After two weeks on holiday in Trinidad and Tobago, I am back with another edition of The Week In Examples. Today, we have the release of Claude 3 from the folks at Anthropic, a paper getting under the skin of the much-loved LMSYS Chatbot Arena, and commentary from Nature on the epistemic risk posed by scientists’ use of AI. As always, it’s hp464@cam.ac.uk for comments, feedback or anything else!
Three things
1. The opus of the masses
What happened? Anthropic released Claude 3, a family of models of varying sizes known as ‘Haiku’, ‘Sonnet’, and ‘Opus’ (for the record, I don’t mind a naming scheme on the whimsical side). Of these three models Opus is the largest and most capable, achieving 86.7% on the MMLU benchmark, which the firm compared to GPT-4’s 86.4% result. That’s pretty good, but it is worth saying that the 86.4% represents the score achieved by GPT-4 when it was released in March last year, not the most recent iteration of the model (that version, GPT-4 Turbo, reportedly scores 90.10% on MMLU). As Anthropic acknowledged in a footnote: “we’d like to note that engineers have worked to optimize prompts and few-shot samples for evaluations and reported higher scores for a newer GPT-4T model.”
What's interesting? Quibbles over evaluation metrics aside, Claude Opus is a really good model. According to the LMSYS Arena, it has an ELO of 1233 versus 1251 for GPT-4 Turbo (which, as we will see in the next section, is a much better way of assessing how useful large models are in the real world). That the model performs well is all the more interesting given Anthropic said that it used “data we generate internally” i.e. synthetic data—amongst data from the internet and third parties—to train the model. It makes sense given CEO Dario Amodei said that he thought data was “not likely to be a blocker” for building increasingly capable models, though nonetheless it’s good to see this intuition supported by a new system. This might seem a bit prosaic, but the implications are potentially very important: model collapse is not going to prevent scaling. At this point, I wouldn’t be surprised that the main blocker to scaling is good old fashioned economics. If models don’t start to create real value even when training runs cost billions of dollars, no-one is going to foot a subsequent run that could feasibly total tens of billions dollars.
What else? Anthropic has taken some flack from people who think that the company’s leadership misled the public about the extent to which the group would push forward the state of the art. Detractors cited comments from Dario Amodei on the Dwarkesh Podcast and the FLI Podcast where he said the group “didn’t cause" the acceleration and that Anthropic “shouldn't be racing ahead” respectively. The rub, though, is that it’s not all that clear to me that Claude 3 does represent a major jump in capabilities. It’s a very impressive model—and no doubt the best in class at certain tasks—but staying at the frontier isn’t the same as pushing it forward. It’s also worth saying that, while the company emphasised safety when it was founded in 2021, it also said: “down the road, we foresee many opportunities for our work to create value commercially and for public benefit.”
2. In the arena, trying things
What happened? I’ve been wanting to write a bit on the LMSYS Arena for a while now, so imagine how happy I was to see the group behind the leaderboard release a paper explaining the project and the methodology that underpins it. For those new to the world of dynamic evaluation, the Arena works by pitting two LLMs against each other to answer a specific prompt on a blind basis. A user enters a prompt and is presented with two options in response, which they then rate against each other. You can see the leaderboard here, which has GPT-4 Turbo at the top, followed by Claude, and Gemini Pro.
What’s interesting? According to the authors, “benchmarks can be categorized based on two factors: the source of questions (either static or live) and the evaluation metric (either ground truth or human preference).” Using this framing, the majority of popular evaluations (such as MMLU) fall into the ‘static, ground truth’ bucket. The problem with these types of evaluations is that they aren’t open-ended, which means they struggle at capturing the flexible and interactive use found in real-world settings. Worse still, the tests become increasingly likely to feature in training data over time, which means that their reliability wanes with the release of each new model.
What else? LMSYS is obviously not perfect—remember, it’s testing the relative capability of models versus their peers, not their absolute performance—but it is much better than static tests. The approach works at the interaction layer of LLM evaluation, which is between upstream capability analysis and a downstream assessment of how people actually use the models in the real world. That it has quickly developed such a large and loyal following in a relatively short space of time shows that static benchmarks don’t tell us much about how good models really are. I expect to see more interaction layer evaluations in the future, as well as those that draw into focus the impact of AI on society by monitoring usage after deployment.
