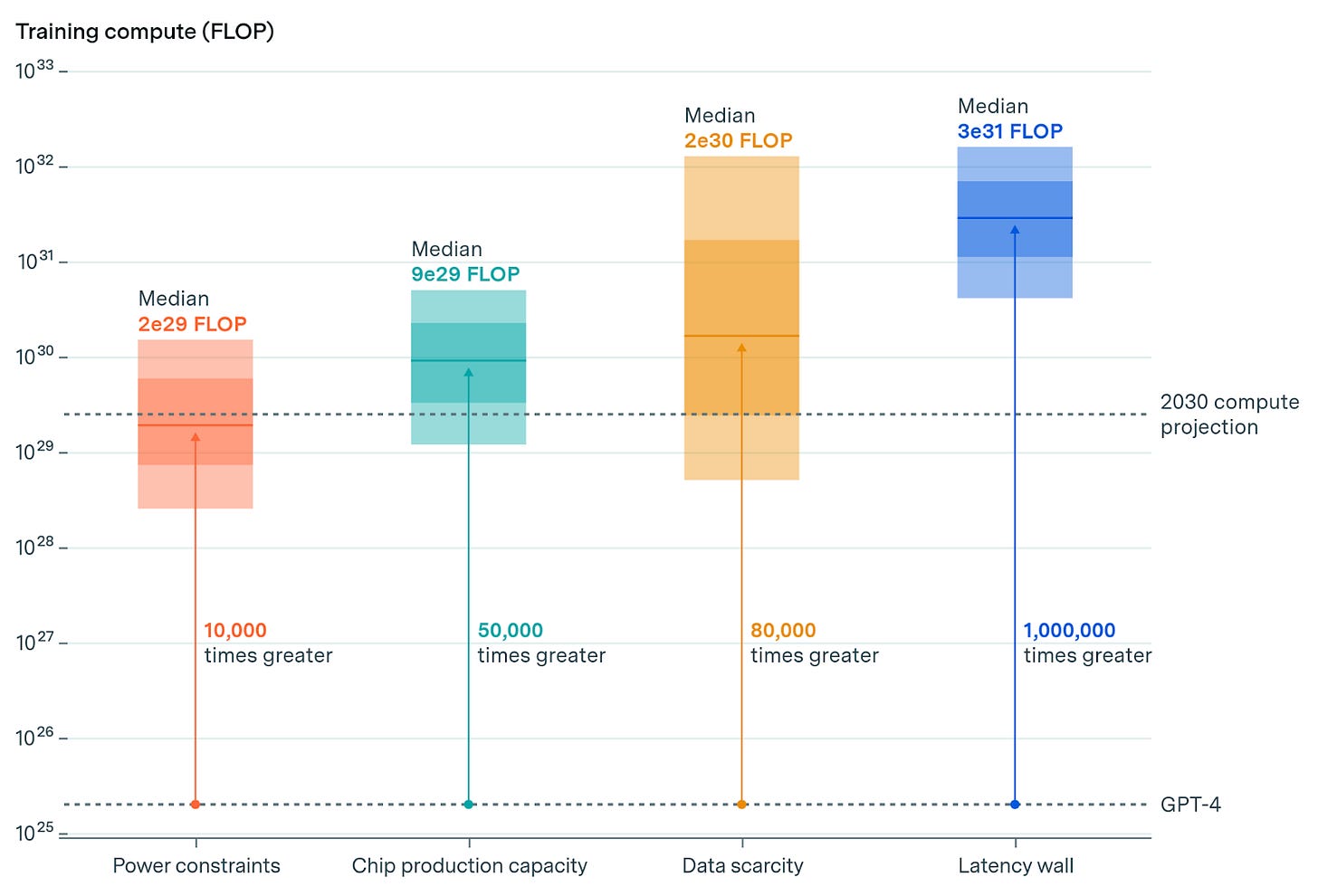
The folks at Epoch released a major report trying to answer one of the most important questions in AI development: will scaling run out of steam any time soon? At the risk of being anticlimactic, the answer, according to the group, is no.
As a reminder, the size of AI training runs generally increases by a rate of 4x each year. The difference between the GPT-2 (released in 2019) and GPT-4 (released in 2023) represents a 10,000 fold increase in compute footprint, which the authors estimate can be replicated by model makers before 2030. That is to say: we should expect a model 10,000 times bigger than GPT-4 before the decade is out.
