It’s a milestone edition of The Week In Examples that marks a full year (give or take some holidays) since I started writing a weekly note about AI and society. Thank you to everyone who has read these posts, sent me ideas for things to include, given me feedback or just said hello during the last year. Please keep doing those things as I continue to try to make sense of how AI is, for better or worse, making things progressively weirder changing the world.
Three things
1. AI factories
The European Commission announced a call for proposals for its AI factory initiative, which will allow AI developers to build on the EuroHPC network of supercomputers by providing access to data, computing, and storage services. There are currently nine EuroHPC supercomputers across Europe, with the best equipped for AI Germany’s Jupiter computer that houses 24,000 of NVIDIA’s new GH200 chips.
According to the proposal, the EU is looking at three options for groups interested in receiving support: those seeking to build new AI-optimised supercomputers, those running existing EuroHPC supercomputers interested in upgrading them to make them better suited to AI, and those seeking to create ‘AI factories’ around either new or upgraded systems. In essence, the AI factory process has three stages:
Existing or new supercomputer hosts apply to become AI factories (I suspect most of these will be existing bodies like the CSC – IT Center for Science in Finland)
Recipients get support to bundle together compute, large-scale data storage and access capabilities, AI development software and tools, and expertise and talent
In turn, AI factories provide startups, SMEs, and researchers with these resources to train large AI models
In some ways it’s a very European proposal: the goal is to harness comparative advantages in science and research to plug the gap left by mature AI firms (by way of mountains of paperwork). Whether it closes the gap with the US, though, will depend on how successful the initiative is in securing enough specialised AI hardware – a tall order without creating powerful capital incentives.
2. LLMs can generate novel research ideas
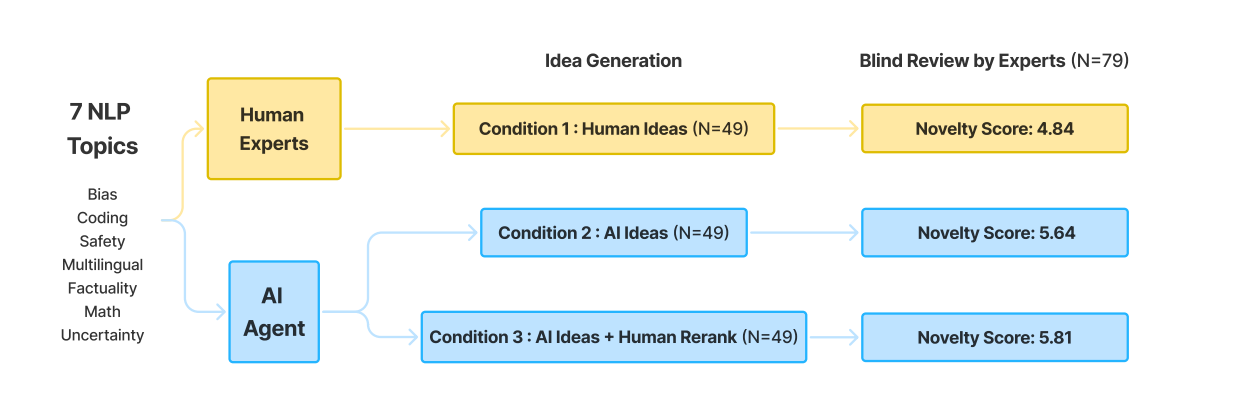
Betteridge's law of headlines famously says “any headline that ends in a question mark can be answered by the word no”. I am thinking about coining a similar term (Law’s law, if you will) for arXiv papers: “any paper title that ends in a question mark can be answered by the word yes”.
A case in point is new work from researchers at Stanford University, which asked “Can LLMs Generate Novel Research Ideas?” To give us the ‘yes’ we were all waiting for, the authors set up an experiment looking at prompting-based NLP research across topics like bias, coding, safety, multilingualism, factuality, maths, and uncertainty. The researchers pitted human NLP researchers against an AI system to create fresh research ideas organised around these topics (e.g. prompting methods to reduce social biases), which were in turn reviewed by third party experts – without knowing which was which.
AI-generated ideas were consistently rated as more novel than the human-generated ideas across multiple rounds of analysis, though it is worth saying that they actually asked the AI (in this case, Anthropic’s Claude) to rewrite the prompts to prevent judges from spotting a rogue ‘delve’ and guessing which ideas belonged to which group.
