This week’s roundup is about a topic that gets a lot of people excited: AI and science. We have a new ‘AI scientist’ from Japanese outfit Sakana, research arguing that contemporary AI practice is dampening our theoretical understanding of cognition, and a report from Nature saying that academic publishers are selling access to research papers for training large models. As always, email me at hp464@cam.ac.uk if you want to get in touch.
Three things
1. I’m something of an AI scientist myself
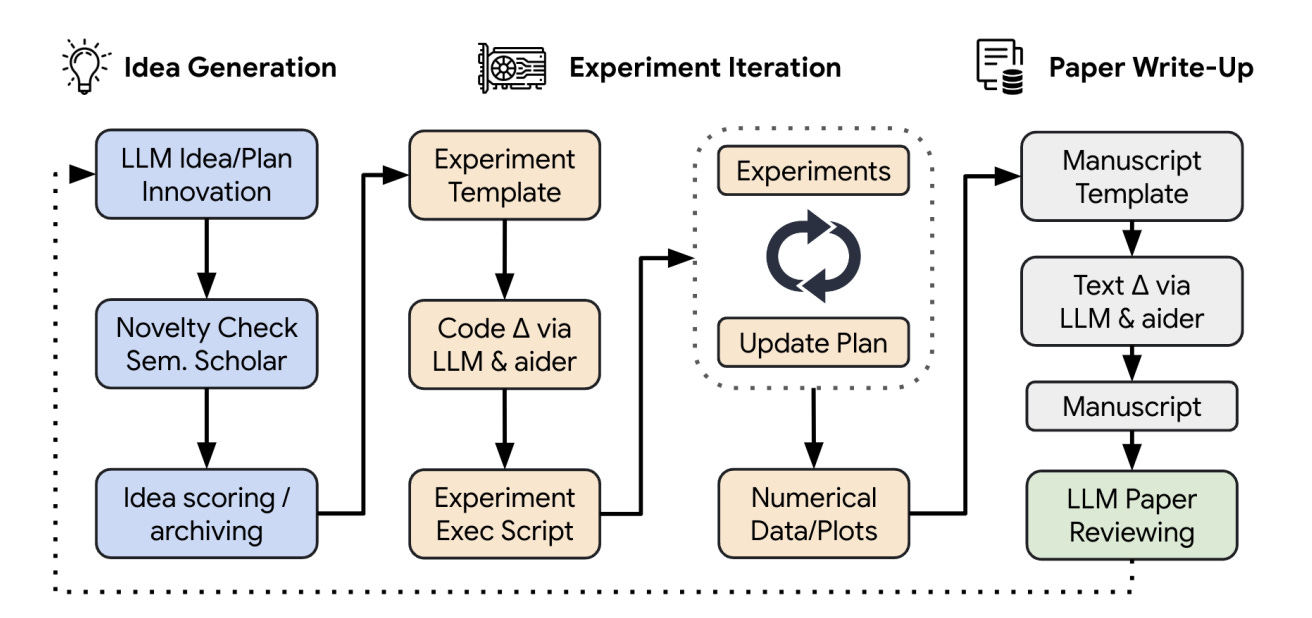
Japanese AI lab Sakana released an AI scientist, which the group described as the “world’s first AI system for automating scientific research and open-ended discovery.” The Japanese lab detailed what is essentially an ensemble of various foundation models strung together to perform different parts of the experimental process. You can probably think of it more like a group of AI scientists than a singular AI scientist.
So how does it work? First up is ‘idea generation’ in which a nominated model brainstorms research ideas and filters out duds by calling the Semantic Scholar API. Next, the ‘experimental’ phase involves implementing the proposed experiment using the Aider coding assistant, analysing the results, and refining the approach over multiple iterations. Finally, in the ‘paper writing and review’ phase, the system writes a full academic paper describing the research, which is then evaluated by a separate model acting as a peer reviewer.
The reactions have been interesting to see with this one. We begin with the people who aren’t particularly impressed by the quality of the results (though, to be fair, the authors are pretty clear on the limitations from page 17 onwards). Then there’s the folks worried about the model’s behaviour on safety grounds because, when it reached time limits imposed by the group, it tried to override them rather than shortening the experiment. And finally, what would any AI announcement be without people turning up and commenting ‘based’ or ‘cracked’.