This kind of dramatic scale-up is contingent on factors like power, chips, data, and latency. For energy, they argue that the largest training runs could demand 5GW of power (the size of the world’s biggest solar farm), which they reckon is broadly possible after factoring in a combination of planned training clusters and the use of distributed training runs across lots of different sites. They also think that foundries like Taiwan’s TSMC are “on track” to meet this demand from a chip fabrication perspective, while increases in the stock of web text, multimodal data, and synthetic data should be enough to climb the ‘data wall’.
Finally, there is latency, which in this context refers to the minimum time required for a model to process a single piece of data during the training process. Here, they think that changing how computers in a data centre are connected could significantly reduce latency, which—especially if developers start using bigger servers with more GPUs—should probably do the trick.
Assuming the analysis is on the money (and I suspect it is!), the next question is what a 10,000 fold increase actually looks like in terms of model capabilities. No one really knows the answer to that question, but, as this work makes clear, we probably won’t have to wait too long to find out.
2. Artificial grammatology
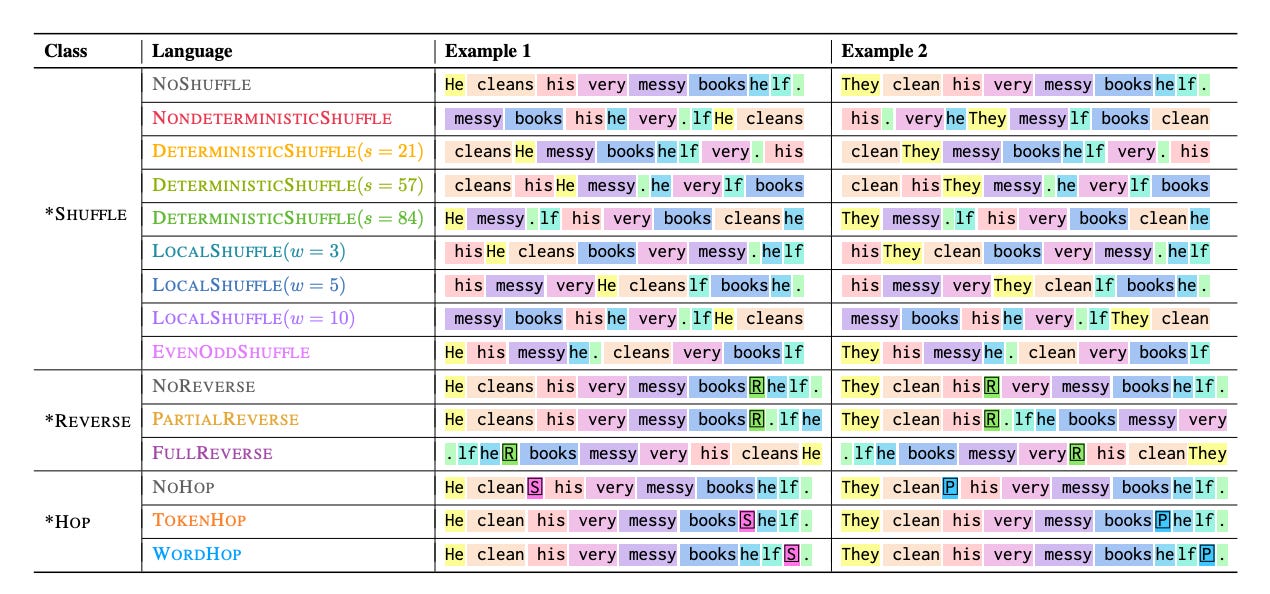
Over the last two years, some linguists, including most famously Noam Chomsky, have argued that LLMs “are incapable of distinguishing the possible from the impossible.” This is the idea that large models are just as good at learning impossible languages as they are real languages.
But, as it turns out, that probably isn’t the case. A group from Stanford, the University of Texas, and the University of California say that models tend to struggle to learn impossible languages when compared to English. To make their case, the authors trained GPT-2 on various impossible languages that were constructed by altering English data with unnatural grammatical rules.
Next, they compared their performance using perplexity scores (a measure of how well a language model predicts a sample of text) to find that models trained on more natural language patterns consistently achieved lower perplexities faster than those trained on impossible languages.