3. Superhuman scientific synthesis
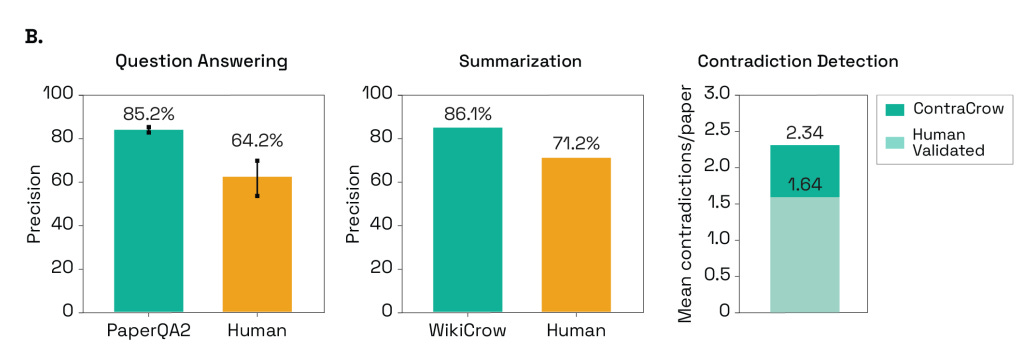
Everyone knows that language models have an unfortunate tendency to hallucinate. Even though we are rightly and repeatedly told to verify outputs, it is a habit that prevents them from being widely used for tasks where they show a lot of promise. One such area is the humble scientific literature review, which essentially boils down to reading, summarising, and drawing comparisons between lots of papers.
Now, researchers from FutureHouse, University of Rochester, and the Francis Crick Institute think they have something much more reliable for scientists to use than popular models like Claude, ChatGPT, or Gemini. They introduced PaperQA2, a language model optimised for “improved factuality” capable of exceeding subject matter experts on literature research tasks.
PaperQA2 was created using LitQA2, a set of 248 multiple choice questions with answers that could only be successfully completed by retrieving pieces of information from the scientific literature. Using LitQA2 as an input for the system, the group built a new form of retrieval augmented generation (RAG) in which the system essentially looks up information from papers to inform its answers.
But where existing versions of RAG (which, as an aside, are already being widely used to curtail hallucinations) tend to work on a one-shot basis, the group’s approach searches for relevant papers, summarises the most relevant parts, searches for more papers by following citation links, and uses the gathered information to generate an answer.
Crucially, though, is that each of these steps can be repeated, which means the model will search again or look deeper into citations if it doesn't find enough information on the first pass. The upshot is that, compared to human experts, PaperQA2 handily beat expert performance on questions like "What effect does bone marrow stromal cell-conditioned media have on the expression of the CD8a receptor in cultured OT-1 T cells?" with scores of 85% for the model and 64% for humans.
Best of the rest
Friday 13 September
Modernizing the WARN Act to Protect US Workers from AI Displacement (Tech Policy Press)
The case for promoting the geographic and social diffusion of AI development (Brookings)
OpenAI o1 System Card (OpenAI)
A.I. Is Changing War. We Are Not Ready. (NYT)
OpenAI, Nvidia Executives Discuss AI Infrastructure Needs With Biden Officials (Bloomberg)
Thursday 12 September
Learning to Reason with LLMs (OpenAI)
Getting the UK’s Legislative Strategy for AI Right (TBI)
Same but more-ism (Substack)
Top tech, U.S. officials to discuss powering AI (Reuters)
Implementation of ISO/IEC 42001:2023 standard as AI Act compliance facilitator (Taylor Wessing)
OpenAI Nears Release of ‘Strawberry’ Model, With Reasoning Capabilities (Bloomberg)
Wednesday 11 September
NotebookLM now lets you listen to a conversation about your sources (Google)
This New Tech Puts AI In Touch With Its Emotions—and Yours (WIRED)
Decoding Gemini with Jeff Dean (X)
Chapter one of AI Snake Oil (Princeton University Press)
Predicting: The future of health? (Ada Lovelace Institute)
Tuesday 10 September
Three Big Ideas #1 (Substack)
Warren McCulloch in 1962 on whether machines that outlived humanity would have purpose (X)
Reflections on Reflection (Substack)
The Race to Reproduce AlphaFold 3 (Substack)
EU Competitiveness: Looking ahead (EU)
Monday 9 September (and things I missed)
Understanding Microsoft's “Aurora: A Foundation Model of the Atmosphere” (Substack)
A post-training approach to AI regulation with Model Specs (Substack)
Verification methods for international AI agreements (arXiv)
Superhuman Automated Forecasting (CAIS)
Conversational Complexity for Assessing Risk in Large Language Models (arXiv)
Job picks
Some of the interesting (mostly) AI governance roles that I’ve seen advertised in the last week. As usual, it only includes new positions that have been posted since the last TWIE (but lots of the jobs from the previous edition are still open).
Content Writer, Freelance, CivAI (Remote, global)
Research Intern, Conditional AI Safety Treaty, Existential Risk Observatory (Netherlands)
PhD Fellowship, US-China AI Governance (2025), Future of Life Institute (Remote, global)
Innovation Fellowships, Policy-Led, Digital Society (2024-2025), The British Academy (UK)
Reporter MIT Technology Review (US)