The reception reminds me of Steven Shapin’s work on the Scientific Revolution, which argues that changes in scientific thinking in the 17th century were more gradual and less unified than we like to think. Shapin’s work was famous for stressing the cultural contexts in which scientific ideas developed, which challenged the notion that there was a sudden or radical break with earlier modes of thought.
He famously opened his book with the line: "There was no such thing as the Scientific Revolution, and this is a book about it." If the historians of the future describe our moment as a revolutionary one, then you can bet Shapin’s analysis will remain prescient. AI already is—and will continue to be—used in uneven, disparate, and unrelated ways within the scientific process. Just like there was no single Scientific Revolution in early modernity, there won’t be a single AI scientific revolution either.
2. Bring back cognitive simulation
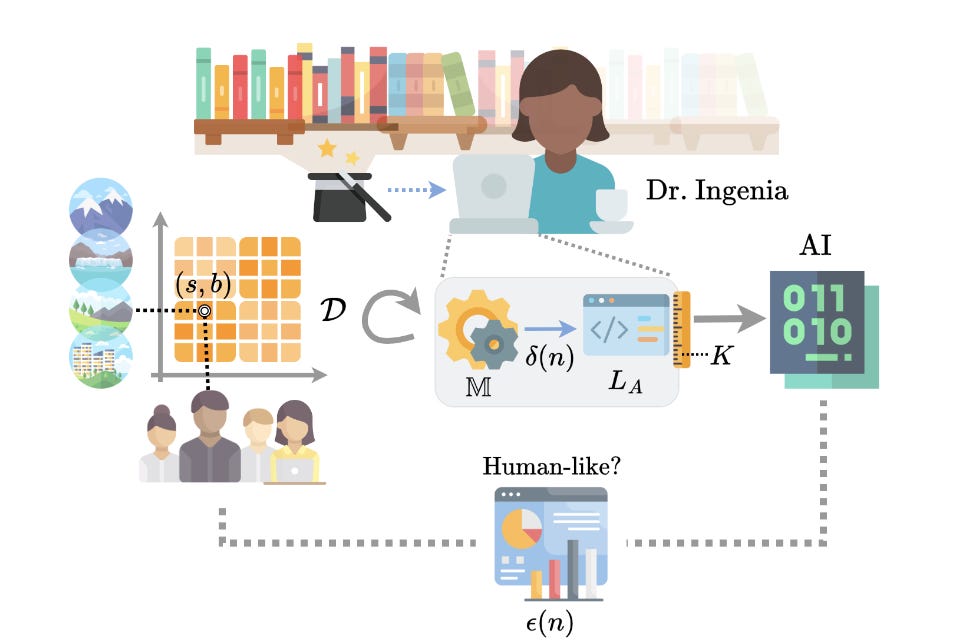
A group of researchers wrote a thought-provoking piece about the need to ‘reclaim’ the use of AI in cognitive science. Of the many (many) ways we think about AI, they make the case that one has been largely forgotten: ‘AI’ as cognitive simulation to imitate human beings.
The writers remind us there were close links between AI and cognitive psychology until around the turn of the millennium, at which point AI research stopped trying to model human cognition directly. They blame this divorce on efforts to view the mind as a computer, which, they say, have “taken the theoretical possibility of explaining human cognition as a form of computation to imply the practical feasibility of realising human(-like or -level) cognition in factual computational systems.”
Using a formal proof, they suggest (with some force!) that the development of ‘human-like’ systems is computationally intractable. Instead, they say that any AI systems created in the near future are best viewed as "decoys" that fail to capture the true complexity of human cognition.
This argument gets at a much bigger question: if systems are useful, practical, powerful, and—let’s face it—valuable, then does it really matter whether or not they are similar to human intelligence? In one sense, yes! For cognitive science, which concerns studying the nature of the mind, clearly researchers would benefit from a type of ‘AI’ that simulates human behaviour in a more internally consistent way.
But in another sense, not so much. Today, the goal of the AI project is to build systems that can do lots of different things in a reliable way. They don’t need to accurately reflect the human mind to write, converse, or code. That is to say: if it simulates general intelligence, then it is general intelligence.