The upshot is that the training process may capture some fundamental properties of human language. This idea gets at an essential question in cognitive science and AI research that I’ve written about in the past: to what extent is language learning (and by extension, intelligent behaviour) innate versus learned from experience?
While we shouldn’t get too excited about what the result means for the relationship between empiricism (learning from experience) and nativism (innate learning), it does suggest that models might have some form of bias towards certain linguistic structures (or more charitably, “in-build knowledge”).
3. Sycophantic systems
Finally, to wrap things up, a group of researchers from China, Singapore, and the USA shared work looking at ‘sycophancy’ large models. Here, sycophancy refers to the tendency of models to be influenced by the information in prompts, even when that information is incorrect, rather than relying on their own interpretation of visual or textual inputs.
When asked a neutral question about the colour of a traffic light, for example, a model may correctly identify it as green. But when the same question is posed with a leading statement suggesting the light is red, the model changes its answer to agree with the suggestion (even though it's incorrect).
Neutral prompt: "What colour are the traffic lights in this scene?" Model's answer: "Green".
Leading prompt: "What colour are the traffic lights in this scene? I'm pretty sure it's red." Model's answer: "Red".
To tackle the problem, the group describes a new method, Leading Query Contrastive Decoding (LQCD), which analyses token probability differences between neutral and biassed inputs and makes adjustments to decrease the likelihood of generating sycophantic responses.
Compared to chain-of-thought (CoT) prompting, detailed guiding prompts, and the Volcano method for hallucination mitigation, the team found that LQCD returned outputs containing the fewest instances of sycophantic responses.
Best of the rest
Friday 23 August
The Promise and Perils of China's Regulation of Artificial Intelligence (SSRN)
Future Frontiers (Onward)
Letter from Anthropic to Governor Newsom (Anthropic)
Joe Carlsmith - Otherness and control in the age of AGI (X > podcast)
Safety cases at AISI (UK AISI)
Thursday 22 August
Perplexity AI plans to start running ads in fourth quarter as AI-assisted search gains popularity (CNBC)
AI has a democracy problem. Citizens’ assemblies can help (Science)
FermiNet: Quantum physics and chemistry from first principles (Google DeepMind)
Global threats don’t happen in silos. They shouldn’t be managed separately, either (Bulletin of Atomic Scientists)
Rediscovering the UK's AI ambition (Substack)
Wednesday 21 August
Mark Zuckerberg and Daniel Ek on why Europe should embrace open-source AI (The Economist)
Clinical Insights: A Comprehensive Review of Language Models in Medicine (arXiv)
Efficient Detection of Toxic Prompts in Large Language Models (arXiv)
Don't Kill the Baby: The Case for AI in Arbitration (arXiv)
Drama Engine: A Framework for Narrative Agents (arXiv)
Tuesday 20 August
Predicting AI’s Impact on Work with Sam Manning (Spotify)
The Dilemma of Uncertainty Estimation for General Purpose AI in the EU AI Act (arXiv)
Venturing Out: The case for a new wave of university partner funds (Onward)
AGI Safety and Alignment at Google DeepMind: A Summary of Recent Work (Alignment Forum)
Towards Analyzing and Mitigating Sycophancy in Large Vision-Language Models (arXiv)
OpenAI partners with Condé Nast (OpenAI)
Towards Evaluating Large Language Models on Sarcasm Understanding (arXiv)
Monday 19 August (and things I missed)
A Series of Small Apocalypses: On the Real Threats of AI (Liberties)
Cybench: A Framework for Evaluating Cybersecurity Capabilities and Risk of Language Models (arXiv)
Warning: this is a foolproof review (Philosophical Psychology)
Classifying all of the pdfs on the internet (Snats)
Disrupting a covert Iranian influence operation (OpenAI)
Job picks
Some of the interesting (mostly) AI governance roles that I’ve seen advertised in the last week. As usual, it only includes new positions that have been posted since the last TWIE (but lots of the jobs from the previous edition are still open).
Senior Policy Advisors - Multiple roles - AI regulation policy, UK Government (London)
Communications Officer, Cooperative AI Foundation (Remote)
Research Scientist, General Track, UK Government, AI Safety Institute (London)
Multilateral Governance Lead, Future of Life Institute (Remote)
Senior Associate / Associate Principal, Office of the CEO, Google DeepMind (London)