3. AI no scientific silver bullet
What happened? Nature’s editorial team wrote on concerns that scientists are placing too much trust in the use of AI tools. The problem, according to the magazine, is that “researchers envision such tools [AI] as possessed of superhuman abilities when it comes to objectivity, productivity and understanding complex concepts.” The editorial cites a recent piece outlining the epistemic risk posed by the use of AI in scientific research. In this article, researchers shared the results of a study based on the review of 100 peer-reviewed papers, preprints, conference proceedings, and books to catalogue the use of AI to summarise literature, assess findings, and conduct quantitative analyses.
What's interesting? The researchers identified three major epistemic risks associated with the use of AI in science: the illusion of explanatory depth, where individuals overestimate their understanding when using algorithms; the illusion of exploratory breadth, leading to a focus on AI-compatible studies at the expense of broader inquiry; and the illusion of objectivity, where AI's inherent biases are overlooked, assuming it offers a neutral perspective. I like all of these points a lot, especially since they represent something of a corrective to the idea that AI only represents upsides for scientific practice. On balance, AI probably will accelerate science—but that is more likely to happen if we acknowledge the risks as well as the rewards.
What else? Meanwhile, the folks at Nature Reviews Physics (one of Nature’s specialist magazines) discussed the limitations of using AI in scientific writing. In a quite good (and quite damning) article, the editors of the journal said “Good writing is about having something interesting and original to say. Generative AI tools might provide technical help, but they are no substitute for perspective”. In both examples, the discussion reminds me of Henry Kissinger’s 2019 essay ‘How the Enlightenment Ends’ that argues that the use of AI will precipitate a wave of scientific deskilling as we delegate the pursuit of new knowledge to machines. I don’t think that is a future we are consigned to, but I do think that there is a risk that we begin to favour fish over the fishing rod.
Best of the rest
Friday 8 March
The Surprising Power of Next Word Prediction: Large Language Models Explained, Part 1 (CSET)
AI mishaps are surging – and now they're being tracked like software bugs (The Register)
France Has AI Talent — But Can Macron Lure Investors? (Bloomberg)
Survey: Consumers don't want AI-generated news (AT)
NIST staffers revolt against expected appointment of ‘effective altruist’ AI researcher to US AI Safety Institute (VentureBeat)
Thursday 7 March
Inflection-2.5: meet the world's best personal AI (Inflection)
Inflection AI's friendly chatbot tops 1 million daily users (Axios)
AI likely to increase energy use and accelerate climate misinformation – report (The Guardian)
Altman-backed Oklo nuclear facility ramps up as AI industry looks to add energy sources (NBC)
AI watermarks aren’t just easy to defeat—they could make disinformation worse (Fortune)
Wednesday 6 March
OpenAI pens response to Musk lawsuit: 'We're sad that it's come to this' (TechCrunch)
Microsoft compares The New York Times’ claims against OpenAI to Hollywood’s early fight against VCR (CNBC)
Microsoft engineer sounds alarm on company's AI image generator in letter to FTC (BI)
This agency is tasked with keeping AI safe. Its offices are crumbling (Washington Post)
Layoffs surged in February to highest level since 2009 — and AI is a big reason (New York Post)
Tuesday 5 March
Consciousness, Machines, and Moral Status (PhilArXiv)
A safe harbor for AI evaluation and red teaming (Substack)
Microsoft derides ‘doomsday futurology’ of New York Times’ AI lawsuit (FT)
Concern as the gambling industry embraces AI (BBC)
AI can be easily used to make fake election photos (BBC)
New approach to regulating AI (Axios)
China’s top advisory body told AI gap with US is widening (South China Morning Post)
Monday 4 March
Goals for the Second AI Safety Summit (GovAI)
Apple Is Playing an Expensive Game of AI Catch-Up (WSJ)
Why AI could boost the economy faster than past technologies – Axios
Musk vs. moguls: Billionaire rivalries erupt in fight for AI's future – Axios
Antitrust and AI Issues Continue to Mold Corporate Landscape – Bloomberg Law
Job picks
These are some of the interesting non-technical AI roles that I’ve seen advertised in the last week. As a reminder, it only includes new roles that have been posted since the last TWIE—though many of the jobs from the previous week’s email are still open. If you have an AI job that you think I should advertise in this section in the future, just let me know and I’d be happy to include it!