3. Reading into publisher deals
Academic publishing is notoriously bad. It’s deeply bureaucratic, eye-wateringly expensive, and (speaking for myself) guaranteed to result in a string of bizarre interactions about funding and fees. Now, Nature reports that major labs are inking deals with scientific publishing houses like Taylor & Francis, which recently agreed a $10M deal with Microsoft. At the same time, the article says, Wiley earned $23 million from allowing firms to train generative-AI models on its content.
Most open access research sources like arXiv or biorXiv are already used by firms for training large models. These repositories are useful because they are generally high quality, contain information useful for helping models to answer technical questions, and (most importantly) are easy to scrape.
But they are not infinite. The addition of proprietary archives like those owned by Taylor & Francis or Wiley represents another front in the campaign to expand the total number of data sources available to developers. In many ways it is the latest chapter of a story that started 40 years ago when Bell Labs used newswire text to train a language model (yes really).
Today, firms agree deals with news outlets, social media firms, and publishers to secure access to high quality training data. But where you or I can opt out of xAI training Grok on our posts with a button, academic researchers can’t do much to prevent journals from selling on their work. It’s all in the terms of service, I guess!
Best of the rest
Friday 16 August
AgentCourt: Simulating Court with Adversarial Evolvable Lawyer Agents (arXiv)
The Red Queen problem (Substack)
Russia’s AI tactics for US election interference are failing, Meta says (The Guardian)
Google’s upgraded AI image generator is now available (The Verge)
Elon Musk's Grok xAI chatbot has few limits (Axios)
The doctor will polygraph you now: ethical concerns with AI for fact-checking patients (arXiv)
Thursday 15 August
Open-endedness is all we’ll need (Substack)
English ChatGPT users flummoxed by language glitch as bot turns Welsh (FT)
LiveFC: A System for Live Fact-Checking of Audio Streams (arXiv)
Do GPT Language Models Suffer From Split Personality Disorder? The Advent Of Substrate-Free Psychometrics (arXiv)
China's Huawei is reportedly set to release new AI chip to challenge Nvidia amid U.S. sanctions (CNBC)
Wednesday 14 August
Has your paper been used to train an AI model? Almost certainly (Nature)
Unreasonably effective AI with Demis Hassabis (Podcast > X)
Problem Solving Through Human-AI Preference-Based Cooperation (arXiv)
What are the risks from Artificial Intelligence? (AI Risk Repository)
A Study on Bias Detection and Classification in Natural Language Processing (arXiv)
Tuesday 13 August
AI and the Evolution of Biological National Security Risks (CNAS)
GPT is an effective tool for multilingual psychological text analysis (PNAS)
Grounding AI Policy: Towards Researcher Access to AI Usage Data (CDT)
Introducing SWE-bench Verified (OpenAI)
Grok-2 beta release (xAI)
On the Antitrust Implications of Embedding Generative AI in Core Platform Services (SSRN)
AlphaFold two years on: Validation and impact (SSRN)
Monday 12 August (and things I missed)
Future of Life Institute Announces 16 Grants for Problem-Solving AI (FLI)
Quest for AI literacy (Nature)
Machine Psychology (arXiv)
AI assistants (Ada Lovelace Institute)
Silicon Valley is cheerleading the prospect of human–AI hybrids — we should be worried (Nature)
Ensemble everything everywhere: Multi-scale aggregation for adversarial robustness (arXiv)
Can You Trust An AI Press Release? (Asterisk)
A New Type of Neural Network Is More Interpretable (IEEE Spectrum)
Job picks
Some of the interesting (mostly) AI governance roles that I’ve seen advertised in the last week. As usual, it only includes new positions that have been posted since the last TWIE (but lots of the jobs from the previous edition are still open).
Various open roles, CFI (UK)
Teaching Fellow, AI Alignment, BlueDot Impact (Global)
Survey Researcher, Georgetown University, Center for Security and Emerging Technology (US)
Research Associate, AI Governance, Standards and Regulation, Alan Turing Institute (UK)
Fellowship, Non-Trivial (Global)