Head of the International AI Safety Report, UK Government, London
AI Policy Fellowship, IAPS, Global
Special Projects Lead, UK Government, London
Responsibility Product Manager, Google DeepMind, London
Summer Research Fellowship, CLTR, London
One for the road
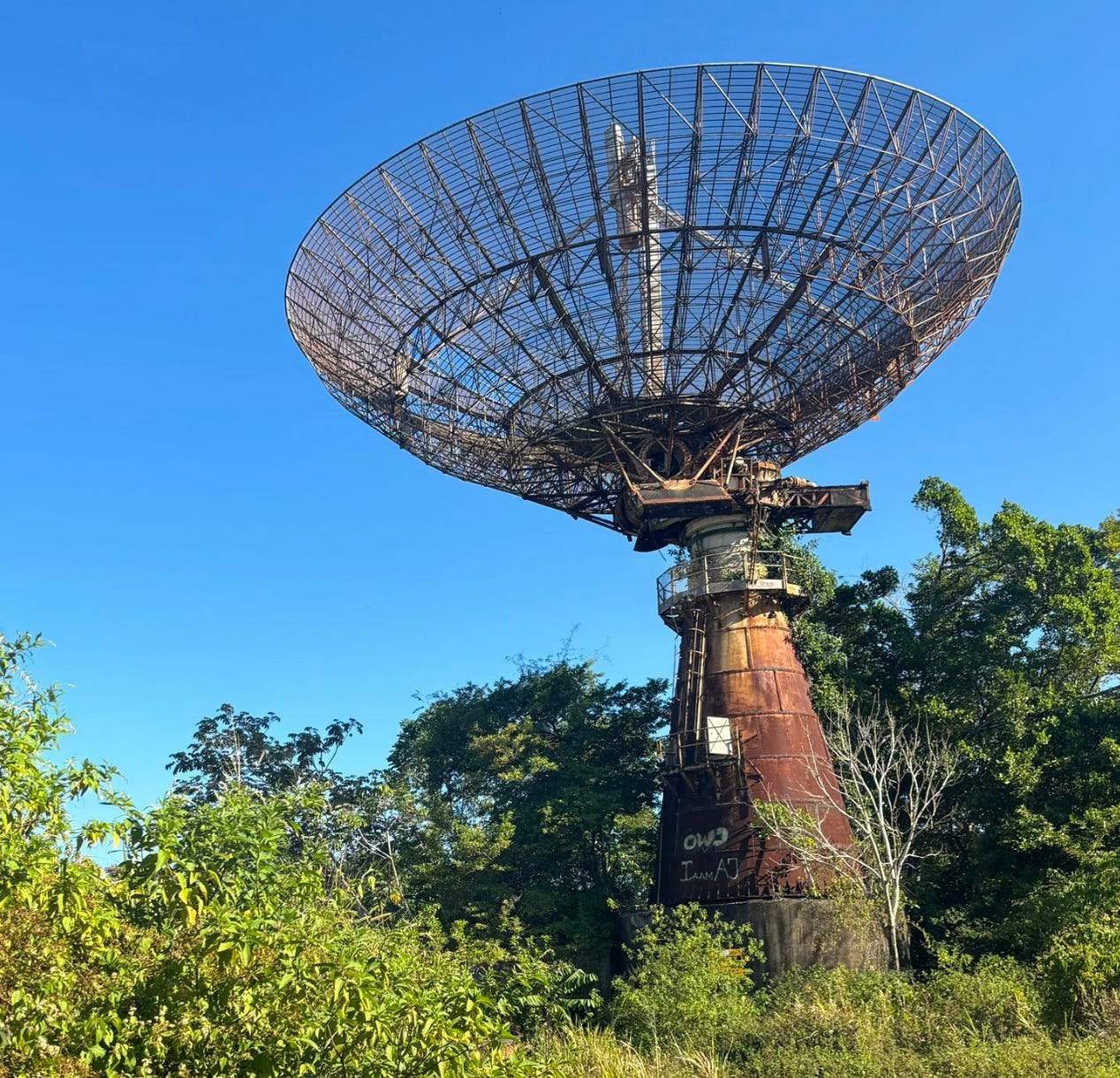